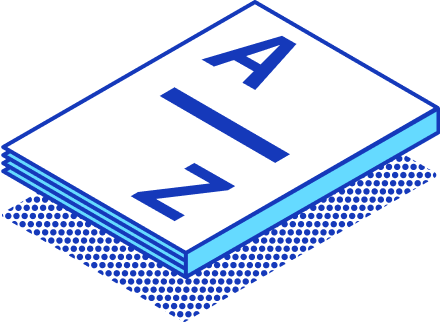
CPG Data Analytics
Understanding Data Analytics for Health Care
Basics of Data Analytics
Definition and Importance
Data analytics involves examining raw data to draw conclusions. Healthcare organizations use data analytics to improve patient outcomes. The process saves money, time, and lives. Descriptive analytics optimizes resource allocation and reduces waste. The approach enhances patient care by evaluating demographic information.
Key Concepts and Terminology
Data analytics includes several key concepts. Descriptive analytics evaluates past data to identify trends. Predictive analytics forecasts future events based on historical data. Prescriptive analytics suggests actions to achieve desired outcomes. Diagnostic analytics investigates the root causes of events.
Data Analytics in Healthcare
Applications and Benefits
Healthcare organizations apply data analytics in various ways. Predictive analysis forecasts disease progression. The method optimizes treatment plans. Descriptive analytics identifies patterns in disease outbreaks. Prescriptive analytics predicts admission rates and resource demands. The approach aids in creating precise cancer treatments based on genetic profiling.
Challenges and Solutions
Data analytics in healthcare faces several challenges. Data privacy concerns arise due to sensitive patient information. Ensuring data accuracy and consistency poses difficulties. Integrating data from multiple sources requires advanced technology. Solutions include robust data governance frameworks. Advanced encryption techniques protect patient information. Interoperability standards facilitate seamless data integration.
Transitioning to CPG Data Analytics
Overview of CPG Industry
Key Players and Market Dynamics
The Consumer Packaged Goods (CPG) industry includes a variety of key players. These players range from multinational corporations to small local businesses. Each player contributes to the dynamic nature of the market. The industry experiences constant change due to consumer preferences and technological advancements. Companies use CPG Data Analytics to navigate these changes effectively. This approach helps in understanding market trends and consumer behavior.
Importance of Data in CPG
Data plays a crucial role in the CPG industry. Companies rely on CPG Data Analytics to make informed decisions. This practice involves analyzing data to optimize operations and improve performance. Predictive insights help companies anticipate market trends. Competitor analysis aids in understanding market positioning. Customer psychographics provide insights into consumer preferences. Partnerships enhance data collection and analysis capabilities. CPG Data Analytics empowers companies to adapt to changing market conditions.
Types of Data in CPG
Observational Data
Observational data captures real-world scenarios. This type of data includes information gathered from direct observation. Companies use observational data to understand consumer interactions with products. CPG Data Analytics transforms this data into actionable insights. These insights inform product development and marketing strategies. Observational data helps identify patterns and trends in consumer behavior.
Activity Data
Activity data records consumer actions. This data includes information about purchasing habits and brand interactions. CPG Data Analytics uses activity data to analyze consumer behavior. This analysis helps companies tailor their marketing efforts. Understanding activity data allows for personalized consumer experiences. Companies can optimize inventory and supply chain operations based on activity data.
Sales Data
Sales data provides insights into product performance. This data includes information about sales volume and revenue. CPG Data Analytics leverages sales data to evaluate market success. Companies use sales data to identify top-performing products. This data informs pricing strategies and promotional efforts. Sales data analysis helps companies forecast future demand. Accurate sales data is essential for strategic planning in the CPG industry.
The Cyclical Process of Continuous Improvement
Real-Time Data and Strategic Planning
How Data Informs Decisions
Real-time data plays a crucial role in decision-making within the CPG industry. Companies gather insights from immediate data to understand market trends. This approach enables businesses to make informed strategic decisions. Real-time data allows companies to adapt quickly to consumer needs. Businesses can optimize marketing strategies and product development efforts.
Examples of Strategic Use
CPG companies utilize real-time data in various strategic ways. Retailers and CPGs collaborate using this data to strengthen relationships. This collaboration enhances decision-making processes. Companies use real-time data to stay ahead of market trends. Businesses can anticipate consumer demands and adjust their offerings accordingly. This proactive approach benefits both businesses and consumers.
Continuous Improvement Cycle
Feedback Loops
Feedback loops are essential in the continuous improvement cycle. Companies collect feedback from various sources to refine strategies. This process involves analyzing consumer responses and market performance. Feedback loops help identify areas for enhancement. Businesses use this information to improve products and services continuously.
Iterative Enhancements
Iterative enhancements focus on gradual improvements over time. Companies implement small changes based on data-driven insights. This method allows for constant refinement of strategies and operations. Iterative enhancements ensure that businesses remain competitive. Companies can adapt to changing market conditions and consumer preferences. This approach leads to sustained growth and success in the CPG industry.
Advanced Technologies in CPG Analytics
Role of AI and Machine Learning
Artificial Intelligence (AI) and Machine Learning (ML) play a significant role in enhancing CPG Data Analytics. AI tools process large datasets efficiently. These tools uncover patterns and trends that remain hidden to human analysts. Machine learning algorithms improve data analysis accuracy by learning from historical data. This leads to better decision-making in the CPG industry.
Enhancing Data Analysis
AI enhances data analysis by automating complex processes. AI systems analyze consumer behavior and market trends. This analysis provides valuable insights for CPG companies. AI-driven analytics optimize supply chain operations. Companies reduce costs and improve efficiency through AI applications. AI also aids in product development by predicting consumer preferences.
Predictive Analytics
Predictive analytics uses historical data to forecast future outcomes. CPG companies leverage predictive analytics to anticipate consumer demands. This approach helps in inventory management and demand forecasting. Predictive models identify potential sales opportunities. Companies use these insights to develop targeted marketing strategies. Predictive analytics enhances strategic planning in the CPG sector.
Case Studies and Real-World Examples
Repsly's Retail Execution Solution
Repsly's Retail Execution Solution demonstrates the power of CPG Data Analytics. Repsly empowers brands with actionable insights. The solution focuses on three key data types: Observational, Activity, and Sales. Repsly transforms raw data into insights that drive sales growth. Brands optimize in-store execution using Repsly's data-driven approach. The solution supports strategic planning with real-time data.
-
Observational Data: Captures real-world scenarios for product interactions.
-
Activity Data: Records consumer actions and purchasing habits.
-
Sales Data: Provides insights into product performance and revenue.
Repsly's solution includes a continuous improvement cycle. This cycle gathers field insights for strategic planning. Targeted actions enhance performance based on real-time data. Repsly equips brands to adapt to changing market conditions. The platform simplifies tracking Key Performance Indicators (KPIs). Brands measure progress against S.M.A.R.T. goals. Repsly's solution positions brands for long-term success.
Other Industry Examples
Other CPG companies also utilize advanced technologies. AI and ML uncover growth opportunities in the industry. Companies personalize products and campaigns at scale. This personalization enhances customer engagement. Data-driven segmentation aids in demand forecasting. Companies optimize supply chain operations with AI insights. Predictive analytics informs strategic decision-making. These technologies help CPG companies stay competitive.
Leveraging Data Analytics for Competitive Advantage
Strategies for Implementation
Best Practices
Data analytics can transform CPG companies. Successful implementation requires adherence to best practices. Companies should establish clear objectives for data use. Goals guide the collection and analysis of data. Organizations need to invest in robust data infrastructure. Reliable systems ensure accurate data processing. Training employees enhances data literacy across teams. Informed staff can make better decisions. Collaboration between departments fosters a data-driven culture. Shared insights lead to cohesive strategies.
Common Pitfalls to Avoid
Avoiding common pitfalls is crucial for effective data analytics. Companies often struggle with data silos. Isolated data limits comprehensive analysis. Integrating systems ensures seamless data flow. Over-reliance on historical data can mislead decision-making. Current trends must inform strategies. Ignoring data quality leads to inaccurate conclusions. Regular audits maintain data integrity. Companies should not underestimate the importance of skilled personnel. Expertise is essential for interpreting complex datasets.
Encouraging Adoption
Benefits for Brands
Data analytics offers numerous benefits for CPG brands. Insights from data drive product innovation. Understanding consumer preferences leads to tailored offerings. Analytics optimize supply chain operations. Efficiency reduces costs and enhances profitability. Brands can personalize marketing campaigns. Targeted strategies increase customer engagement. Predictive analytics aids in demand forecasting. Accurate predictions improve inventory management.
Survey Results:
-
69% of retailers reported increased revenue due to AI adoption.
-
72% experienced reduced operating costs through analytics.
Call to Action
CPG companies should embrace data analytics. The competitive landscape demands informed strategies. Brands must leverage data for sustained growth. Investment in technology enhances capabilities. Training programs empower employees with data skills. Collaboration fosters a unified approach to analytics. Companies should prioritize data-driven decision-making. The future of CPG lies in harnessing the power of data.
Conclusion
CPG data analytics holds immense value for businesses. Companies can achieve operational efficiency and cost reduction through insights. Data-driven strategies resonate with consumer behavior. Tailor-made approaches enhance marketing and sales efforts. Businesses gain a competitive edge by leveraging these insights. CPG analytics transforms supply chain performance. Companies can anticipate market changes and respond effectively. Data-driven decision-making sustains long-term success. Embracing analytics is crucial for thriving in the CPG industry.
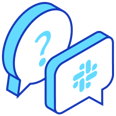
Join StarRocks Community on Slack
Connect on Slack