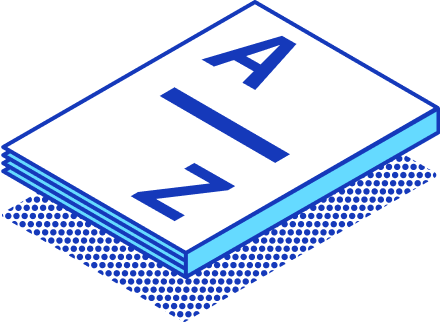
Behavioral Analytics
What Is Behavioral Analytics?
Behavioral Analytics involves the systematic collection and analysis of data from user interactions on digital platforms. This field focuses on understanding user actions, preferences, and behaviors to optimize digital experiences. Businesses use Behavioral Analytics to gain insights into user behavior, which helps in making informed decisions and improving customer satisfaction.
Key Metrics and KPIs
Key metrics in Behavioral Analytics include user engagement, retention rates, and conversion rates. Engagement metrics measure how users interact with a product, such as the number of sessions or time spent on a platform. Retention rates indicate the percentage of users who return to a product over a specific period. Conversion rates track the percentage of users who complete a desired action, such as making a purchase or signing up for a newsletter. These metrics help businesses understand user behavior and identify areas for improvement.
Methodologies
Data Collection Techniques
Data collection in Behavioral Analytics involves various techniques to gather user interaction data. Common methods include:
-
Clickstream Analysis: Tracks user clicks and navigation paths on a website or app.
-
Event Tracking: Monitors specific user actions, such as button clicks or form submissions.
-
Session Recording: Captures user sessions to analyze behavior patterns and identify usability issues.
-
Surveys and Feedback Forms: Collects direct input from users about their experiences and preferences.
These techniques provide comprehensive data that businesses can use to understand user behavior and improve digital experiences.
Data Analysis Methods
Data analysis in Behavioral Analytics involves several methods to interpret user interaction data. Key methods include:
-
Segmentation: Divides users into groups based on specific criteria, such as demographics or behavior patterns. This helps identify differences between user segments and tailor strategies accordingly.
-
A/B Testing: Compares two versions of a digital experience to determine which performs better. This method helps optimize user interactions and improve key metrics.
-
Funnel Analysis: Examines the steps users take to complete a specific action, such as making a purchase. This analysis identifies drop-off points and areas for improvement.
-
Predictive Analytics: Uses machine learning and statistical models to forecast future user behavior. This helps businesses anticipate user needs and make proactive decisions.
By employing these data analysis methods, businesses can gain valuable insights into user behavior and enhance their digital products.
Tools and Technologies in Behavioral Analytics
Popular Tools
Google Analytics
Google Analytics stands as a cornerstone in the realm of Behavioral Analytics. This service tracks website traffic and generates comprehensive reports. Businesses gain insights into customer demographics, interests, and visit sources. Detailed statistics on time spent on site and funnel analysis further enrich the data. Google Analytics excels in providing raw data that helps businesses understand user behavior. The platform's ability to track various metrics makes it indispensable for optimizing digital strategies.
Mixpanel
Mixpanel offers an advanced analytics platform designed to delve deeper into user interactions. Unlike Google Analytics, Mixpanel provides granular data on user activity. This includes detailed insights into funnels, segmentation, user paths, and product impact. Mixpanel proves particularly useful for examining the results of large campaigns with many small events or microtransactions. The tool enables product teams to track and analyze in-app product engagement, facilitating effective changes based on user behavior.
Emerging Technologies
AI and Machine Learning in Behavioral Analytics
Artificial Intelligence (AI) and Machine Learning (ML) are revolutionizing Behavioral Analytics. These technologies enable businesses to identify patterns, trends, and anomalies in user behavior data. AI and ML enhance predictive analytics, allowing companies to forecast future user actions. This proactive approach helps businesses anticipate user needs and tailor their strategies accordingly. The integration of AI and ML in Behavioral Analytics provides deeper insights, driving more informed decision-making processes.
Applications of Behavioral Analytics
E-commerce
Customer Journey Mapping
Behavioral Analytics plays a crucial role in mapping the customer journey. Businesses track user interactions across various touchpoints on digital platforms. This tracking helps identify the steps users take from initial contact to final purchase. By understanding these steps, companies can pinpoint areas where users drop off or face challenges. This insight allows businesses to optimize the customer journey, ensuring a smoother and more enjoyable experience. The ultimate goal is to increase conversion rates and customer satisfaction.
Personalization and Recommendations
Personalization has become a key strategy in e-commerce. Behavioral Analytics enables businesses to tailor experiences based on individual user behavior. Companies analyze data such as browsing history, past purchases, and interaction patterns. This analysis helps create personalized recommendations that resonate with each user. For instance, an online retailer might suggest products similar to those a customer has previously viewed or purchased. Personalized recommendations enhance user engagement and drive higher sales. Behavioral Analytics ensures that each user receives a unique and relevant shopping experience.
Digital Marketing
Campaign Optimization
Marketing and advertising firms leverage Behavioral Analytics to optimize campaigns. By analyzing user behavior data, marketers gain insights into what works and what doesn't. This data-driven approach allows for real-time adjustments to campaigns. For example, if a particular ad is not performing well, marketers can modify it or replace it with a more effective one. Behavioral Analytics helps in identifying the best-performing channels and strategies. This optimization leads to more effective campaigns and better return on investment (ROI).
Audience Segmentation
Audience segmentation is another critical application of Behavioral Analytics in digital marketing. Marketers divide the audience into segments based on specific criteria such as demographics, interests, and behavior patterns. This segmentation allows for more targeted and personalized marketing efforts. For instance, a company might create different marketing messages for new customers versus returning customers. Behavioral Analytics provides the data needed to understand these segments deeply. Targeted marketing efforts result in higher engagement rates and improved campaign performance.
Benefits of Behavioral Analytics
Business Insights
Improved Decision Making
Behavioral Analytics provides businesses with a wealth of data on user actions. This data helps companies make informed decisions. By analyzing user interactions, businesses can identify patterns and trends. These insights guide strategic planning and operational adjustments. Companies can validate their business decisions with concrete data. This reduces the risk of costly mistakes and enhances overall decision-making processes.
Enhanced Customer Experience
Understanding user behavior allows businesses to enhance customer experiences. Behavioral Analytics reveals customer preferences and pain points. Companies can use this information to tailor their products and services. Personalized experiences increase customer satisfaction and loyalty. Businesses can also identify areas for improvement in their digital platforms. This leads to a smoother and more enjoyable user experience.
Operational Efficiency
Resource Allocation
Behavioral Analytics helps businesses allocate resources more effectively. By understanding user behavior, companies can prioritize high-impact areas. This ensures that resources are directed towards initiatives that drive the most value. For example, businesses can focus on features that users engage with the most. This targeted approach maximizes the return on investment and improves operational efficiency.
Performance Tracking
Tracking performance is crucial for continuous improvement. Behavioral Analytics provides detailed metrics on user interactions. Companies can monitor key performance indicators such as engagement and conversion rates. This data helps businesses track the effectiveness of their strategies. Regular performance tracking enables businesses to make timely adjustments. This ensures that they stay on track to meet their goals.
Challenges and Considerations in Behavioral Analytics
Data Privacy and Security
Compliance with Regulations
Behavioral Analytics requires strict adherence to data privacy regulations. Laws such as the General Data Protection Regulation (GDPR) and the California Consumer Privacy Act (CCPA) mandate explicit consumer consent for data collection. Organizations must ensure that data collection practices comply with these regulations to avoid legal repercussions. Failure to comply can result in hefty fines and damage to the company's reputation. Businesses must implement robust data protection measures to safeguard personal information.
Ethical Considerations
Ethical considerations play a crucial role in Behavioral Analytics. Excessive data collection can lead to ethical concerns. Companies must balance the need for data with respect for user privacy. Transparency in data collection practices fosters trust between businesses and users. Organizations should inform users about the types of data collected and the purposes behind it. Ethical data handling practices enhance user trust and contribute to long-term business success.
Data Quality and Integration
Ensuring Data Accuracy
Accurate data is vital for effective Behavioral Analytics. Inaccurate data can lead to misleading insights and poor decision-making. Businesses must implement rigorous data validation processes to ensure data accuracy. Regular audits and quality checks help maintain data integrity. High-quality data enables organizations to derive meaningful insights and make informed decisions.
Integrating Multiple Data Sources
Integrating data from multiple sources poses a significant challenge in Behavioral Analytics. Different data sources may use varying formats and structures. Businesses must employ advanced data integration techniques to consolidate data from diverse sources. Tools like ETL (Extract, Transform, Load) processes facilitate seamless data integration. Effective integration ensures a comprehensive view of user behavior, leading to more accurate and actionable insights.
Conclusion
Behavioral analytics holds immense importance in today's digital landscape. Businesses must leverage behavioral analytics to drive growth, optimize conversions, and enhance customer retention. The future of behavioral analytics promises even deeper insights with advancements in AI and machine learning. Companies should invest in robust behavioral analytics tools to stay competitive and meet evolving customer needs.
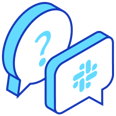
Join StarRocks Community on Slack
Connect on Slack