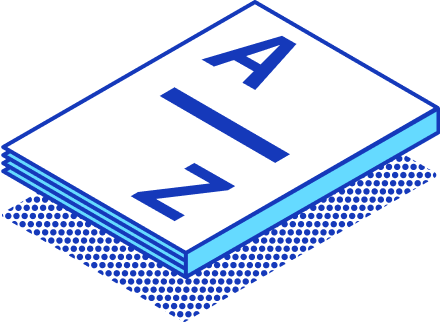
Data Blending
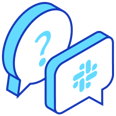
Join StarRocks Community on Slack
Connect on SlackWhat is Data Blending?
Data blending involves merging data from multiple sources to create a single, unified dataset. This technique allows analysts to perform comprehensive analyses by combining diverse datasets. Data blending emerged in late 2013 and has since enhanced efficiency and user experience. By integrating various data points, businesses can uncover deeper insights and trends.
Difference between Data Blending and Data Integration
Data blending and data integration often get confused, but they serve different purposes. Data integration involves combining data at the storage level, creating a single repository. In contrast, data blending merges data at the analysis level without altering the original datasets. Data blending allows for rapid analysis without the need for complex ETL (Extract, Transform, Load) processes.
Key Components of Data Blending
Data Sources
Data blending relies on multiple data sources. These sources can include databases, spreadsheets, cloud services, and APIs. Each source provides unique data points that contribute to a more comprehensive analysis. Internal data sources might include company databases, while external sources could involve social media or market research data.
Blending Techniques
Several techniques exist for data blending. Common methods include matching and merging data based on key identifiers. Analysts may use aggregation to summarize data before blending. Handling discrepancies between datasets ensures accuracy and consistency. These techniques enable seamless integration of diverse data types.
Tools and Software
Various tools facilitate data blending. Sisense, for example, allows users to combine data from multiple sources with ease. The intuitive interface and drag-and-drop functionality make it accessible for both technical and non-technical users. Other popular tools include Tableau and Alteryx, which offer robust data blending capabilities. These tools empower organizations to drive data-informed decision-making.
Steps to Perform Data Blending
Identifying Data Sources
Internal Data Sources
Internal data sources form the backbone of any data blending process. These sources include company databases, CRM systems, and ERP systems. Analysts often rely on these sources for accurate and relevant data. Internal data provides a wealth of information about business operations, customer interactions, and financial transactions.
External Data Sources
External data sources complement internal data by offering additional perspectives. These sources can include social media platforms, market research reports, and public datasets. External data helps analysts understand broader market trends and customer sentiments. Combining internal and external data enhances the depth and breadth of analysis.
Preparing Data for Blending
Data Cleaning
Data cleaning ensures the accuracy and consistency of datasets. This step involves removing duplicates, correcting errors, and standardizing formats. Clean data forms the foundation for reliable analysis. Tools like Alteryx and Tableau offer robust data cleaning functionalities. These tools streamline the process and reduce manual effort.
Data Transformation
Data transformation converts raw data into a suitable format for analysis. This step may involve aggregating data, creating calculated fields, or normalizing values. Transformation makes data compatible across different sources.
Blending the Data
Matching and Merging Data
Matching and merging data involves combining datasets based on common identifiers. This step creates a unified dataset for comprehensive analysis. Analysts use key fields such as customer IDs or product codes for matching.
Handling Data Discrepancies
Handling data discrepancies is crucial for maintaining accuracy. Discrepancies arise from differences in data formats, missing values, or conflicting records. Analysts must resolve these issues to achieve a consistent dataset. Tableau and other data blending software offer features to identify and rectify discrepancies. These tools enhance the reliability of blended data.
Benefits of Data Blending
Enhanced Data Insights
Improved Decision Making
Data blending enables organizations to combine diverse datasets, leading to more informed decision-making. By integrating data from various sources, analysts can uncover hidden patterns and trends. This comprehensive view allows businesses to make strategic decisions based on a holistic understanding of their operations. For instance, combining sales data with customer feedback can reveal insights into product performance and customer satisfaction.
Comprehensive Analysis
A unified dataset created through data blending facilitates comprehensive analysis. Analysts can evaluate multiple variables simultaneously, providing a deeper understanding of complex relationships. This approach enhances the ability to identify correlations and causations that might remain unnoticed when analyzing isolated datasets. For example, blending financial data with market research can offer valuable insights into economic trends and investment opportunities.
Time and Cost Efficiency
Streamlined Processes
Data blending streamlines the data preparation process, reducing the time required for analysis. Traditional methods often involve lengthy ETL processes, which can delay insights. Data blending bypasses these steps by allowing analysts to merge data at the analysis level. This efficiency enables quicker access to actionable insights, enhancing the agility of business operations.
Reduced Manual Effort
Automating the data blending process significantly reduces manual effort. Analysts no longer need to spend extensive time cleaning, transforming, and merging datasets. Advanced tools handle these tasks efficiently, freeing up resources for more critical analytical work. This reduction in manual labor not only saves time but also minimizes the risk of human error. Consequently, organizations can allocate their workforce to more strategic initiatives, driving innovation and growth.
Use Cases of Data Blending
Marketing and Sales
Customer Segmentation
Data blending enables marketers to create detailed customer segments. Combining data from CRM systems, social media platforms, and purchase histories provides a comprehensive view of customer behavior. This approach helps identify high-value customers and tailor marketing strategies accordingly. For example, blending demographic data with purchasing patterns reveals insights into customer preferences, allowing for targeted campaigns.
Campaign Analysis
Analyzing marketing campaigns becomes more effective with data blending. Marketers can merge data from email marketing tools, social media analytics, and sales records. This unified dataset offers a holistic view of campaign performance. By evaluating different metrics, such as click-through rates and conversion rates, marketers can optimize future campaigns. Data blending also helps in identifying the most effective channels and messages for reaching the target audience.
Finance and Accounting
Financial Reporting
Financial reporting benefits significantly from data blending. Accountants can combine data from various financial systems, including ERP and accounting software. This integration ensures accurate and comprehensive financial statements. Data blending allows for real-time financial analysis, improving decision-making processes. For instance, blending revenue data with expense reports provides a clear picture of profitability.
Risk Management
Risk management becomes more robust with data blending techniques. Financial analysts can merge data from market trends, historical financial records, and external economic indicators. This comprehensive dataset helps in identifying potential risks and developing mitigation strategies. By analyzing diverse data points, organizations can anticipate market fluctuations and make informed decisions. Data blending enhances the ability to manage financial risks effectively.
Healthcare
Patient Data Analysis
Healthcare providers use data blending to analyze patient data comprehensively. Combining electronic health records (EHR), lab results, and patient feedback offers a complete view of patient health. This approach helps in identifying trends and patterns in patient outcomes. For example, blending clinical data with patient demographics can reveal insights into disease prevalence and treatment effectiveness. Data blending improves patient care by enabling personalized treatment plans.
Operational Efficiency
Operational efficiency in healthcare improves through data blending. Administrators can merge data from scheduling systems, resource management tools, and financial records. This unified dataset helps in optimizing hospital operations and reducing costs. By analyzing various operational metrics, healthcare providers can identify bottlenecks and streamline processes. Data blending facilitates better resource allocation and enhances overall efficiency.
Challenges in Data Blending
Data Quality Issues
Inconsistent Data
Inconsistent data poses a significant challenge in data blending. Different data sources often use varying formats and standards. This inconsistency can lead to errors during the blending process. Analysts must standardize data formats to ensure accuracy.
Missing Data
Missing data can hinder the effectiveness of data blending. Incomplete datasets can result in inaccurate analyses. Analysts need to identify and address missing data before blending. Techniques such as imputation or data interpolation can fill gaps.
Technical Challenges
Compatibility Issues
Compatibility issues arise when integrating data from diverse sources. Different systems may use incompatible formats or structures. These issues can complicate the data blending process. Analysts must ensure that all data sources are compatible.
Scalability Concerns
Scalability concerns emerge as data volumes grow. Large datasets can strain existing infrastructure. This can slow down the data blending process. Organizations need scalable solutions to handle increasing data loads.
Organizational Challenges
Data Governance
Data governance is crucial for successful data blending. Organizations must establish clear policies and procedures. Proper governance ensures data quality and security. Analysts must adhere to these guidelines during the blending process.
Collaboration Between Teams
Collaboration between teams can be challenging in data blending projects. Different departments may have varying priorities and workflows. Effective communication and coordination are essential. Organizations should foster a collaborative environment.
Conclusion
Data blending plays a crucial role in modern data analysis. Combining data from various sources provides a holistic view, enhancing insights and decision-making. Organizations can leverage data blending to improve operational efficiency and gain actionable insights. Implementing data blending across different fields can unlock new opportunities for comprehensive analysis. The future of data blending looks promising, with advancements in tools and techniques continuing to drive innovation. Embracing data blending will empower organizations to fully utilize their data assets and make informed decisions.