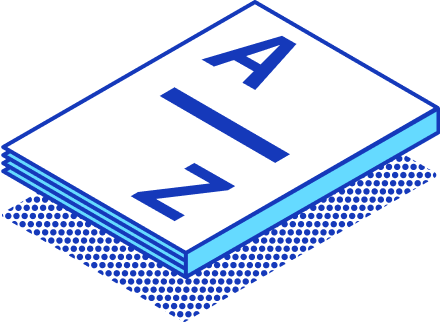
A/B Testing
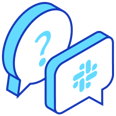
Join StarRocks Community on Slack
Connect on SlackWhat is A/B Testing?
A/B Testing, also known as split testing, involves comparing two versions of a webpage or app to determine which one performs better. Marketers and product managers use this method to test different elements, such as headlines, images, or call-to-action buttons. The goal is to identify which version yields higher engagement or conversion rates. By systematically changing one variable at a time, businesses can make data-driven decisions that enhance user experience and optimize performance.
Key components (Control and Variation)
A/B Testing consists of two key components: the control and the variation. The control represents the original version of the content, while the variation introduces a change to one specific element. For example, a company might test a new headline on a landing page. The control would display the original headline, and the variation would show the new headline. By comparing the performance of both versions, businesses can determine which one achieves better results based on predefined metrics.
How A/B Testing Works
Hypothesis creation
The first step in A/B Testing involves creating a hypothesis. This hypothesis should be a clear and testable statement predicting how a change will impact a specific metric. For example, a marketer might hypothesize that changing the color of a call-to-action button from blue to red will increase click-through rates. A well-defined hypothesis provides a foundation for designing the test and measuring its success.
Test execution
Once the hypothesis is established, the next step is to execute the test. This involves setting up the control and variation, ensuring that both versions are identical except for the one element being tested. Tools like Google Optimize, Optimizely, and VWO can facilitate this process by randomly assigning users to either the control or variation group. Proper test execution ensures that the results are reliable and free from bias.
Data collection and analysis
After running the test, data collection and analysis become crucial. Metrics such as click-through rates, conversion rates, and user engagement levels are gathered for both the control and variation. Statistical analysis helps determine whether the observed differences are significant or due to random chance. Businesses can then use these insights to make informed decisions, optimizing their digital strategies and improving overall performance.
Benefits of A/B Testing
Improved User Experience
Personalization
A/B Testing allows businesses to tailor their content to individual user preferences. By testing different versions of a webpage or app, companies can identify which elements resonate most with users. This approach leads to a more personalized experience, enhancing user satisfaction and loyalty. For example, changing the layout or color scheme based on user behavior can significantly improve engagement.
User Engagement
Increased user engagement often results from effective A/B Testing. By experimenting with various design elements and functionalities, businesses can determine what keeps users on their site longer. Higher engagement levels often translate to better user retention and higher conversion rates. A/B Testing helps pinpoint the most effective strategies for capturing and maintaining user interest.
Increased Conversion Rates
Sales and Revenue Impact
A/B Testing has a proven track record of boosting sales and revenue. Companies that regularly conduct A/B Tests see significant improvements in their conversion rates. For instance, some firms have reported up to a 300% increase in conversions through systematic testing. These improvements directly impact the bottom line, making A/B Testing an invaluable tool for revenue growth.
Lead Generation
Generating leads becomes more efficient with A/B Testing. By optimizing landing pages and call-to-action buttons, businesses can attract more potential customers. A/B Testing helps identify the most compelling elements that drive users to take action. This leads to higher lead generation rates, contributing to overall business growth.
Data-Driven Decision Making
Reducing Guesswork
A/B Testing eliminates the need for guesswork in decision-making. Businesses can rely on concrete data to determine the effectiveness of different strategies. This data-driven approach reduces the risk of implementing changes that may not yield positive results. Instead, companies can make informed decisions that are backed by empirical evidence.
Enhancing Strategy
Strategic planning benefits immensely from A/B Testing. By continuously testing and iterating, businesses can refine their strategies over time. This iterative process ensures that companies stay ahead of market trends and user preferences. A/B Testing provides valuable insights that help enhance overall business strategy, leading to sustained success.
Implementing A/B Testing
Setting Up Your Test
Identifying Goals
Identifying goals forms the foundation of effective A/B Testing. Goals should align with business objectives and focus on measurable outcomes. For example, a company might aim to increase click-through rates or boost sales conversions. Clear goals guide the entire testing process and ensure that efforts remain focused on achieving desired results.
Choosing Metrics
Choosing appropriate metrics is crucial for evaluating the success of A/B Testing. Metrics should directly relate to the identified goals. Common metrics include conversion rates, bounce rates, and user engagement levels. Selecting the right metrics allows businesses to accurately measure the impact of changes and make data-driven decisions.
Designing Your Test
Creating Variations
Creating variations involves developing different versions of the content to test. Each variation should introduce a single change to isolate its effect. For instance, a company might test different headlines or call-to-action buttons. Tools like Google Optimize, Optimizely, and VWO facilitate this process by allowing teams to create and manage multiple variations efficiently.
Ensuring Statistical Significance
Ensuring statistical significance is vital to validate the results of A/B Testing. Statistical significance indicates that observed differences are not due to random chance. Businesses must calculate the required sample size and run tests long enough to gather sufficient data. This approach ensures that conclusions drawn from the test results are reliable and actionable.
Running Your Test
Duration and Timing
Determining the appropriate duration and timing for A/B Testing is essential. Tests should run long enough to capture meaningful data but not so long that external factors skew the results. The timing of the test can also impact outcomes. For example, running a test during a high-traffic period might yield different results compared to a low-traffic period. Proper planning ensures that the test duration and timing align with business needs.
Monitoring Performance
Monitoring performance throughout the A/B Testing process is critical. Continuous monitoring helps identify any issues or anomalies that may arise. Tools like Google Optimize, Optimizely, and VWO provide real-time data and insights. Regularly reviewing performance metrics allows businesses to make informed adjustments and optimize their strategies effectively.
Analyzing Results
Interpreting Data
Interpreting data forms a crucial step in the A/B testing process. Accurate interpretation ensures that businesses derive meaningful insights from their experiments. Tools like Google Optimize, Optimizely, and VWO provide robust analytics capabilities. These platforms help in visualizing data trends and understanding user behavior.
Google Optimize offers a user-friendly interface for analyzing test results. The platform provides detailed reports on key metrics such as conversion rates and user engagement. Businesses can easily compare the performance of control and variation groups.
Optimizely excels in providing comprehensive data analysis. The platform allows teams to segment data based on various parameters. This segmentation helps in identifying specific user behaviors and preferences. Optimizely also supports multipage testing, offering a broader perspective on user interactions.
VWO goes a step further by integrating feedback mechanisms. The platform includes tools like Survey and Reveal to gather user feedback and monitor customer behavior. This holistic approach enables businesses to understand the 'why' behind user actions.
Making Informed Decisions
Making informed decisions relies heavily on the insights gained from data analysis. Businesses must ensure that decisions are backed by empirical evidence. A well-structured A/B test provides a clear direction for optimization efforts.
Google Optimize supports decision-making by highlighting statistically significant results. The platform's intuitive dashboards make it easy to identify winning variations. Businesses can confidently implement changes that show a positive impact.
Optimizely offers advanced features for decision-making. The platform's robust analytics engine helps in identifying trends and patterns. Teams can use these insights to refine their strategies and improve overall performance.
VWO enhances decision-making with its comprehensive data collection. The platform's integration of user feedback provides a deeper understanding of user needs. Businesses can leverage these insights to create more personalized experiences.
Best Practices and Common Pitfalls
Best Practices
Clear Objectives
Establishing clear objectives forms the foundation of effective A/B testing. Objectives should align with business goals and focus on measurable outcomes. For example, a company might aim to increase click-through rates or boost sales conversions. Clear objectives guide the entire testing process and ensure that efforts remain focused on achieving desired results.
Consistent Testing
Consistent testing ensures the reliability and validity of A/B test results. Regularly conducting tests helps businesses stay updated with market trends and user preferences. For instance, companies that frequently run A/B tests can adapt more quickly to changes in user behavior. Consistency in testing also allows for continuous improvement and optimization of digital strategies.
Common Pitfalls
Sample Size Issues
Sample size issues often undermine the reliability of A/B test results. A small sample size may lead to inaccurate conclusions due to random chance. Businesses must calculate the required sample size before running tests. Larger companies are 81% more likely to run A/B tests compared to smaller ones, which often have limited resources. Ensuring an adequate sample size helps in obtaining statistically significant results.
Misinterpreting Data
Misinterpreting data can lead to incorrect decisions and strategies. Accurate data interpretation is crucial for deriving meaningful insights from A/B tests. Tools like Google Optimize, Optimizely, and VWO provide robust analytics capabilities to help businesses understand user behavior. Over 85% of tests produce better insights when combining behavioral customer data with A/B testing. Proper data analysis ensures that decisions are backed by empirical evidence, reducing the risk of implementing ineffective changes.