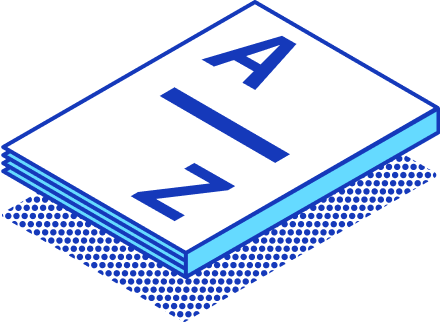
Data Lakehouse
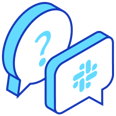
Seeking Data Lakehouse Solutions?
Connect on SlackWhat is a Data Lakehouse?
A data lakehouse blends the expansive storage of a data lake with the structured processing power of a data warehouse. This hybrid system, especially in its open form, is designed to accommodate large volumes of varied data types, making it an ideal solution for comprehensive data analytics.
The Growing Popularity of Data Lakehouses
A data lakehouse is a modern data management architecture that combines the vast storage capabilities of a data lake with the structured, performance-enhanced environment of a data warehouse. It's designed to store, manage, and analyze vast amounts of structured, semi-structured, and unstructured data, making it an ideal solution for big data analytics, AI, and machine learning.
Data Lakehouse Architecture Explained
Data lakehouse architecture integrates several key components:
-
Open Data Formats: Supports a variety of data types, including JSON, Parquet, and Avro, facilitating the storage and processing of both structured and unstructured data.
-
Metadata Management: Implements a shared metadata layer, often utilizing formats like the Iceberg table format, to organize and govern data efficiently.
-
Diverse Query Engines: Incorporates multiple engines, like enhanced versions of Presto and Spark, to cater to various analytics and AI use cases.
-
Governance and Security: Features robust built-in mechanisms for data security, privacy, and compliance, ensuring data integrity and trustworthiness.
Advantages and Challenges of Data Lakehouses
Benefits
-
- Cost Efficiency: By leveraging low-cost object storage, the lakehouse model significantly improves storage efficiency and cost-effectiveness. It simplifies data management infrastructure, reducing the need for and expense of operating multiple storage systems.
- Supports ACID Transactions: The architecture ensures data consistency across simultaneous read/write operations with robust support for ACID transactions. This capability is vital for maintaining data integrity in environments with concurrent data pipeline activities.
- Advanced Schema Management: Lakehouse facilitates the development and governance of data schemas, such as star and snowflake, ensuring data integrity and compliance through strong governance and audit mechanisms. This support extends to both implementation and evolution stages.
- Open and Standardized Formats: Utilizing open storage formats like Parquet, the architecture promotes interoperability, allowing a wide array of tools and engines—including those for machine learning and data analysis in Python/R—to access data efficiently and directly.
- Decouples Storage and Compute: Separating storage and computational processes enables scalable solutions that accommodate growing data volumes and user numbers without sacrificing performance. This decoupling is essential for modern data warehouses looking to support large-scale data operations.
- Broad Workload Compatibility: The lakehouse model is designed to support a diverse range of data processing tasks, from data science and machine learning to SQL queries and analytics. This versatility ensures that various tools can operate on the same data repository, streamlining workflow processes.
- Real-Time Data Streaming and Analytics: With built-in support for end-to-end stream computing, the architecture facilitates real-time data reporting and analytics. This feature eliminates the need for additional systems specifically dedicated to real-time processing, simplifying the data analytics infrastructure.
Challenges
-
Integration Complexities: Incorporating a data lakehouse into existing data ecosystems can present challenges, requiring careful planning and execution.
-
Data Quality Management: Consistent monitoring and management are essential to maintain high data quality and avoid the risk of data becoming stale or irrelevant.
-
Demand for Specialized Expertise: Effective utilization of a data lakehouse architecture requires specialized knowledge and skills.
Data Lakehouse vs. Data Lake vs. Data Warehouse
Data Lakehouse
- Data Handling: Capable of managing structured, semi-structured, and unstructured data. It combines the best of both worlds, offering a versatile environment for all types of data.
- Purpose: Suitable for both data analysis and ML workloads. It's the versatile multi-tool in your data toolkit, ready for various tasks.
- Cost: Offers cost-effectiveness, speed, and flexibility in storage. It's like having an expandable storage space that adjusts to your needs without breaking the bank.
- ACID Compliance: Complies with ACID, ensuring consistency in data across multiple reads or writes. This makes it a reliable foundation for collaborative and complex data operations.
Data Lake
- Data Handling: Handles semi-structured and unstructured data well. Imagine a vast digital ocean where data flows in freely, in various forms.
- Purpose: Ideal for Machine Learning (ML) and Artificial Intelligence (AI) workloads. It's like a playground for data scientists to explore and innovate.
- Cost: Storage is cost-effective, quick, and flexible. It's like renting a big storage unit where you can easily add or remove items.
- ACID Compliance: Not ACID compliant. Updating and deleting data can be complex tasks, making it a bit like a wild garden that grows in every direction.
Data Warehouse
- Data Handling: Excellently manages structured data. It's like a traditional library where every book (data) is cataloged and easy to find.
- Purpose: Best suited for data analysis and Business Intelligence (BI). It's the go-to for generating reports and insights that help in decision-making.
- Cost: Storage can be expensive and time-consuming since everything needs to be neatly organized and indexed.
- ACID Compliance: Fully complies with ACID (Atomicity, Consistency, Isolation, Durability) standards, ensuring the highest level of data integrity. This means that transactions are processed reliably.
Summary
- Data Warehouses are like well-organized libraries perfect for structured data analysis and BI tasks but come with higher costs and strict data management.
- Data Lakes are vast storages for all data types, especially beneficial for ML and AI, offering flexibility and cost efficiency but lacking in transactional integrity.
- Lakehouses merge the strengths of lakes and warehouses, providing a flexible, cost-effective solution that handles all data types and supports complex analytics and ML, all while maintaining high data integrity.
Data Lakehouse in Practice: Industry Applications
Financial Services
- Risk Analysis and Fraud Detection: Financial institutions leverage data lakehouses to process vast amounts of transactional data for real-time fraud detection and risk assessment.
- Customer Insights and Personalization: Banks and financial firms use data lakehouses to analyze customer data, enabling personalized service offerings and improving customer experience.
Healthcare
- Patient Data Analysis: Healthcare providers utilize data lakehouses to store and analyze patient records, treatment histories, and clinical research data, leading to improved patient care and treatment outcomes.
- Medical Research: Data lakehouses facilitate the storage and analysis of large-scale medical research data, accelerating discoveries and advancements in medical science.
Retail and E-Commerce
- Customer Behavior Analysis: Retailers and e-commerce platforms use data lakehouses to understand customer buying patterns, optimize inventory management, and tailor marketing strategies.
- Supply Chain Optimization: Data lakehouses enable efficient analysis of supply chain data, helping businesses optimize logistics, reduce costs, and improve delivery times.
Technology and Media
- Content Personalization: Media and technology companies utilize data lakehouses for content recommendation algorithms, personalizing user experiences based on viewing or usage patterns.
- Product Development Insights: Tech companies leverage data lakehouses to gather insights from user feedback and usage data, driving product innovation and improvement.
Frequently Asked Questions (FAQs) about Data Lakehouse
-
What are the main advantages of data lakehouse architecture?
- The main advantages of data lakehouse architecture include integrating the strengths of data lakes and data warehouses, enabling organizations to store all types of data on a single platform while supporting complex queries and analytics. Additionally, this integrated architecture offers greater flexibility and efficiency, helping businesses better meet their evolving data needs.
-
What should be considered when implementing a data lakehouse architecture?
- When implementing a data lakehouse architecture, organizations should consider several factors. First, clarify data requirements and objectives to guide the design and implementation of the architecture. Second, choose suitable technologies and tools for data integration, storage, processing, querying, and governance. Finally, continuously manage and optimize the architecture to adapt to changing data needs.
-
Is data lakehouse architecture suitable for all types of businesses?
- Not all businesses need to implement a data lakehouse architecture. The necessity mainly depends on the business's data requirements and resources. For businesses with large and diverse data needs and sufficient resources for implementation, adopting a data lakehouse architecture might be a good choice.
-
What are the future development trends of data lakehouse architecture?
- The future development of data lakehouse architecture is likely to see more possibilities with the emergence of new technologies and tools, such as artificial intelligence and machine learning. As business requirements evolve, companies will need more flexible and efficient data processing architectures, which will drive the development of data lakehouse architecture. Additionally, as the architecture becomes more popular, more standards and guidelines are expected to emerge to guide implementation and management.
-
Which companies have successfully applied data lakehouse architecture?
- Several large e-commerce platforms, financial service institutions, and manufacturing companies have successfully applied data lakehouse architecture. They utilize this architecture to process and analyze various business data, improving product and service offerings, enhancing operational efficiency, and driving business innovation.
Open Data Lakehouse: A Step Further
Building upon the data lakehouse model, the open data lakehouse brings in the principles of openness, enhancing interoperability, transparency, and flexibility. This model fosters a more democratized and adaptable approach to data analytics, crucial for seamless tool and system integration.
At the latest Data & AI Summit organized by Databricks, Ali Ghodsi, CEO of Databricks, acknowledged StarRocks, an open-source real-time OLAP database from the Linux Foundation, for its successful integration into Databricks' open data lakehouse architecture. This recognition also highlights StarRocks' contribution to enhancing openness in the analytics industry.