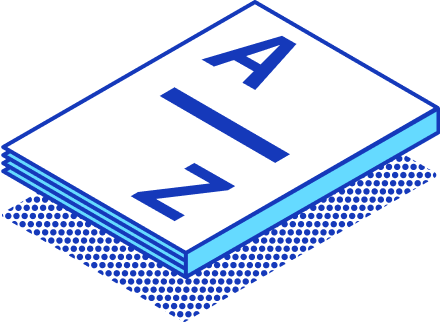
Retail Data Analytics
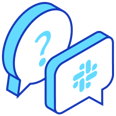
Join StarRocks Community on Slack
Connect on SlackWhat Is Retail Data Analytics
Definition and Explanation
Retail analytics involves using data to gain insights into retail operations. Retailers collect data from sales, inventory, and customer interactions. This data helps in making informed decisions.
Key Concepts
Key concepts in retail analytics include data collection, analysis, and interpretation. Retailers gather data from various sources like point-of-sale systems and online transactions. Analysis involves examining this data to identify patterns and trends. Interpretation helps in applying these insights to improve business strategies.
Historical Context
Retail analytics has evolved over time. Initially, retailers relied on basic sales reports. Over the years, technology advancements have enhanced data collection and analysis capabilities. Today, sophisticated tools provide deeper insights into consumer behavior and market trends.
Importance in Modern Retail
Retail analytics plays a vital role in modern retail. It transforms raw data into actionable insights that drive business success.
Enhancing Customer Experience
Retail analytics enhances customer experience by personalizing interactions. Data analysis helps retailers understand customer preferences and buying habits. This understanding allows for tailored marketing strategies and product recommendations. Improved customer satisfaction leads to increased loyalty and sales.
Optimizing Inventory Management
Retail analytics optimizes inventory management by predicting demand patterns. Accurate demand forecasting reduces overstock and stockouts. Retailers can maintain optimal inventory levels, minimizing costs and maximizing efficiency. Effective inventory management ensures product availability and meets customer expectations.
Types of Retail Data Analytics
Descriptive Analytics
Descriptive analytics provides a summary of past retail performance. Retailers use this type of analytics to understand sales trends and customer demographics. This helps in making informed decisions about consumer behavior and product popularity.
Sales Trends Analysis
Sales trends analysis involves examining historical sales data. Retailers identify patterns in sales over time. This helps in understanding which products are popular and when. Effective retail analytics enables better inventory management by predicting demand trends.
Customer Segmentation
Customer segmentation divides customers into distinct groups based on purchasing behavior. Retailers analyze data to identify these segments. This allows for targeted marketing and personalized promotions. Analytics data helps in tailoring strategies to meet the needs of different customer groups.
Predictive Analytics
Predictive analytics uses historical data to forecast future outcomes. Retailers apply this to anticipate customer behavior and market trends. This type of analytics supports strategic planning and decision-making.
Demand Forecasting
Demand forecasting predicts future customer demand. Retailers use analytics data to analyze past sales and market conditions. This helps in maintaining optimal inventory levels. Inventory analytics reduces overstocking and stockouts, improving cash flow and efficiency.
Customer Behavior Prediction
Customer behavior prediction anticipates how customers will act in the future. Retailers analyze data from previous interactions and purchases. This insight guides marketing strategies and product offerings. Predictive analytics enhances customer satisfaction and loyalty.
Prescriptive Analytics
Prescriptive analytics recommends actions to achieve desired outcomes. Retailers use this to optimize pricing and supply chain operations. This type of analytics provides actionable insights for business improvement.
Pricing Strategy Optimization
Pricing strategy optimization involves analyzing data to set competitive prices. Retailers consider factors like customer demand and competitor pricing. This ensures profitability while meeting customer expectations. Analytics data supports dynamic pricing strategies.
Supply Chain Efficiency
Supply chain efficiency focuses on streamlining operations. Retailers use analytics to diagnose issues and suggest improvements. This includes optimizing inventory levels and reducing costs. Inventory analytics ensures products are available when needed, enhancing overall efficiency.
Practical Applications of Retail Data Analytics
Personalization and Targeted Marketing
Retail analytics revolutionizes how retailers approach personalization and marketing. By analyzing customer data, retailers can create personalized recommendations that resonate with individual shoppers. This approach increases engagement and boosts sales.
Personalized Recommendations
Retail analytics empowers suppliers to tailor product suggestions based on customer preferences. By examining purchase history and browsing behavior, retailers can offer relevant products. This strategy enhances customer satisfaction and loyalty.
Targeted Promotions
Retail analytics enhances the effectiveness of promotions. Retailers can identify specific customer segments and craft targeted offers. This precision ensures that marketing efforts reach the right audience, maximizing return on investment.
Operational Efficiency
Retail business analytics drives improvements in operational efficiency. By leveraging data, retailers can optimize various aspects of their operations, leading to cost savings and enhanced performance.
Workforce Management
Retail analytics provides insights into workforce management. Retailers can analyze employee performance and scheduling needs. This data-driven approach ensures optimal staffing levels, reducing labor costs and improving customer service.
Store Layout Optimization
Retail analytics empowers suppliers to refine store layouts. By studying customer movement patterns, retailers can design more efficient spaces. This optimization leads to increased sales and a better shopping experience.
Best Practices in Retail Data Analytics
Data Collection and Management
Ensuring Data Quality
Retailers must prioritize data quality to gain accurate insights. High-quality data leads to better decision-making and customer satisfaction. Retailers should implement robust data validation processes. Regular audits help maintain data integrity. Data consistency across platforms ensures reliable analytics.
Ethical Considerations
Ethical data management builds trust with customers. Retailers should adhere to privacy regulations and protect customer information. Transparent data practices foster consumer confidence. Ethical considerations include obtaining consent for data use. Responsible data handling enhances brand reputation.
Implementation Strategies
Aligning with Business Goals
Retail analytics should align with business objectives. Retailers need to identify key performance indicators (KPIs) that support goals. Analytics tools should provide insights into these KPIs. Strategic alignment ensures analytics drive business success. Retailers can optimize operations by focusing on relevant metrics.
Continuous Monitoring and Improvement
Continuous monitoring enhances retail analytics effectiveness. Retailers should regularly review analytics outcomes. Ongoing analysis helps identify areas for improvement. Retailers can adapt strategies based on current data insights. Continuous improvement leads to increased efficiency and competitiveness.
Retail Analytics Best Practices enable retailers to harness data effectively. By ensuring data quality and ethical management, businesses can build trust and drive success. Aligning analytics with business goals and maintaining continuous monitoring ensures optimal performance. These practices distinguish retailers in a competitive market.
Future Trends in Retail Data Analytics
Innovations on the Horizon
Real-time Analytics
Real-time analytics represents a transformative shift in the retail industry. Retailers can now access data instantly, allowing them to make informed decisions quickly. This capability enhances customer experiences by providing timely product recommendations and personalized promotions. Real-time data analysis enables retailers to respond to market changes and consumer demands effectively. Retailers can optimize inventory levels and pricing strategies based on current sales trends. The ability to analyze data in real-time positions retailers to stay competitive in a fast-paced market.
Augmented Reality in Retail
Augmented Reality (AR) is revolutionizing the retail experience. Retailers use AR to create immersive shopping environments that engage customers. Shoppers can visualize products in their homes before making a purchase. This technology enhances customer satisfaction by providing a realistic view of products. Retailers can offer virtual try-ons for clothing and accessories. AR-driven analytics provide insights into customer preferences and behavior. Retailers can tailor marketing strategies and product offerings based on these insights. The integration of AR in retail analytics opens new avenues for innovation and growth.
Challenges and Opportunities
Data Privacy Concerns
Data privacy remains a significant challenge in retail analytics. Retailers must prioritize the protection of customer information. Compliance with privacy regulations builds trust with consumers. Retailers should implement robust security measures to safeguard data. Transparent data practices foster consumer confidence and loyalty. Addressing data privacy concerns is crucial for maintaining a positive brand image. Retailers can leverage analytics to enhance data security and ensure ethical data management.
Evolving Consumer Expectations
Consumer expectations continue to evolve in the digital age. Retailers must adapt to changing preferences and demands. Analytics provides valuable insights into consumer behavior and trends. Retailers can anticipate shifts in demand and adjust strategies accordingly. Personalization plays a key role in meeting consumer expectations. Retailers can use data to deliver tailored experiences that resonate with customers. The future of retail analytics involves understanding and exceeding consumer expectations. Retailers can achieve success by leveraging data-driven insights to enhance customer satisfaction.
Conclusion
Retail data analytics transforms retail operations by optimizing inventory management and enhancing decision-making. Businesses gain a competitive edge by adopting these tools, which provide insights into demand trends and operational efficiency. Retailers can explore further resources to deepen their understanding and leverage analytics for innovation and growth. Embracing analytics positions retailers to meet evolving consumer expectations and drive success in a dynamic market.