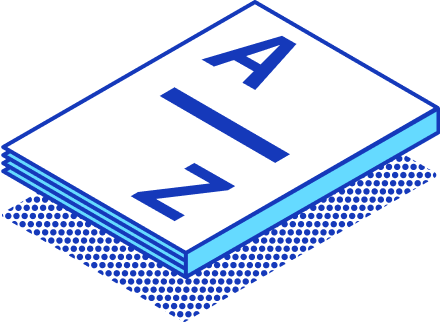
Data Governance
What Is Data Governance?
Data Governance refers to a comprehensive set of management practices within an organization that ensure the effective and efficient use of data. Typically led by an enterprise data governance team, it involves the creation and implementation of policies and processes that guide both the business and technical management of data across the organization. The quality of data directly impacts its value, influencing not only the outcomes of data analysis but also the accuracy of decisions made based on that data. In today’s data-driven world, making informed decisions relies on high-quality data. However, poor or inaccurate data can lead to misguided conclusions and suboptimal decision-making. Data governance is essential for enhancing data quality, unlocking the full potential of data as a valuable asset.
What Are the Main Objectives of Data Governance?
The primary objective of data governance is to create a structured framework for managing data as a valuable enterprise asset. This framework supports several critical goals:
-
Establish Internal Data Usage Rules: Data governance sets clear policies and standards for how data is collected, stored, and used across the organization. This ensures consistency, accountability, and compliance with internal and external requirements, creating a strong foundation for reliable data management.
-
Enhance Data Quality: By improving both data accuracy and consistency, data governance ensures that data is clean, reliable, and actionable. Validation processes, standardization, and regular audits help maintain high data quality, enabling accurate decision-making and operational efficiency.
-
Reduce Risk: Through stringent controls and oversight, data governance reduces data-related risks such as breaches, inaccuracies, and misuse. Proactively managing these risks helps avoid financial, legal, and reputational damage, safeguarding the organization.
-
Improve Communication: High-quality, accessible data improves both internal and external communication. Data governance fosters seamless collaboration by ensuring that all stakeholders work with accurate and consistent data, driving better coordination and more effective decision-making.
-
Ensure Compliance: Data governance ensures adherence to regulatory requirements such as GDPR, HIPAA, and industry-specific standards. This reduces legal risks, protects sensitive information, and fosters trust with customers and partners by maintaining compliance across all data practices.
-
Facilitate Data Management: A well-governed data environment simplifies the management of data across its lifecycle. Governance structures streamline data access, storage, and usage, making it easier for organizations to efficiently manage their data resources.
-
Increase Data Value: Data governance enhances the value of data by improving its quality, availability, and relevance. This turns data into a more powerful asset for driving business insights, innovation, and competitive advantage.
-
Ensure Long-Term Sustainability: Effective data governance helps organizations remain sustainable by optimizing data processes, managing risks, and enabling data-driven decision-making. This ongoing support ensures long-term growth and resilience.
-
Lower Costs: By reducing inefficiencies, eliminating redundant processes, and preventing errors caused by poor-quality data, data governance helps lower operational costs. This leads to more efficient use of resources and a stronger return on data investments.
In summary, data governance aims to build a structured, optimized approach to data management that aligns with the organization's broader goals of operational efficiency, compliance, innovation, and long-term sustainability.
What Are the Core Principles of Data Governance?
Data governance principles serve as a blueprint for managing data and facilitate decision-making around data storage, access, and usage.
Accountability
Accountability refers to the clear assignment of responsibility for data-centric activities and decisions within the organization. It ensures that individuals and teams understand their roles in managing and safeguarding data assets.
By holding individuals accountable for their duties, organizations can identify areas that need improvement and make the necessary adjustments, thereby strengthening data governance practices.
The most critical step in establishing accountability is integrating strategies and mechanisms such as data validation rules, data cleansing processes, and regular data audits. These mechanisms ensure data accuracy is maintained throughout its lifecycle, from collection and input to storage and usage.
Standardized Rules and Regulations
This principle refers to consistent guidelines and procedures for data management across the organization. Standardized rules create a uniform approach to handling data, such as using consistent methods for data input, access, and storage across different departments, eliminating discrepancies in practice.
Standardization begins with creating a common language—a centralized data glossary that serves as a single source of truth for data definitions. Additionally, establishing data classification schemes based on sensitivity, security requirements, and access control translates directly into actionable data validation rules.
Data Quality Standards
Data quality standards are internal guidelines within a data governance framework that ensure the data used in business processes is of high quality, reliable, and fit for its intended purpose.
When data quality standards are in place, employees can confidently use data to analyze trends, identify opportunities, and mitigate risks. High-quality data supports informed decision-making by providing reliable information to stakeholders across the organization.
Achieving data quality requires focusing on key dimensions such as accuracy, completeness, and timeliness. To support these dimensions, organizations should establish both manual and automated data cleansing procedures to address existing inaccuracies and schedule regular cleaning activities.
Data Compliance
In data governance, organizations must adhere to all applicable data laws, regulations, and industry standards. An effective data governance program must include comprehensive policies and procedures to ensure ongoing compliance.
These policies should cover all aspects of data collection, processing, storage, transmission, disposal, access, and privacy. Additionally, compliance strategies should be adaptable and responsive to changing regulations.
To ensure data compliance, organizations should conduct regular audits, risk assessments, and compliance checks to verify adherence to all relevant laws and regulations. Additionally, employees should receive regular training on data compliance, embedding it into the organizational culture.
Transparency
Data transparency in governance refers to making all data governance processes and operations clear and accessible to stakeholders across the organization.
Transparent governance processes ensure that stakeholders understand how data is managed, who is responsible for it, and who has access to it. This understanding fosters accountability and ensures everyone within the organization is aware of their roles and responsibilities in data governance.
Achieving transparency in a data governance framework involves three key steps:
- Develop clear and easily accessible data policies that outline data collection practices, usage guidelines, and user permissions.
- Establish communication channels to keep stakeholders informed about data governance initiatives, such as updates to the data inventory and changes in data access agreements.
- Implement a transparent, documented process for data access requests that is readily available to all authorized personnel.
Conclusion
An effective data governance program ensures data accuracy and reliability, facilitating informed decision-making. Every organization needs a set of core principles or guidelines to structure its data governance strategy. Implementing these principles promotes a culture of accountability, ensures data quality and consistency, and establishes a solid foundation for data management. Organizations that adopt and regularly evaluate these principles position themselves for successful data governance.
What is a Data Governance Framework and Its Models?
In today's digital landscape, data has emerged as a key production factor, recognized by organizations as a critical strategic asset. However, many organizations struggle with how to harness data's productivity and create value from their data assets. This is where a data governance framework comes into play, serving as a foundational structure to manage and utilize data effectively, aligning it with business strategies.
A data governance framework provides organizations with a structured approach to managing data by defining policies, processes, roles, responsibilities, and metrics that ensure data is managed consistently, securely, and in alignment with the organization’s goals. This framework is strategic, not operational, and ensures that data assets are leveraged in ways that maximize their value and help drive innovation in business models.
Key components of a data governance framework include:
-
Establishing Data Governance Operational Models:
- Data governance must reflect the organization's business model, data value, and operational needs. Centralized, decentralized, and hybrid models can be employed, based on how data is used and the organization's regulatory and cultural context.
-
Setting Data Governance Principles and Policies:
- Clear guidelines, goals, and policies are developed to guide data management practices. These are crafted with input from various stakeholders and ensure alignment with broader business strategies.
-
Executing Data Management Projects:
- Data governance frameworks facilitate the planning and execution of data management projects that enhance organizational efficiency, reduce risks, and drive value from data assets. These projects often require cross-functional collaboration and prioritization by governance bodies.
-
Driving Change Management:
- Implementing a data governance framework often necessitates significant organizational changes. Ensuring buy-in from leadership and managing the cultural shift towards data-driven decision-making are crucial for success.
-
Compliance and Regulatory Adherence:
- An effective data governance framework ensures that all data management practices comply with industry standards and regulatory requirements (e.g., GDPR, HIPAA), thus reducing legal risks and enhancing data security.
Data Governance Models:
Several data governance models provide structured approaches to implementing governance strategies:
-
DAMA Data Management Body of Knowledge (DMBOK):
- DAMA defines data governance as the exercise of authority and control over data assets through planning, monitoring, and enforcement. It emphasizes creating a formalized governance process to make better decisions about data usage and maximize value from data assets.
-
Data Management Capability Maturity Model (DCMM):
- The DCMM framework defines data governance maturity across various domains, such as data strategy, governance, architecture, and security. It helps organizations assess their governance maturity level and create roadmaps for improvement.
-
Data Governance Institute (DGI) Framework:
- DGI’s framework outlines 10 core components of data governance, divided into governance rules and policies, people and organization, and ongoing processes. It emphasizes defining roles, responsibilities, and control mechanisms to ensure data quality and proper data management practices.
Key Elements in Data Governance Strategy Planning:
- Vision and Mission: Clearly define the organization’s vision for how data will be used and its mission in managing data.
- Goals and Metrics: Establish specific, measurable goals for data management and governance that align with the organization’s strategic objectives.
- Governance Structure: Define roles, responsibilities, and the organizational structure needed to support governance activities.
- Capabilities and Processes: Identify the necessary capabilities for effective data management, including tools, technologies, and workflows.
- Communication and Collaboration: Establish mechanisms for ongoing communication and collaboration between all stakeholders involved in data governance.
By using a well-defined data governance framework, organizations can strategically manage their data assets, enhance their data quality, reduce risks, and drive long-term business value.
Best Practices for Data Governance
-
Identify Data Challenges and Clarify Objectives Before implementing a data governance strategy, it is essential to understand the underlying business drivers and data challenges. Common issues such as data inconsistency, duplication, inaccuracy, and incomplete or outdated data can disrupt operations and communication across departments, leading to increased costs. The goal of data governance is to address these issues and create high-quality data that supports business objectives. To get started, organizations should clearly define their data governance goals, aligning them with overall business strategies.
Best Practices:
- Conduct interviews with key stakeholders and perform site visits to assess current data challenges.
- Develop a preliminary communication plan and project roadmap.
- Align data governance objectives with business growth and operational needs.
-
Analyze the Current Data Landscape Perform a comprehensive analysis of the organization's current data governance environment, including organizational structure, personnel, processes, and data quality. This step helps in identifying gaps in data management practices and highlights the areas that need improvement.
Best Practices:
- Conduct data governance assessments across departments, processes, and data management systems.
- Evaluate the adequacy of personnel, such as data analysts, modelers, and quality assurance teams.
- Identify specific data quality issues (e.g., inconsistency, inaccuracies, privacy concerns) and map out the root causes.
-
Assess Data Governance Maturity Use industry-standard maturity models (such as DCMM or CMMI DMM) to evaluate the current level of data governance in the organization. A maturity assessment helps in understanding how well data is managed, the effectiveness of data governance policies, and the integration of governance practices into business operations.
Best Practices:
- Utilize structured maturity models to objectively evaluate governance practices.
- Develop an action plan to address gaps, prioritizing areas that need immediate attention.
- Ensure that the assessment results guide the development of future governance strategies.
-
Conduct Root Cause Analysis of Data Quality Issues Data quality is a core focus of any governance effort. Often, data issues arise due to a mix of business, management, and technical challenges. Identify the root causes of these problems, as solving a few critical issues can significantly improve overall data quality.
Best Practices:
- Use problem-solving techniques such as SWOT analysis, 5W1H, and root-cause (fishbone) diagrams to identify the key causes of data quality issues.
- Prioritize the most impactful data quality issues and develop targeted solutions.
-
Evaluate Business Impact and Implementation Priorities After assessing the maturity and analyzing root causes, evaluate the business impact of addressing each issue. This includes determining the urgency, difficulty, and benefits of solving data challenges. Focus on those areas that have the highest business impact and align with organizational priorities.
Best Practices:
- Use a prioritization matrix (e.g., the Four Quadrant Model) to rank issues based on business impact and ease of implementation.
- Develop a clear strategy to address the most urgent and impactful governance issues.
-
Develop a Data Governance Roadmap The roadmap is a detailed, step-by-step plan for implementing data governance across the organization. It outlines the stages of implementation, sets goals for each stage, and specifies the timeline and resources needed to achieve those goals.
Best Practices:
- Create a phased implementation plan with clear objectives for each phase.
- Incorporate feedback loops for continuous evaluation and adjustment of the roadmap.
- Align short-term actions with long-term governance objectives.
-
Create a Detailed Implementation Plan Based on the roadmap, develop a detailed plan that includes key areas of governance such as data architecture, metadata management, data quality management, and data security. Additionally, ensure that the governance framework covers organizational structure, roles and responsibilities, governance processes, and technology requirements.
Best Practices:
- Define core governance areas such as master data management, metadata management, and data quality control.
- Set up cross-functional teams and allocate roles clearly to ensure smooth execution.
- Integrate data governance into the organization’s technology infrastructure and workflows.
-
Control and Monitor Implementation Effective data governance requires continuous monitoring and control throughout the implementation process. Track progress, quality, and costs closely to ensure that the governance initiatives are meeting their targets.
Best Practices:
- Employ project management best practices such as risk management and integrated project monitoring.
- Use tools like project plans, risk registers, and performance reports to keep implementation on track.
- Regularly review progress and adjust the strategy as needed.
-
Measure and Evaluate Governance Effectiveness Once the governance strategy is in place, regularly assess its effectiveness using key performance indicators (KPIs) related to data quality, timeliness, and completeness. Conduct evaluations to identify areas for improvement and ensure that governance initiatives continue to align with business objectives.
Best Practices:
- Develop a set of KPIs to monitor data quality, security, and governance compliance.
- Conduct regular assessments (weekly, monthly, or quarterly) to evaluate the performance of governance initiatives.
- Use visual tools such as dashboards to track and communicate governance results.
-
Ensure Continuous Improvement Data governance should be treated as an ongoing process, not a one-time project. Embed data governance into the day-to-day operations of the business to ensure continuous improvement in data quality and governance practices.
Best Practices:
- Establish a governance framework that evolves with the organization’s needs.
- Encourage cross-functional collaboration and ongoing training to maintain governance standards.
- Integrate data governance practices into everyday business processes to make data management an inherent part of operations.
By following these best practices, organizations can establish a robust data governance framework that not only addresses immediate data quality issues but also supports long-term business growth and innovation through data-driven decision-making.
Conclusion
Effective data governance ensures data quality, security, and compliance. Organizations benefit from improved decision-making and operational efficiency. Data ethics provides the foundation for defining right and wrong in data usage. Data governance implements these ethical principles.
Data governance remains crucial for modern enterprises. Robust frameworks enhance data integrity and trustworthiness. Organizations must prioritize data governance to stay competitive and compliant.
Implementing data governance practices requires commitment. Organizations should establish clear policies and roles. Continuous monitoring and improvement ensure long-term success. Prioritize data governance to unlock the full potential of data assets.
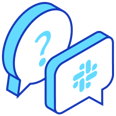
Join StarRocks Community on Slack
Connect on Slack