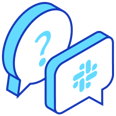
Join StarRocks Community on Slack
Connect on Slack“Time is money”
– Benjamin Franklin, Founding Father of the United States
Which analytics report is more important – understanding what happened to your business last year or understanding what is happening today? Well, both reports provide useful insights, but in today’s VUCA (volatility, uncertainty, complexity, and ambiguity) world understanding what’s going on here-and-now holds the key for improved business performance. Given that, businesses are increasingly assessing the opportunity cost these days, this means, each choice enterprises make could be delayed if timely insights are not available and this usually leads to decreased performance. In other words, time is money in business.
Against this backdrop, companies today across different industry sectors need real-time analytics. Netflix uses data to make on-the-fly decisions on what you want to watch based on what is currently trending. Facebook uses real-time analytics to identify and purge posts related to fake news and abusive posting. Amazon changes product prices 2.5 million times a day, meaning that an average product listed on Amazon changes prices every 10 minutes. That is fifty times more often than Walmart and Best Buy. The constant price changes based on real-time analytics has helped Amazon boost profits by 25 percent [1]. Basically, the list of real-time analytics use cases is endless.
This blog, the second one as part of my 3-series blog is to help the data and analytics community understand and guide the implementation of real-time analytics solutions in business enterprises. The first blog discussed the fundamentals of real-time analytics [2]. Let me quickly recap key concepts discussed in that first blog. According to Gartner, real time simply means the analytics is completed within a few seconds or minutes after the arrival of new data. For some use cases, real time simply means the analytics is completed within a few seconds or minutes after the arrival of new data.
Overall, real-time analytics enables businesses to react to the available data with minimal delay. It is about minimizing the latency associated with data ingestion into the data warehouse and querying of data within the data warehouse. In other words, real-time analytics addresses data latency and query latency. Data latency is a measure of the time from when data is generated to when it is queryable. As there is usually a time lag between when the data is collected and when it is available to query, real-time analytics systems minimize the lag associated in getting the data to the data warehouse. Query latency is the amount of time it takes to execute a query on the data in the data warehouse and receive the output.
In this blog, I will discuss some practical use-cases of real-time analytics. Before we look at the use-cases of real-time analytics, let us first look at the main driver for real-time analytics. Basically, businesses want more granular data, derive insights quickly, and take timely decisions. The process of generating timely insights from data which includes noticing the change, formulating questions, deriving insights, and taking action can be days, and in some cases weeks or months. The key to shrink the decision cycle time is to reduce the time lag between data availability and insights derivation. This can lead to quicker decisions and greater business productivity. Real-time analytics is about capturing and deriving insights as it happens – or as close as it is possible to get. With real-time analytics businesses can take advantage of opportunities that could otherwise be missed and prevent problems before they arise. Now, let use look at the four popular use-cases on real-time analytics that have a significant business impact.
- Targeted promotions in Retail.Retail businesses can use real-time analytics to deliver promotions and incentives to customers to increase the chances of a sale. This can happen when the customers are inside the store or even when they are near the store using the geolocation feature in their mobile phones by creating relevant messages based on current events, trends, or feedback. For instance, Sephora, a multi-national retailer of personal care and beauty products, keeps a constant pulse on in-store performance and make necessary adjustments on a real-time basis. This real-time analytics is enabled as each store tracks and analyzes up to 40 different metrics each day, gauging the store and customer performance and patterns, and turning data into actionable insights. [3].
- Fraud detection in Financial Services. Financial Services organizations especially Banks and Insurance firms apply real time analytics to help detect and prevent fraud. This includes using data for risk scoring to group consumers into identifiable clusters based on typical customer behavior. By applying real time analytics on the vast amounts of historical data, Financial Services organizations are able to categorize customers into a number of different risk profiles that can be used to make predictions on future financial transactions. For example, an account could be profiled as "eats at restaurants on weekends" or "fills car with gasoline on Tuesdays”, and so on. When these transactions are made, real time analytics can be used to determine whether or not the customer fits a particular pattern or departs enough from the norm to warrant being flagged thereby offering a secure solution to fraud prevention while maintaining a high-quality customer experience.
- Asset reliability in Oil and Gas. With real time analytics, oil and gas companies can ensure their employees are safe, their fields are more productive, and their capital assets are operating at peak efficiency. The oil and gas industry has historically generated vast quantities of real-time data through sensors and SCADA (Supervisory Control and Data Acquisition) systems. Real-time analytics can help oil and gas companies to identify and prevent safety or production issues, schedule maintenance including turnarounds and repairs more efficiently and optimise the reliability of their production assets. In addition, with real-time analytics personnel can be deployed more effectively and safely, plant downtime reduced, production maximised, and incident response times improved.
- Optimization of Electricity Distribution. In the utilities sector, real-time analytics can help achieve improved operational efficiencies in electricity grids. Computer load flow analysis has been used in electricity transmission and distribution utilities to simulate and analyze voltage, current, and other variables for system planning and operations. SCADA systems capture data on generators, control systems, transmission lines, substations, distribution lines, and in-line equipment and devices. Smart meters collect data on how much the electricity is used and when it is used. By dealing with huge amount of data from all these systems on a real-time basis, many benefits can be brought to the existing power system, including improved the customer service and optimized distribution of electricity.
Organizations today are collecting and processing more data, faster than ever before. But many companies are struggling to turn these enormous amounts of data into useful insights and decisions in a timely fashion. This is hampering their ability to grow the revenue, reduce expenses, and mitigate risks. In today’s competitive business environment, delayed decision is practically a bad decision. Real-time analytics turns data into insights quickly after it is collected and these kinds of insights are very useful in situations when time is of the essence. In other words, real-time analytics can help organizations in every industry turn data into insights at speed and scale.
References
- https://www.businessinsider.com/amazon-price-changes-2018-8
- https://starrocks.com/blog/real-time-analytics-what-so-what-and-now-what-starrocks
- https://www.retailtouchpoints.com/features/retail-success-stories/sephora-empowers-store-leaders-with-real-time-data
- https://www.datacenterdynamics.com/en/opinions/cost-decision-latency/
About the Author
Dr. Prashanth Southekal is the Managing Principal of DBP Institute (www.dbp-institute.com), data and analytics consulting, research and education firm. He is a Consultant, Author, and Professor. He has consulted for over 75 organizations including P&G, GE, Shell, Apple, and SAP. Dr. Southekal is the author of two books — “Data for Business Performance” and "Analytics Best Practices” — and writes regularly on data, analytics, and machine learning in Forbes.com, FP&A Trends, and CFO.University.
ANALYTICS BEST PRACTICES is in the top 100 analytics books of all time and in May 2022 was ranked #1 by BookAuthority. Apart from his consulting pursuits, he has trained over 3,000 professionals worldwide in Data and Analytics. Dr. Southekal is also an Adjunct Professor of Data and Analytics at IE Business School (Madrid, Spain). CDO Magazine included him in the top 75 global academic data leaders of 2022. He holds a Ph.D. from ESC Lille (FR) and an MBA from Kellogg School of Management (U.S.). He lives in Calgary, Canada with his wife, two children, and a high-energy Goldendoodle dog. Outside work, he loves juggling and cricket.