Understanding how users interact with your platforms and products is paramount to success. It's no surprise then that user behavior analytics, a discipline that deciphers the choices, actions, and patterns of users, continues to grow in popularity. In this article, we'll dive deep into the intricacies of user behavior analytics, its significance, and how it is shaping the future of digital experiences.
Learn more about user behavior analytics in this webinar
Defining User Behavior Analytics
User behavior analytics is a type of analytics that derives insights from user actions and enables analysts to understand behavior patterns in greater detail and clarity. This makes it ideal for identifying product issues, spotting new business opportunities, and refining operations to be more precise and effective.
Figure 1: Key components of user behavior analytics
The core of user behavior analytics focuses on the user (who) and the event or action (what). This often includes:
-
Observing specific user metrics based on one or a series of defined actions like event tracking and funnel and retention analyses.
-
Identifying a particular user or group of users to monitor their actions, such as audience segmentation or path analytics of specific groups.
Types of User Behavior Analytics
Below are a few of the most common scenarios you'll find when it comes to user behavior analytics.
Event analysis
Event analysis is a foundational element of all behavior analytics scenarios. Every action by a user can be defined as an event and assigned business relevance. The most common manifestation of event analytics is in the form of a metrics dashboard. Dashboard operators frequently monitor key product metrics to understand business changes and adjust their strategies accordingly.
Retention analysis
Retention analytics is typically used to gauge user stickiness. For example, examining the retention rate of new users on the 2nd, 3rd, or 7th day post-registration provides insights into user loyalty. Monitoring changes in the spending of retained new users can also be insightful; for example, if the average expenditure of newly retained users over the past week has decreased, there might be issues with user acquisition.
Funnel analysis
Funnel analytics refers to the conversion analytics within a specified time frame and on a designated path. For instance, tracking the conversion rate from "Page View -> Add To Cart -> Purchasing -> Successful Payment". If the overall conversion rate is low, individual step conversion rates can be analyzed to pinpoint problems. Specific optimizations and A/B tests can then be applied. For example, if there's a notable drop in conversions from "Page View" to "Add To Cart", the order process might need fine-tuning.
Figure 2: An example of funnel analysis
User path analysis
In funnel analytics, we specify a desired user journey to monitor conversions. However, user actions are often unpredictable. Thus, studying what diverted users did is crucial. For instance, if a significant percentage of users drop off between "browsing an event page" and "adding to cart", we can track these users to see what they did post-event browsing. If over half engaged in a "search" action, it could imply the event page lacked items of interest, suggesting a potential area for algorithm improvement.
Session analytics
A session, in this context, refers to a series of user actions on a website or app within a certain timeframe. For instance, a session could include multiple page views and interaction events. A prerequisite for session analytics is defining how sessions are segmented, including their duration and the events they encompass.
User-behavior Analytics Use Cases
User behavior analytics plays a critical role in businesses across nearly every industry. Here are a few real-life examples:
Gaming: A leading gaming company is leveraging user-behavior analytics to gauge the performance of its vast array of games. With each game crafted by different studios and teams, the unifying method to assess their performance company-wide is through user-behavior analytics. This comprehensive approach enables the company to execute insightful A/B testing, subsequently evaluating the outcomes based on users' behaviors and preferences.
Ride-sharing: One of the globe's leading ride-sharing platforms is capitalizing on user-behavior analytics to ensure seamless service delivery and an optimized user experience. By obtaining real-time aggregated data, such as the count of riders inspecting the app within distinct areas, they can adeptly anticipate user demand. This proactive approach extends to monitoring real-time order placements, thus predicting demand spikes and refining wait times for passengers and route strategies for drivers. Moreover, by scrutinizing real-time user behavior data on varying dimensions—from an overarching company perspective down to specific user groups in confined regions—this company can make informed strategic decisions regarding promotions and pricing adjustments.
Entertainment services: A major entertainment service company, boasting an impressive on-demand video streaming platform with over 200 million monthly active users, utilizes user-behavior analytics to drive its strategies. By engaging in real-time monitoring of user behavior metrics, the company is able to run targeted promotions and maintain an updated trending board that resonates with its vast audience.
Challenges in User Behavior Analytics
As popular as user behavior analytics is, it is not without its share of challenges. User behavior analytics typically relies on embedded logs, which are often semi-structured or unstructured data. This brings with it several issues that need to be considered:
Huge data volumes:
-
Scale of data collection: As users interact with platforms, every touchpoint, from clicks to page views, accumulates data. When scaled to thousands or millions of users, the volume becomes substantial.
-
Performance overhead: Such voluminous data can introduce performance issues, slowing down retrieval times and complicating real-time analytics.
-
Data management: Storage, backup, and retrieval become increasingly intricate tasks, demanding more advanced infrastructure and heightened maintenance efforts.
Integration challenges:
-
Inconsistent formats: User behavior analytics often involves mixed data types including semi-structured data, making it harder to process and standardize.
-
Data transformation: Before analytics, user behavior data often requires transformation into a more usable format, adding an additional layer of processing.
-
Integration hurdles: Merging semi-structured data with structured datasets (like databases) can be complex, posing challenges in creating a unified data view.
Highly flexible queries, tightly coupled with business logic:
-
Diverse analytics needs: Tailored to specific business goals, queries need to be crafted with precision, making generic approaches less effective.
-
Evolving requirements: As business strategies and goals shift, so do the queries, necessitating constant revisions and adjustments.
-
Execution complexity: Custom, intricate queries can be resource-intensive, leading to potential performance bottlenecks and increased processing times.
Get Started with User Behavior Analytics Today
From gaming and ride-sharing to entertainment services, the far-reaching implications of user-behavior analytics underscore its significance in sculpting enhanced user experiences.
It's also now easier than ever to adopt user behavior analytics for your business or next project. New solutions like CelerData Cloud, offer an analytics platform designed to effortlessly tackle the challenges of user-behavior analytics. Quickly get a feel for how user-behavior analytics can benefit your business with a 30-day free trial. Sign up now.
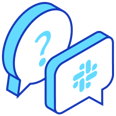
Join StarRocks Community on Slack
Connect on Slack