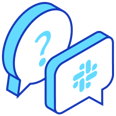
Join StarRocks Community on Slack
Connect on SlackTABLE OF CONTENTS
In the world of data analytics, new technologies and solutions are emerging nearly every day. Businesses need to stay current on these developments so they can spot opportunities and adopt solutions that will give them an edge in the market. It can be daunting to keep up with all of these changes, so this article will bring you up to date on the most recent, and important, innovations in data analytics.
Data analytics is the practice of extracting insights from data sets to support business decisions.
Introduction to Data Analytics
Since data analytics can mean different things depending where you sit in an organization, it's important we define what data analytics means when we talk about it here.
Data analytics is the practice of extracting insights from data sets to support business decisions. Data analytics spans multiple industries and uses a variety of technologies, including data integration, data warehousing, machine learning, and predictive modeling.
There can be no doubt that the biggest developments in data analytics in the last several years has been in the collection and storage of unprecedented amounts of data. Originally, we called this Big Data, and only some of the largest enterprises had the resources to utilize it. This is no longer the case. The emergence of cloud platforms such as AWS has given businesses of all sizes, in theory, unlimited storage capacity.
Businesses are still on the hook, however, to figure out how to make sense of this data. In this article we will take a look at 5 of the latest trends in the Data Analytics world and how they can help you.
1. Machine Learning and AI Are Enhancing Data Analytics
A fairly recent development in data analytics has been the integration of artificial intelligence (AI) and machine learning (ML). Machine learning, as the name indicates, 'learns' from large volumes of data to identify patterns and make predictions about the future. Predictive modeling, for example, is a method of machine learning that models future results by analyzing patterns in past data. Machine learning can be used for all sorts of applications, but it’s especially relevant to data analytics since it gets 'smarter' as it acquires more data.
In the past few years, a few new developments in machine learning and AI have paved the way for more real-time data analytics. New hardware architectures like GPUs are showing up in mainstream applications, and new programming frameworks like TensorFlow and PyTorch have made it easier for data scientists to create machine learning applications.
Finally, businesses have developed new systems for storing data, including cloud data lakes, data hubs, and real-time data warehouses. With more data and more powerful computing resources, AL/ML algorithms can automate many analytics tasks, identify patterns, and draw insights from data assets without human instructions.
2. Real-Time Analytics Is Gaining Momentum
Previously, businesses could only analyze data that they had collected in the past — maybe in the previous day or week. The only real-time data analytics were occurring in places like stock exchanges or weather reporting where you'd deal with a limited window of real-time data. Big Data, which was used to store this data, wasn’t always available for real-time analytics.
Now, though, businesses can use the cloud to store large amounts of data and make it easily accessible for real-time analytics. Moreover, new developments in query engine technologies are fundamentally changing how real-time analytics are conducted, enabling more use cases for businesses. The wider adoption of real-time technologies will allow businesses to make better decisions faster, which is especially helpful in sectors like healthcare, transportation, and supply chain.
3. Metadata Makes Data More Accessible to Business Users
Because the sources of data can vary, it’s important to be mindful of the metadata associated with it. Metadata can include critical information such as who collected the data, when they collected it, and what steps they took to preserve accuracy.
New technologies that provide advanced metadata management capabilities, such as data catalogs, semantic layers, metrics stores, and feature stores, have gained traction in recent years. They are also quite different from the last generation of metadata management tools built for data warehouses. Designed to support today's data volumes, complexity, and timeliness, these new solutions are becoming essential for data analytics.
4. Enhanced Data Quality for Greater Confidence
Building end to end observability for the analytics pipeline will be on more than one data team's project list in the coming year. Observability gives businesses confidence about the insights gained through their data analytics and the decisions made based on those insights.
From data to insight to decision, observability applies software engineering and devops principles to ensure the end to end quality of your analytics platform.
According to Gartner, data observability has now become essential for supporting and augmenting existing, and future, data management architectures. Observability is becoming an integrated part of data analytics processes. If it isn't a priority today, it will be tomorrow.
5. Blockchain Is the New Friend of Data Analytics
Blockchain is an innovative technology that you've likely seen in the headlines thanks to its use in the cryptocurrency space. As a decentralized digital ledger, Blockchain records transactions across a network of computers. These computers, called nodes, are connected to each other via a peer-to-peer network.
But, blockchain isn’t just for cryptocurrency. Businesses are now starting to use it for other applications since blockchain creates a tamper-proof digital record of transactions. This enables businesses to store sensitive data like personal information, trade secrets, and financial records without worrying about security. This makes blockchain especially useful for industries like healthcare and supply chain management, where data needs to be secured. Analytics and blockchain are quickly becoming mutually beneficial. Data analytics helps blockchain companies better understand the transactions happening on the chain, while blockchain can improve the security and authenticity of data analytics.
What's Ahead for Data Analytics in 2023
Data analytics is not just a one-time activity — it’s a continuous process of looking at your data and figuring out what it means. Data analytics started out as a way of analyzing large amounts of data stored in databases. When these databases became too large and complicated for traditional computers, engineers developed new technologies like Hadoop and Apache Spark that allowed them to keep up. Cloud technology has further enabled businesses to store and process even more data. Innovation in the data analytics space is always taking place, and new technologies such as AI/ML, real-time data streams, and blockchain are pushing the boundaries of analytics to enable more accurate and timely decision making.
copy success