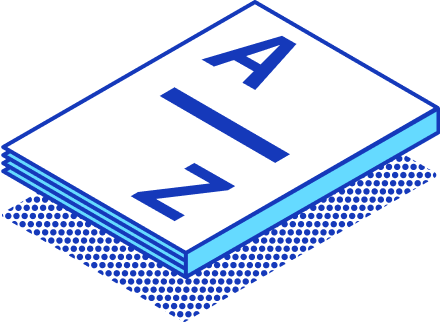
Data Quality
What Is Data Quality?
Data quality refers to the condition of data based on specific criteria. These criteria include accuracy, completeness, consistency, reliability, and validity. High-quality data meets these standards, ensuring that data serves its intended purpose effectively. Organizations rely on data quality to make informed decisions, conduct accurate analyses, and achieve operational efficiency.
Different industries define data quality according to their unique needs. In healthcare, data quality ensures patient records are accurate and complete. Financial institutions focus on data quality to comply with regulatory requirements and prevent fraud. Retail businesses prioritize data quality to manage inventory and improve customer satisfaction. Each industry tailors data quality standards to meet specific operational goals.
Historical Context
Evolution of Data Quality Concepts
The concept of data quality has evolved significantly over time. Initially, organizations focused on basic data entry accuracy. As data volumes grew, the need for comprehensive data quality management became apparent. Advances in technology introduced new methods for assessing and improving data quality. Today, data quality encompasses a wide range of practices and tools designed to maintain high standards across diverse data sets.
Key Milestones in Data Quality Management
Several key milestones have shaped the field of data quality management. The introduction of relational databases in the 1970s marked a significant advancement. These databases allowed for more efficient data storage and retrieval. In the 1990s, data warehousing and business intelligence tools emerged, enabling organizations to analyze large data sets. More recently, the rise of big data and machine learning has further transformed data quality practices. These technologies offer new ways to ensure data accuracy, completeness, and consistency.
Importance of Data Quality
Impact on Business Operations
Decision-Making
Accurate data quality plays a crucial role in business decision-making. High-quality data provides reliable insights, enabling organizations to make informed choices. Poor data quality can lead to incorrect conclusions and misguided strategies. For instance, inaccurate sales data can result in ineffective marketing campaigns. Businesses must invest in data quality tools to ensure accurate data for decision-making processes.
Operational Efficiency
Operational efficiency depends heavily on data quality. Efficient operations require accurate and consistent data. Poor data quality can cause operational errors, leading to delays and increased costs. For example, incorrect inventory data can disrupt supply chain management. By maintaining high data quality, businesses can streamline operations and reduce errors.
Regulatory and Compliance Requirements
Legal Implications
Data quality is essential for meeting regulatory and compliance requirements. Organizations must comply with various legal standards that mandate accurate and complete data. Non-compliance can result in hefty fines and legal actions. For instance, financial institutions must ensure data quality to prevent fraud and adhere to regulations. High-quality data helps organizations avoid legal repercussions.
Industry Standards
Different industries have specific standards for data quality. These standards ensure that data meets the required accuracy, completeness, and consistency levels. For example, healthcare providers must maintain accurate patient records to comply with industry regulations. Adhering to industry standards helps organizations build trust and credibility. High data quality ensures compliance with these standards, safeguarding the organization's reputation.
Customer Satisfaction and Trust
Data Accuracy
Data accuracy directly impacts customer satisfaction. Accurate data ensures that customers receive the correct information and services. Inaccurate data can lead to customer dissatisfaction and loss of trust. For example, incorrect billing information can frustrate customers. By prioritizing data accuracy, businesses can enhance customer satisfaction and loyalty.
Data Consistency
Consistency in data quality fosters customer trust. Consistent data ensures that customers receive uniform information across different channels. Inconsistent data can confuse customers and damage the organization's reputation. For instance, varying product information on different platforms can mislead customers. Maintaining data consistency helps businesses build a trustworthy relationship with their customers.
Key Elements of Data Quality
Accuracy
Definition and Importance
Accuracy refers to the correctness of data. Accurate data ensures that the information reflects real-world conditions. High accuracy in data quality is crucial for reliable decision-making. Organizations depend on accurate data to develop strategies, analyze trends, and measure performance. Inaccurate data can lead to poor decisions, resulting in financial losses and operational inefficiencies.
Methods to Ensure Accuracy
To ensure accuracy, organizations should implement several methods:
-
Data Validation: Regularly check data against known standards or rules.
-
Error Detection: Use software tools to identify and correct errors.
-
Training: Educate employees on proper data entry techniques.
-
Audits: Conduct periodic audits to verify data accuracy.
Completeness
Definition and Importance
Completeness refers to the extent to which all required data is present. Complete data ensures that no critical information is missing. High completeness in data quality allows businesses to perform comprehensive analyses. Incomplete data can result in gaps in understanding, leading to flawed conclusions and missed opportunities.
Methods to Ensure Completeness
To ensure completeness, organizations should adopt the following practices:
-
Data Profiling: Analyze data sets to identify missing elements.
-
Standardization: Establish clear guidelines for data collection.
-
Automation: Use automated tools to fill in missing data where possible.
-
Monitoring: Continuously monitor data to detect and address gaps.
Consistency
Definition and Importance
Consistency refers to the uniformity of data across different systems and platforms. Consistent data ensures that information remains the same regardless of where it is accessed. High consistency in data quality builds trust and reliability. Inconsistent data can cause confusion and lead to incorrect decisions.
Methods to Ensure Consistency
To ensure consistency, organizations should implement these strategies:
-
Data Integration: Combine data from multiple sources into a single, unified view.
-
Synchronization: Regularly update data across all systems to maintain uniformity.
-
Standard Formats: Use standardized formats for data entry and storage.
-
Validation Rules: Apply rules to ensure data remains consistent during processing.
Timeliness
Definition and Importance
Timeliness refers to the availability of data when needed. Data must be up-to-date and accessible at the right moment. Timely data ensures that businesses can make decisions based on the most current information. Without timely data, organizations risk making decisions on outdated or irrelevant data, leading to potential losses and missed opportunities. In industries like healthcare and finance, timeliness is crucial for compliance and operational efficiency.
Methods to Ensure Timeliness
To ensure timeliness, organizations should adopt several practices:
-
Real-Time Data Processing: Implement systems that process data in real-time to provide immediate access.
-
Regular Updates: Schedule frequent updates to keep data current.
-
Automated Alerts: Use automated alerts to notify stakeholders of data updates.
-
Data Integration: Integrate data from multiple sources to ensure comprehensive and up-to-date information.
Validity
Definition and Importance
Validity refers to the correctness and reasonableness of data within its context. Valid data adheres to defined formats, ranges, and constraints. Ensuring data validity is essential for maintaining the integrity of analyses and reports. Invalid data can lead to incorrect conclusions and flawed strategies. For example, invalid financial data can result in inaccurate financial statements, affecting business credibility.
Methods to Ensure Validity
To ensure validity, organizations should implement these strategies:
-
Validation Rules: Establish rules to check data against predefined criteria.
-
Data Cleansing: Regularly clean data to remove or correct invalid entries.
-
Consistency Checks: Perform checks to ensure data remains valid across different systems.
-
Training Programs: Educate employees on data entry standards and validation procedures.
Uniqueness
Definition and Importance
Uniqueness refers to the absence of duplicate records within a dataset. Unique data ensures that each record is distinct and not repeated. Maintaining uniqueness is vital for accurate data analysis and reporting. Duplicate records can skew results and lead to incorrect insights. For instance, duplicate customer records can result in inaccurate customer segmentation and targeted marketing efforts.
Methods to Ensure Uniqueness
To ensure uniqueness, organizations should adopt the following practices:
-
Duplicate Detection: Use software tools to identify and eliminate duplicate records.
-
Standardization: Standardize data entry processes to reduce the likelihood of duplicates.
-
Regular Audits: Conduct periodic audits to detect and resolve duplicate entries.
-
Data Matching: Implement data matching techniques to compare records and ensure uniqueness.
Conclusion
High-quality data remains essential for business success. Accurate, complete, and consistent data enables informed decision-making and operational efficiency. Organizations must prioritize data quality to avoid costly errors and enhance customer trust. Investing in robust data quality management tools and practices ensures data reliability. Businesses should consider adopting automated solutions for data validation, error detection, and duplicate elimination. Prioritizing data quality fosters a culture of trust and empowers teams to make strategic decisions. For further reading, explore resources on advanced data governance and data quality management techniques.
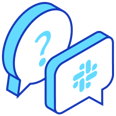
Join StarRocks Community on Slack
Connect on Slack