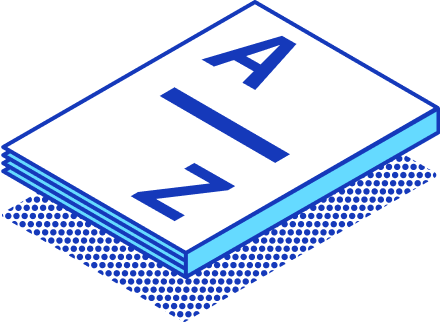
Big Data Analytics
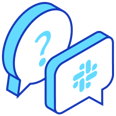
Join StarRocks Community on Slack
Connect on SlackWhat Is Big Data Analytics
Understanding Big Data
Big Data involves large and complex datasets that traditional tools cannot handle. These datasets include structured, unstructured, and semi-structured data. The growth of mobile technology and social media contributes to the volume of data. Big Data analytics describes the process of analyzing these vast datasets to extract valuable insights. Organizations use these insights to improve operations and make informed decisions.
The Role of Analytics in Big Data
Analytics plays a crucial role in processing and interpreting Big Data. Businesses use analytics to uncover patterns, trends, and correlations within data. This process helps organizations optimize operations and enhance customer engagement. Big data analytics refers to the methods and tools used to achieve these goals. Companies rely on analytics to gain a competitive advantage and drive innovation.
Key Characteristics of Big Data
Volume, Velocity, Variety, Veracity, and Value
Big Data has five key characteristics:
-
Volume: The sheer amount of data generated every second.
-
Velocity: The speed at which new data is created and processed.
-
Variety: The different types of data, including text, images, and videos.
-
Veracity: The accuracy and reliability of the data.
-
Value: The potential insights and benefits derived from data analysis.
These characteristics define how organizations approach Big Data Analytics.
How these characteristics impact analytics
The characteristics of Big Data impact analytics in several ways. High volume requires scalable storage solutions and efficient processing methods. Rapid velocity demands real-time analytics to keep up with data flow. Diverse data types necessitate advanced tools for integration and analysis. Ensuring data veracity involves maintaining data quality and accuracy. Extracting value from data requires sophisticated analytics techniques to derive actionable insights.
How Big Data Analytics Works
Data Collection and Storage
Sources of Big Data
Big Data originates from a multitude of sources. Social media platforms generate vast amounts of unstructured data. Mobile devices contribute to the continuous flow of information. The Internet of Things (IoT) adds to the complexity with real-time data streams. Organizations must tap into these diverse sources to harness valuable insights. Analyzing big data from these origins helps in understanding customer behavior and market trends.
Storage Solutions and Technologies
Efficient storage solutions are crucial for managing Big Data. Traditional databases struggle with the volume and variety of datasets. Modern technologies like Hadoop and NoSQL databases provide scalable options. Cloud-based solutions offer flexibility and cost-effectiveness for storing large datasets. Augmented Data Quality Solutions ensure data integrity and reliability. Organizations rely on these technologies to maintain seamless data access and processing.
Data Processing and Analysis
Techniques and Methods
Big Data Analytics involves various techniques to process information. Data mining uncovers patterns and correlations within datasets. Predictive analytics forecasts future trends based on historical data. Machine learning algorithms enhance decision-making by learning from data. These methods form the backbone of big data analytics processes. Organizations use these techniques to optimize operations and improve customer experiences.
Real-time vs. Batch Processing
Real-time processing offers immediate insights from data streams. This approach suits scenarios requiring instant decision-making. Batch processing handles large volumes of data at scheduled intervals. Organizations use batch processing for comprehensive analysis over time. Both methods play vital roles in big data ecosystems. Choosing the right approach depends on specific business needs and objectives.
Tools and Technologies in Big Data Analytics
Popular Tools
Hadoop, Spark, and NoSQL Databases
Hadoop stands as a cornerstone in the realm of Big Data. This open-source framework excels at storing and processing large datasets. Organizations rely on Hadoop to handle both structured and unstructured data efficiently. The framework's ability to manage vast amounts of information makes it indispensable for Big Data Analytics.
Spark offers a faster alternative to Hadoop. This tool uses random access memory (RAM) for data processing, which speeds up analytics tasks. Spark supports a wide range of data analytics queries and tasks. Many businesses prefer Spark for its efficiency and speed in handling Big Data.
NoSQL Databases provide flexible storage solutions. These databases accommodate diverse data types, making them ideal for Big Data environments. NoSQL databases support scalability and performance, essential for managing large datasets. Businesses use these databases to store and retrieve data quickly.
Data Visualization Tools
Data visualization tools transform complex data into understandable visuals. These tools help users identify patterns and trends in Big Data. Visual representations make data insights accessible to a broader audience. Tools like Tableau and Power BI lead the market in data visualization. These platforms enable users to create interactive dashboards and reports. Businesses use these tools to communicate data-driven insights effectively.
Emerging Technologies
Machine Learning and AI in Big Data
Machine learning enhances Big Data Analytics by automating data analysis. Algorithms learn from data to make predictions and identify patterns. Artificial intelligence (AI) further augments data analytics capabilities. AI systems process large datasets to uncover valuable insights. Businesses leverage machine learning and AI to optimize operations and drive innovation.
Cloud-based Analytics Solutions
Cloud-based analytics solutions offer flexibility and scalability. Platforms like Azure provide comprehensive big data analytics technology. Azure Data services enable real-time data processing and analysis. Azure Data Lake Analytics supports large-scale data operations. Cloud solutions reduce infrastructure costs and improve accessibility. Businesses adopt cloud-based solutions to streamline their Big Data Analytics lifecycle.
Benefits of Big Data Analytics
Business Insights and Decision Making
Big data analytics enhances decision-making processes. Businesses gain valuable insights from vast datasets. These insights improve customer experience and operational efficiency. Companies analyze data to understand customer preferences. This understanding leads to personalized services and increased satisfaction.
Enhancing Customer Experience
Big data brings big improvements to customer interactions. Businesses use data to tailor marketing strategies. Personalized recommendations increase customer engagement. Companies analyze feedback to enhance product offerings. Improved customer experience leads to loyalty and retention.
Optimizing Operations and Reducing Costs
Big data analytics makes operations more efficient. Companies identify inefficiencies through data analysis. Streamlined processes reduce operational costs. Data-driven decisions lead to resource optimization. Cost savings result from improved operational strategies.
Innovation and Competitive Advantage
Big data analytics products drive innovation. Companies leverage data to develop new offerings. Data insights reveal emerging market trends. Businesses stay ahead by adapting to changing demands. Innovation fosters a competitive edge in the market.
Driving Product Development
Data plays a crucial role in product development. Companies analyze consumer behavior for insights. These insights guide the creation of new products. Data-driven development meets customer needs effectively. Successful products result from informed design choices.
Identifying New Market Opportunities
Big data analytics important for identifying opportunities. Companies explore untapped markets through data analysis. Insights reveal potential areas for expansion. Businesses capitalize on new opportunities for growth. Market exploration leads to increased revenue streams.
Challenges in Big Data Analytics
Data Privacy and Security
Protecting Sensitive Information
Big data analytics involves handling vast amounts of personal and sensitive information. Organizations must prioritize protecting this data to maintain trust. Implementing robust security measures ensures that unauthorized access does not occur. Encryption and access controls play a vital role in safeguarding data. Regular audits help identify potential vulnerabilities in data systems.
Compliance with Regulations
Organizations face the challenge of complying with various data protection regulations. Laws like the General Data Protection Regulation (GDPR) require strict adherence. Companies must understand these legal frameworks to avoid penalties. Clear data usage policies ensure compliance with regulatory standards. Training employees on data privacy laws enhances organizational readiness.
Technical and Organizational Challenges
Data Quality and Integration
Ensuring high-quality data presents a significant challenge in big data analytics. Inconsistent or inaccurate data leads to flawed insights. Organizations must establish processes for data cleansing and validation. Integrating data from multiple sources requires sophisticated tools. Effective data integration enhances the accuracy of analytical outcomes.
Skill Gaps and Resource Allocation
The demand for skilled professionals in big data analytics continues to grow. Many organizations struggle to find experts with the necessary skills. Investing in training programs helps bridge this gap. Allocating resources efficiently ensures that analytics projects succeed. Organizations benefit from hiring and retaining talented data analysts.
Applications of Big Data Analytics Across Industries
Healthcare
Personalized Medicine and Predictive Analytics
Big Data Analytics finds significant applications in healthcare. Personalized medicine tailors treatments to individual patients. Analyzing patient data helps identify risk factors for diseases. Predictive analytics forecasts health outcomes based on historical data. Healthcare providers use these insights to improve patient care. Early disease detection enhances treatment effectiveness.
Improving Patient Care and Outcomes
Improving patient care requires data-driven decisions. Big Data Analytics applications enhance healthcare delivery. Analyzing clinical data helps optimize treatment plans. Patient outcomes improve with personalized interventions. Healthcare systems benefit from efficient resource allocation. Data insights lead to better patient experiences.
Finance
Fraud Detection and Risk Management
Financial institutions rely on Big Data Analytics for fraud detection. Analyzing transaction patterns identifies suspicious activities. Early detection prevents financial losses. Risk management strategies benefit from data insights. Financial organizations make informed decisions to mitigate risks. Data-driven approaches enhance security measures.
Enhancing Customer Experience
Enhancing customer experience remains a priority in finance. Big Data Analytics applications provide valuable insights into customer behavior. Financial services personalize offerings based on data analysis. Customer satisfaction increases with tailored financial products. Data insights guide strategic planning for customer retention. Financial institutions build stronger relationships with clients.
Retail
Inventory Management and Demand Forecasting
Retailers use Big Data Analytics for inventory management. Analyzing sales data optimizes stock levels. Demand forecasting predicts future product needs. Retailers reduce costs with efficient supply chain management. Big supply chain analytics ensures timely product availability. Data-driven strategies improve operational efficiency.
Personalized Marketing and Customer Insights
Personalized marketing enhances customer engagement in retail. Analyzing customer data reveals preferences and trends. Retailers tailor marketing campaigns to individual needs. Customer insights drive product development and pricing strategies. Big Data Analytics applications influence customer acquisition and retention. Retailers gain a competitive edge with data-driven decisions.
Conclusion
Big Data Analytics holds immense value in today's digital world. Organizations leverage analytics to drive innovation and gain a competitive edge. The impact of data-driven insights transforms industries and enhances decision-making processes. You should explore additional resources to stay informed about industry trends. Engaging with the latest developments ensures that you remain at the forefront of technological advancements. Share your thoughts or questions to foster a collaborative learning environment.