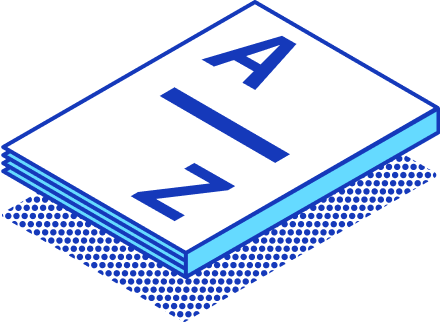
Best Practices for Building Data Lakes in 2025
Modern organizations rely on data lakes to manage the growing complexity of data. These systems provide a centralized repository for storing structured and unstructured data, enabling advanced analytics and decision-making. However, building a scalable and secure data lake requires addressing several key considerations. For example, data governance issues, security risks, and integration challenges often hinder implementation. A lack of skilled resources and poor metadata management can further complicate operations. By adopting best practices, you can overcome these obstacles and ensure your data lake remains efficient and reliable.
Statistic Description |
Projected Adoption Rate |
Year |
---|---|---|
Enterprises adopting data lakehouse solutions |
60% |
2026 |
Enterprises deploying real-time analytics |
35% |
2025 |
Large enterprises implementing AI-driven lakes |
40% |
2025 |
Key Takeaways
-
Create a clear plan for your data lake. Focus on goals, how to bring in data, and organize it to match your company’s needs.
-
Set up strong rules to keep data correct and legal. Give clear jobs to people, check data quality, and review often.
-
Build your data lake to grow and work fast. Use smart storage methods and tools to make searches quicker and handle more data.
-
Use new tech like data lakehouses and AI tools. These make managing data easier and improve how you analyze it.
-
Add strong security, like controlling who can access data and encrypting it. Check and review often to keep private data safe.
Understanding Data Lakes
What Is a Data Lake?
A data lake is a centralized storage system that holds vast amounts of raw data in its original format. Unlike traditional storage systems, it does not require you to predefine a schema or structure before storing data. This flexibility allows you to store structured, semi-structured, and unstructured data, such as text files, images, and videos, all in one place.
Data lakes are designed to handle the growing complexity of modern data. They allow you to ingest data from multiple sources without the need for extensive preprocessing. This makes them ideal for organizations that need to analyze diverse datasets or support advanced analytics like machine learning and artificial intelligence.
Key Features of Data Lakes
Data lakes stand out due to their unique characteristics. Here are some of the defining features:
-
They store massive amounts of data, including structured, semi-structured, and unstructured formats.
-
The data remains in its raw state, preserving its original form for future use.
-
They support a wide range of analytics, such as batch processing, real-time stream computing, and machine learning.
-
They are highly scalable, allowing you to expand storage horizontally as your data grows.
Additionally, data lakes provide the flexibility to work with both fixed-format data and data that lacks a predefined structure. This adaptability ensures that your organization can handle evolving data requirements without significant reengineering.
Data Lakes vs. Data Warehouses
Data lakes and data warehouses serve different purposes, and understanding their differences can help you choose the right solution for your needs.
-
Data Lakes: These systems store raw, unstructured, and semi-structured data without requiring a predefined schema. They support both batch and real-time processing, making them suitable for machine learning, big data analytics, and exploratory analysis.
-
Data Warehouses: These systems focus on structured data with a predefined schema. They require data cleaning and transformation before ingestion. Their primary use cases include historical data analysis, business intelligence, and reporting.
In terms of performance, data lakes may have slower query speeds due to the unstructured nature of the data. However, they excel in handling diverse datasets and enabling advanced analytics. Data warehouses, on the other hand, are optimized for fast queries and structured data, making them ideal for generating business insights.
By understanding these differences, you can determine whether a data lake, a data warehouse, or a combination of both best suits your organization's goals.
Benefits of Data Lakes
Scalability and Flexibility
Data lakes provide unmatched scalability and flexibility for modern enterprises. Unlike traditional storage systems, a data lake can grow horizontally as your data needs expand. This means you can store vast amounts of structured, semi-structured, and unstructured data without worrying about infrastructure limitations. The schema-on-read architecture allows you to adapt to evolving data types without requiring upfront commitments.
You can also manage diverse datasets from multiple sources in a single repository. This unified approach eliminates data silos and ensures seamless integration across your organization. Distributed storage systems further enhance scalability, enabling dynamic growth as data volumes increase. Whether you need to store text, images, or videos, data lakes offer the flexibility to handle it all.
Cost-Effectiveness for Big Data
Data lakes are a cost-effective solution for managing large-scale data. They allow you to store unstructured data at a lower cost compared to traditional data warehouses. This affordability stems from their ability to use distributed storage systems, which reduce the need for expensive infrastructure.
While data warehouses prioritize query performance, they often come with higher costs. In contrast, data lakes provide economical storage for vast amounts of data, making them ideal for big data applications. Their scalability ensures you only pay for the storage you need, avoiding unnecessary expenses. This cost-efficiency makes data lakes a practical choice for organizations aiming to optimize their data management budgets.
Enabling Advanced Analytics and AI
Data lakes empower you to unlock the full potential of advanced analytics and AI. By serving as a centralized storage system, a data lake allows you to store structured, semi-structured, and unstructured data in one place. This flexibility is crucial for implementing AI-driven solutions.
You can use data lakes to support predictive analytics, where AI models analyze historical data to forecast future outcomes. They also enable natural language processing (NLP) for analyzing large volumes of text and image recognition for detecting patterns in visual data. These capabilities enhance decision-making and allow you to respond quickly to market trends. With data lakes, your organization can leverage innovative analytics to stay ahead in a competitive landscape.
Challenges in Data Lake Implementation
Governance and Compliance Issues
Governance and compliance present significant challenges when implementing a data lake. Without clear data ownership, accountability for maintaining data accuracy diminishes. This can lead to outdated or incorrect information, which undermines the reliability of your data lake. Additionally, regulations like GDPR and CCPA impose strict requirements for data handling. Poor governance complicates compliance, increasing the risk of audits, fines, and reputational damage.
To address these issues, you must establish a robust governance framework. Define clear roles and responsibilities for data ownership. Implement policies to ensure data accuracy and consistency. Regular audits can help you monitor compliance and identify gaps. By prioritizing governance, you can reduce risks and maintain trust in your data lake.
Security and Access Control
Security is a critical concern for data lakes. These systems store vast amounts of sensitive information, making them attractive targets for cyberattacks. Without proper access control, unauthorized users can exploit vulnerabilities and compromise your data. To protect your data lake, you need to adopt a multi-layered security approach.
Start by segmenting your network to isolate sensitive data. Use firewalls to monitor traffic and restrict access to authorized users. Encrypt data both at rest and in transit to prevent unauthorized access. Implement role-based access control (RBAC) to limit user permissions based on their roles. Multi-factor authentication adds an extra layer of security, while continuous monitoring and anomaly detection help identify suspicious activities in real time. Regular backups and disaster recovery planning ensure data availability even during emergencies. These measures will safeguard your data lake and maintain its integrity.
Integration with Legacy Systems
Integrating a data lake with legacy systems often proves challenging. Legacy systems rely on outdated technologies, which complicates compatibility. Data lakes gather information from various sources, leading to inconsistent data formats and processing inefficiencies. These issues can hinder data ingestion and reduce the effectiveness of your data lake.
To overcome these challenges, you should adopt standardized data formats like JSON or CSV. These formats simplify data exchange and improve compatibility. Robust APIs can facilitate seamless communication between legacy systems and your data lake. Additionally, you must assess the scalability of your existing infrastructure. Upgrading outdated components may be necessary to handle the increased data volume. By addressing these integration challenges, you can ensure your data lake operates efficiently alongside legacy systems.
Risk of Data Swamps
A poorly managed data lake can quickly turn into a data swamp. This happens when data becomes disorganized, inaccessible, or unreliable. You may find yourself struggling to extract meaningful insights, which defeats the purpose of having a data lake in the first place.
Data swamps create several risks for your organization:
-
Decreased productivity: Data scientists spend more time searching for and cleaning data instead of analyzing it.
-
Poor decision-making: Disorganized data leads to inaccurate analytics, which can result in flawed business strategies.
-
Increased costs: Managing redundant or irrelevant data adds unnecessary expenses.
-
Regulatory risks: A lack of governance can lead to non-compliance with data protection laws.
-
Missed opportunities: Difficulty accessing relevant data can prevent you from capitalizing on market trends.
-
Reduced customer satisfaction: Disorganized data affects your ability to deliver personalized customer experiences.
-
Security risks: Inadequate security measures increase the likelihood of data breaches.
To avoid these pitfalls, you need to implement proactive measures. Start by establishing strong data governance. Clear guidelines for data handling ensure consistency and accountability. Standardizing data formats reduces inconsistencies, making it easier to process and analyze information. Metadata management is another critical step. By tagging data with relevant context and characteristics, you can improve its discoverability and usability.
Proper access controls and security measures are essential for protecting sensitive information. Role-based access ensures that only authorized users can interact with specific datasets. Regular maintenance, such as data cleaning and updates, prevents your data lake from becoming cluttered. These practices not only reduce risks but also enhance the overall efficiency of your data lake.
By addressing the risk of data swamps, you can maintain a clean, organized, and secure data lake. This ensures that your organization continues to benefit from accurate insights and advanced analytics.
Best Practices for Data Lake Implementation
Define a Clear Data Lake Strategy
A well-defined data lake strategy is essential for building a successful and efficient system. Start by identifying clear objectives for your data lake. These goals will guide your efforts and ensure alignment with your organization's needs. Next, focus on seamless data ingestion. Collect data from diverse sources in real-time or batch mode to maintain a steady flow of information.
Your strategy should include five key components:
-
Data Ingestion Layer: Collects data from various sources.
-
Storage Layer: Stores raw data in structured, semi-structured, and unstructured formats.
-
Processing Layer: Cleans and transforms data for analytics.
-
Data Management Layer: Organizes and governs data to ensure accuracy and security.
-
Consumption Layer: Provides access to data for analysis using BI tools.
Additionally, prioritize scalability and efficient management. These elements maximize the potential of your data assets and ensure long-term success.
Establish Robust Governance Frameworks
Strong governance frameworks are critical for managing your data lake effectively. Begin by defining clear goals and objectives for governance. Establish a dedicated data governance team to oversee the process. This team should create a framework that outlines policies for data handling, security, and compliance.
Understanding your data is another vital step. Implement data cataloging and metadata management to improve discoverability and accessibility. Data quality measures ensure the accuracy and reliability of your datasets. Security and privacy protocols protect sensitive information from unauthorized access. Regular education and communication help your team stay informed about governance practices. Finally, plan for continuous monitoring and improvement to adapt to changing requirements.
Design for Scalability and Performance
Designing your data lake for scalability and performance ensures it can handle growing data volumes and complex analytics. Optimize storage structures by using columnar formats like Apache Parquet or ORC. These formats improve query efficiency and reduce response times. Efficient indexing further enhances data retrieval, especially for semi-structured or unstructured data.
Invest in query optimization tools such as Apache Spark or Presto to boost performance. Address latency issues in real-time analytics by separating historical and real-time datasets. This approach minimizes delays and supports timely decision-making. By focusing on these design principles, you can create a data lake that meets your organization's performance needs.
Leverage Emerging Data Lake Solutions
Data Lakehouses for Unified Storage
Data lakehouses combine the strengths of data lakes and data warehouses into a single platform. They allow you to manage and analyze data more efficiently. These systems support both batch and real-time processing, making them ideal for modern analytics. By integrating the flexibility of data lakes with the structured processing capabilities of data warehouses, data lakehouses simplify data management. They also enhance performance and improve governance.
Leading companies like Databricks and Snowflake use advanced engines such as Apache Spark and Presto to power their data lakehouses. These tools enable real-time analytics and machine learning on a unified platform. With a data lakehouse, you can standardize data management and allow multiple users to access and manipulate data simultaneously. This approach reduces complexity and ensures seamless data movement.
AI-Driven Data Management Tools
AI-driven tools are transforming how you manage data lakes. These tools automate repetitive tasks like ETL (Extract, Transform, Load), freeing up your team to focus on strategic analysis. They also validate and clean data, ensuring high-quality datasets. AI can uncover hidden patterns in large datasets, enabling predictive analytics and real-time decision-making.
For example, natural language processing (NLP) helps analyze large volumes of text data, while pattern recognition identifies trends in visual or numerical datasets. AI tools also enhance security by automating data anonymization processes, helping you comply with regulations. By leveraging these tools, you can optimize your data lake operations and unlock advanced analytics capabilities.
Ensure Data Quality and Metadata Management
Maintaining data quality is essential for effective data lake implementation. Start by implementing a metadata catalog. This catalog describes data sources, structures, and classifications, making it easier to locate and use data. Regular audits help you review data quality and governance policies. These audits ensure your data remains accurate and reliable.
Establishing data lifecycle management policies is another critical step. Retain only relevant data and remove outdated or redundant information. This approach reduces clutter and improves efficiency. By following these best practices, you can maintain a clean and organized data lake that supports your analytics goals.
Implement Strong Security Measures
Role-Based Access Control
Role-based access control (RBAC) ensures only authorized users can access specific datasets. Assign roles based on job responsibilities and limit permissions accordingly. This approach minimizes the risk of unauthorized access and protects sensitive information. RBAC also simplifies user management, especially in large organizations with multiple teams.
Data Encryption and Monitoring
Data encryption is vital for securing your data lake. Encrypt data both during transmission and while stored to prevent unauthorized access. Continuous monitoring tools help detect suspicious activities in real time. Use anomaly detection systems to identify unusual patterns in data usage. These tools allow you to respond quickly to potential security breaches and maintain the integrity of your data lake.
Optimize Data Ingestion and Processing
Optimizing data ingestion and processing is critical for successful data lake implementation. Proper planning ensures your data lake remains efficient and avoids common pitfalls like data swamps. You should start by understanding the intended use of your data before ingestion. This approach helps you categorize datasets effectively and prevents them from becoming disorganized or unusable.
Data ingestion should be a priority early in the process. By doing so, you can avoid future access difficulties and optimize storage for analytic performance. For streaming event data, ensure exactly-once processing to maintain accuracy and reliability.
Here are some best practices for optimizing data ingestion and processing:
Best Practice |
Description |
---|---|
Planning for Data Ingestion |
Ensures data is stored properly and remains accessible for future use. |
Making Multiple Copies of Data |
Facilitates data recovery and guarantees availability during disruptions. |
Setting Retention Policies |
Manages the data lifecycle and reduces unnecessary storage costs. |
Using Metadata for Context |
Provides essential information about datasets, improving usability. |
Continuous Monitoring |
Maintains performance and prevents your data lake from becoming a swamp. |
Retention policies play a vital role in managing storage costs and the data lifecycle. By defining how long data should be retained, you can eliminate outdated or irrelevant information. Metadata management is equally important. Adding context to your data through metadata improves discoverability and usability, making it easier for teams to extract insights.
Continuous monitoring and optimization ensure your data lake performs at its best. Regularly review ingestion pipelines to identify bottlenecks or inefficiencies. Use automation tools to streamline repetitive tasks and maintain consistency. These steps will help you build a robust data integration process that supports your analytics goals.
Avoiding Common Pitfalls
Preventing Data Swamps
A data swamp occurs when your data lake becomes disorganized and unusable. To prevent this, you need to adopt proactive strategies that ensure your data remains clean and accessible. Follow these steps to maintain a well-structured data lake:
-
Establish strong data governance policies to guide data handling and ensure accountability.
-
Standardize data formats to minimize inconsistencies and simplify processing.
-
Use metadata management tools to improve data discovery and usability.
-
Conduct automated data quality checks during ingestion to catch errors early.
-
Enforce access controls to restrict unauthorized access and protect sensitive data.
-
Implement a data cataloging system to index data for easy retrieval.
-
Schedule regular audits and cleanup processes to remove outdated or irrelevant data.
By following these practices, you can avoid the risks of a data swamp, such as reduced productivity, poor decision-making, and increased costs. A clean and organized data lake ensures your team can extract meaningful insights efficiently.
Avoiding Over-Complex Architectures
Overly complex architectures can hinder the performance of your data lake. As your system grows, you may encounter bottlenecks, slower query execution, and delays in data retrieval. These issues can disrupt decision-making and reduce operational agility.
To avoid these challenges, focus on simplicity and optimization. Here are some key steps:
-
Clearly define your use cases and requirements before designing the architecture.
-
Establish robust governance policies to maintain control over data management.
-
Implement effective metadata management to streamline data organization.
-
Regularly assess and optimize your architecture to address inefficiencies.
Avoid over-engineering your system by prioritizing functionality over unnecessary features. A well-optimized architecture ensures your data lake remains efficient and scalable as your needs evolve.
Ensuring Stakeholder Collaboration
Collaboration among stakeholders is essential for the success of your data lake project. To foster effective teamwork, you need to create an environment where everyone can contribute without conflicts.
-
Enable simultaneous teamwork by allowing multiple teams to work on data assets together.
-
Adhere to data governance policies to protect privacy and ensure proper access.
-
Share specific data versions during discussions to maintain clarity and consistency.
-
Track changes made to data to promote transparency and accountability.
When you empower stakeholders with self-serve infrastructure, they can explore data independently and generate insights without relying on others. This approach encourages innovation and ensures your data lake delivers value across your organization.
Balancing Cost and Performance
Balancing cost and performance is essential when implementing a data lake. You need to ensure that your system delivers high performance without exceeding budget constraints. Achieving this balance requires strategic planning and efficient resource management.
Start by optimizing your storage structures. Use columnar formats like Apache Parquet to improve query efficiency and reduce storage costs. These formats allow you to retrieve data faster while minimizing the space required to store it. Efficient indexing further enhances performance by speeding up data retrieval. This approach ensures that your data lake remains responsive even as data volumes grow.
Cloud-based storage solutions offer another way to balance cost and performance. These platforms provide scalability, allowing you to expand storage as needed without investing in expensive hardware. You only pay for the resources you use, which helps control costs. Compression and deduplication techniques also save storage space by eliminating redundant data. These methods reduce expenses while maintaining the integrity of your datasets.
To align your data lake with business objectives, prioritize value-driven projects. Focus on initiatives that deliver measurable benefits to your organization. Foster a cost-conscious culture by promoting accountability and transparency among your team. Regular audits and monitoring help you optimize resource usage and identify areas for improvement. These practices ensure that your data lake operates efficiently without unnecessary expenditures.
By implementing these strategies, you can build a data lake that balances cost and performance effectively. This ensures your system remains scalable, efficient, and aligned with your organization's goals.
The Future of Data Lakes
Trends in Data Lake Technology
Data lake technology is evolving rapidly, shaping how organizations manage and analyze data. By 2025, over 40% of large enterprises will implement AI-driven data lakes. These systems automate data ingestion, enhance query performance, and deliver advanced insights. By 2026, 60% of enterprises are expected to adopt data lakehouse solutions. This approach combines the flexibility of data lakes with the structured processing of data warehouses, enabling real-time analytics and simplifying data management. Additionally, 35% of enterprises will deploy real-time analytics platforms integrated with data lakes by 2025. This trend highlights the growing demand for faster insights and improved business outcomes.
To stay ahead, you must focus on integrating your data lake with emerging technologies. AI, machine learning, and IoT are transforming data lakes into central hubs for interconnected ecosystems. Managing security and compliance remains critical due to the sensitive nature of stored data. You can prevent chaos by implementing data cataloging and clear ownership policies. Sophisticated ETL pipelines ensure seamless integration with existing systems, creating a smart and efficient data environment.
Real-Time Analytics and Stream Processing
Real-time analytics is revolutionizing how you use data lakes. These systems capture data from sources like IoT devices and social media, enabling continuous processing as data is generated. An event-driven architecture supports immediate analysis, allowing you to respond quickly to changing conditions. For example, you can monitor customer behavior in real time and adjust your strategies accordingly.
The flexibility of real-time data lakes enhances decision-making. You can adapt your data strategies to current business scenarios, ensuring relevance and agility. This capability is essential for industries like finance, healthcare, and retail, where timely insights drive success.
Data Lakes as a Foundation for AI and Machine Learning
Data lakes play a vital role in supporting AI and machine learning applications. They provide a centralized repository for vast amounts of raw data, essential for training intelligent algorithms. AI can analyze historical data to predict future trends, such as sales patterns or customer preferences. Natural Language Processing (NLP) enables AI to process large volumes of text, while image recognition identifies patterns in visual data.
By leveraging data lakes, you can unlock the full potential of AI. These systems allow you to develop predictive models, automate decision-making, and enhance operational efficiency. A well-structured data lake ensures your AI initiatives remain scalable and effective.
Building a successful data lake requires you to follow best practices that ensure scalability, security, and efficiency. Start by exploring how a data lake will create value for your organization. Clearly defined goals, robust governance frameworks, and efficient data ingestion processes are essential. Test scalability and upgradability to future-proof your system. Use tools like data catalogs and metadata management to enhance discoverability and usability.
Emerging technologies play a pivotal role in shaping the future of data lakes. Cloud-based solutions offer cost-efficiency and scalability, while AI integration improves data processing and decision-making. A centralized repository eliminates silos, enabling seamless integration and advanced analytics.
FAQ
What is the difference between a data lake and a data lakehouse?
A data lake stores raw, unstructured data, while a data lakehouse combines the flexibility of a data lake with the structured processing of a data warehouse. A lakehouse supports real-time analytics and simplifies data management, making it ideal for modern use cases.
How can you prevent a data lake from becoming a data swamp?
You can prevent a data swamp by implementing strong governance, standardizing data formats, and using metadata management tools. Regular audits and cleanup processes also help maintain organization and usability. These steps ensure your data remains accessible and reliable.
Are data lakes suitable for small businesses?
Yes, data lakes can benefit small businesses by providing cost-effective storage for diverse datasets. Cloud-based solutions make data lakes scalable and affordable. They also enable advanced analytics, helping small businesses gain insights and improve decision-making without significant infrastructure investments.
What role does AI play in managing data lakes?
AI automates tasks like data cleaning, metadata tagging, and anomaly detection. It improves data quality and enhances security. AI also enables advanced analytics, such as predictive modeling and natural language processing, helping you unlock valuable insights from your data lake.
How do you ensure data security in a data lake?
You can secure a data lake by encrypting data at rest and in transit, implementing role-based access control, and using continuous monitoring tools. Regular audits and anomaly detection systems further protect sensitive information and maintain the integrity of your data.