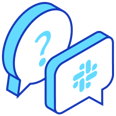
Join StarRocks Community on Slack
Connect on SlackReal-time data is wired, historical data is tired. Or is it?
Real-time data represents the future of analytics. So much so that it seems most industry analysts, including Intellyx—are constantly being asked to cover real-time data topics—because event-driven architectures are where the streaming edge of innovation seems to be happening these days.
Real-time represents reality. There’s significant business value to be gained, in terms of predictive accuracy and business agility, from only working with the freshest possible real-time data. It must be way more valuable than that old, historical data.
But wait a minute.
Technically, real-time data becomes historical data the very moment it is stored in a volume somewhere. So historical data doesn’t necessarily mean ‘old data’ anymore. Most of the data we deal with is actually historical, and will still be, for the known future.
After all, what good is knowing the velocity of a fast-moving object, if you have no data to understand where it just came from, and the trajectory it’s heading toward?
Why Historical Data Matters
Mark Twain once said that “History never repeats itself, but it does often rhyme.”
Perhaps industry analysts and experts have been lax in overlooking the value of bringing existing analytics applications and historical data to the table.
Take an e-commerce analytics example, where the only ‘real-time’ part of the data is new orders coming in through a payment gateway, or customer messages to a feedback app. There’s not much a digital business leader could take away from such information in terms of charting a future course.
Yes, the real-time data may tell the executive that there is some activity at this moment, but it’s hardly indicative of a trend unless this moment is compared to other ranges of similar times the business has existed in.
As a decision maker in the e-commerce company, I would need good historical data next to my real-time data for spotting cyclical or seasonal trends. More than that, I would also want to build models and scenarios for responding to events such as unexpected shifts in demand and inventory as well.
What’s Limiting Both Real-Time and Historical Data Value?
Any company that serves customers—and has managed to thrive over the last two decades—has largely done so thanks to finding an edge on information, so they can plan and react faster than their competition.
To maintain this edge companies built up extensive analytics capabilities and models, perhaps starting from core systems and SaaS platforms like Salesforce, and importing events and metrics to a Hadoop cluster, then adding several OLAP-style BI or reporting tools, as well as analytics packages such as Tableau or PowerBI.
Over time, as companies invested in analytics, they started to build up time-tested business models that could address their own industry and region-specific needs, while also accumulating extensive data stores and cloud data warehouses.
Newer noSQL and event-based backends like Kafka, Druid and Presto entered the picture to start to address the need for more current data that could be searchable at higher levels of detail.
As the enterprise-wide data model increases in scale, the performance of complex batch processes, queries and joins naturally starts to degrade. This puts the analytics owner in an uncomfortable situation of either accepting shortcomings in quality and speed, with increasing maintenance labor and cloud costs over time, or trying to modernize everything onto a new real-time system, with even bigger risks of losing existing analytics knowledge and switching costs.
Keep What Works, Make It Faster and Fresher
A data layer for real-time analytics should support signals from edge devices, system-level monitors, and third party services and feeds, but it shouldn’t be so specialized that it requires throwing away existing analytics models, with their underlying tabular data and batch architectures.
Figure 1. The data layer of a real-time analytics application needs to scale to support thousands of concurrent application processes and user queries. The time and cost of traditional data ingest, transfer, normalization, and access processes limit the potential uses of real-time and historical data. A business-responsive analytics data strategy allows fast queries and massive concurrency across both event-based and historical data sources, while eliminating unnecessary development and maintenance labor, and cloud storage and compute costs. (Source: Intellyx infographic, Jason English, 2023)
StarRocks offers an open source data layer that can optimize lookup times and normalization efforts and costs for both real-time and historical data, unlike other approaches that are specialized only for event-driven or batch architectures. The latest version handles asynchronous views and caches queries for better analytics performance atop Hudi and multiple data lake ecosystems.
By unifying analytics through a common, highly responsive data layer, businesses can double the value of real-time data by marrying it with the best analytics of historical data.
Meeting Guarantees at Airbnb
Airbnb, the born-in-the-cloud property sharing company, was seeking to better serve hosts and end customers to make sure everyone can meet promises of availability on listings–while also optimizing data usage across their whole network to improve their own analytics for planning and yield management.
With an existing analytics data table that could be as large as 10 TB in size, they were experiencing long processing, update and migration times.
A response time for a real-time fraud detection and prevention workload might be optimized for results within one second, while a broader investigation or strategic trends query used only occasionally by a handful of data scientists in planning could afford to allow a few more seconds of response time, given the amount of comparison to historical data sources.
Rather than rewrite from scratch, they decided to set multiple service level objectives for determining which ingestion use cases, latency and response times were acceptable for which use cases, by region and regulatory privacy regime.
They were able to do on-the-fly denormalization of tens of thousands of metrics from S3 storage and Iceberg data warehouses into the StarRocks’ native format at a fraction of the cost of prior methods using Presto and Druid. They could then use their Tableau dashboard to look at log-in data and detect and respond to fraud and identity threats in real time, ingesting Kafka and logging events, and supporting real-time SQL queries in approximately 3 seconds as opposed to 3 minutes to protect customers.
The Intellyx Take
It’s something all too often overlooked by technology vendors and pundits.
In today’s hyper-charged competitive environment where speed and agility mean everything, it’s all too easy to forget that real-time data alone isn’t worth as much unless it can be combined with historical data to respond better and faster to change than competitors.
Like how societies pass down their knowledge to future generations through schools and libraries, analytics models and data represent the institutional memory of a company.
Don’t throw away your existing batch architecture, when it can enrich the value of combined real-time analytics, and processing, in one higher performance data pipeline.
©2023 Intellyx LLC. Intellyx is editorially responsible for the content of this document. No AI bots were used to write this content. At the time of writing, StarRocks / CelerData is an Intellyx customer. Image sources: Infographic by Intellyx - Jason English; and “double down poker chips” on Craiyon.