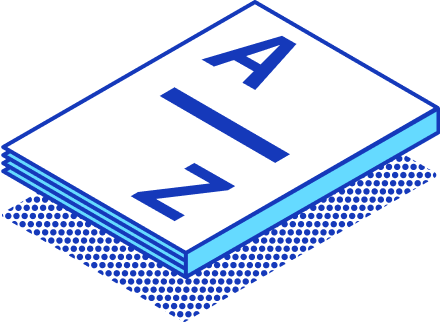
ETL (Extract, Transform, Load)
What is ETL?
How ETL Works:
-
Extraction: The initial phase of the ETL process involves extracting data from various source systems, which might include databases, CRM systems, ERP systems, and even flat files. This phase is critical since it involves identifying which data needs to be moved into the data warehouse.
-
Transformation: Once the data is extracted, it's not yet ready for analysis. There can be inconsistencies, redundancies, and errors in the raw data. The transformation phase addresses these. It can involve various operations, such as cleaning (removing anomalies or errors), filtering, splitting, summarizing, or merging datasets. The goal is to ensure that data is consistent, reliable, and suitable for analytics.
-
Loading: After transforming the data into a clean, reliable format, it's time to move it into its final destination: the data warehouse or another storage system. The loading phase ensures that data is correctly and efficiently inputted into the system, ready for analysis.
ETL vs. ELT:
-
Process Order:
-
ETL: Extract data from source systems, Transform the data externally using a dedicated engine, and then Load it into the target database.
-
ELT: Extract data, Load it directly into the target database, and then Transform the data within the database itself.
-
-
System Design:
-
ETL: Utilizes a separate data processing engine, often requiring a dedicated server.
-
ELT: Primarily relies on the database engine itself for data processing, ensuring the entire transformation process takes place within the database.
-
-
Efficiency & Scalability:
-
ETL: To improve efficiency, one might need to enhance the server's configuration or optimize the data processing flow.
-
ELT: Optimizations are primarily made within the database, benefiting from inherent database functions and enabling scalability through the database engine.
-
-
Data Flow:
-
ETL: Data flows from the source to an ETL tool and finally to the target data warehouse.
-
ELT: Data flows directly between source and target databases, with transformations happening at either end.
-
- Ideal Use Cases:
-
-
ETL: Best when complex calculations are needed, significant data cleaning is required before loading, or when dealing mainly with structured data.
-
ELT: Effective when leveraging database-specific optimizations, avoiding redundant data transfer, or optimizing parallel processing based on data distribution.
-
Designing a Robust ETL Process
Data Extraction
-
Understand the Source: Every data extraction begins by thoroughly understanding the source systems. Familiarize yourself with the structure, granularity, volume, and update frequency of each data source.
-
Incremental vs. Full Extraction: Based on the volume and update frequency, determine whether you need to perform a full extraction each time or if incremental extracts would suffice.
-
Diverse Data Sources: Keep in mind that data might come from varied sources like relational databases, flat files, APIs, or CRMs. Each might require a unique approach.
-
Real-time vs. Batch Extraction: Depending on the business requirements, you might need real-time data extraction or scheduled batch extractions. Each has its advantages and challenges, so choose wisely.
Data Cleaning
-
Data Validation: Establish a process that checks for data accuracy, consistency, and completeness. Ensure that any missing, outdated, or irrelevant data is identified.
-
Handling Duplicates: Implement algorithms to detect and eliminate duplicate records, ensuring the uniqueness and reliability of data.
-
Standardization: Convert data into a standard format. For instance, if date values are represented differently in source systems, unify them under a common format.
-
Error Handling: Establish a mechanism to handle anomalies. Rather than halting the entire ETL process upon encountering an error, the system should flag it and move on.
Data Transformation
-
Business Rules Application: Incorporate business rules to guide data transformations, ensuring that the transformed data aligns with specific analytical and operational requirements.
-
Aggregation and Summarization: Depending on the destination system and the intended use of the data, you might need to aggregate or summarize data points.
-
Data Enrichment: Consider adding value to your data by linking it with other datasets or adding derived attributes.
-
Scaling and Normalization: For data sets that will be used in machine learning or statistical models, scaling and normalization might be necessary to ensure that data points are on a comparable scale.
ETL Logging and Alerting
-
ETL Logs: The primary purpose of logging is to have real-time insights into the ETL process. If something goes wrong, logs can provide clues about the point of failure. ETL logs can be categorized into three main types:
-
Execution logs detailing every step.
-
Error logs specifying module failures and reasons.
-
Overall logs highlighting start times, end times, and success status.
-
-
Alerting Mechanisms: In case of ETL failures, not only should the system log the error, but it should also alert designated stakeholders. Common alerting mechanisms include sending emails to system administrators with detailed error descriptions, enabling them to troubleshoot effectively.
-
Log Retention and Review: Establish policies about how long logs should be retained. Periodic reviews of these logs can offer insights into potential areas of optimization or recurring issues that need attention.
ETL Workload Bottlenecks in Modern Data Lake Query Acceleration
In the quest to enhance data lake query efficiency, ETL workloads pose distinct challenges. The heart of these challenges lies in refining slow queries to keep pace with the demands of modern data analysis. To achieve this, data engineers are increasingly leveraging sophisticated pre-computation techniques. For instance, denormalization transforms normalized tables into expansive, flattened structures, effectively reducing join costs. Similarly, pre-aggregation targets the optimization of high-cardinality dimensions, a common bottleneck in query operations due to their computational intensity.
However, these advanced techniques bring about transformative implications for both data users and engineers. The introduction of new tables, a consequence of pre-computation, necessitates the meticulous rewriting of SQL queries. This task becomes even more daunting when dealing with complex queries, placing a significant responsibility on users to adapt and on engineers to anticipate these changes in the early stages of pipeline development.
The ripple effects of these strategies extend into the broader development cycle. The necessity for extensive planning and the potential creation of underutilized pre-computed tables may inflate costs, complicate testing procedures, and elongate the timeline from development to deployment. This reality underscores the importance of strategic foresight and meticulous planning in the ETL process.
Moreover, the landscape of query engine technologies is undergoing a rapid transformation. With query engines gaining speed, the traditional dependence on pre-computed data is diminishing, giving rise to more agile, on-demand ETL pipelines. This evolution prompts a critical reassessment of the planning phase in ETL pipeline construction, challenging engineers to strike a delicate balance between real-time computation and the utility of pre-computed data structures.
In essence, optimizing data lake queries is an intricate dance of efficiency and practicality. The fast-evolving nature of query engine technologies and the growing complexity of data workloads call for innovative, thoughtfully planned solutions to ensure the effectiveness and efficiency of ETL processes in this ever-changing digital landscape.
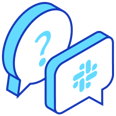
Join StarRocks Community on Slack
Connect on Slack