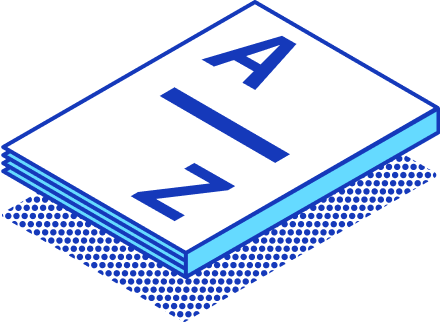
Data Lifecycle
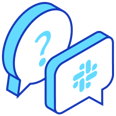
Join StarRocks Community on Slack
Connect on SlackWhat Is Data Lifecycle
The Data Lifecycle represents the journey of data from its inception to its eventual disposal. Organizations use this framework to manage data effectively. Each stage in the Data Lifecycle presents unique challenges and opportunities.
Key Components
-
Data Creation: Data originates from various sources, including user input and sensors.
-
Data Storage: Organizations store data in databases or cloud services.
-
Data Usage: Analysts and systems utilize data for decision-making.
-
Data Sharing: Data moves across departments or organizations.
-
Data Archiving: Older data moves to long-term storage.
-
Data Deletion: Data undergoes secure removal when no longer needed.
Importance in Data Management
Understanding the Data Lifecycle helps organizations optimize data management strategies. Effective management ensures data integrity and confidentiality. Organizations face increasing data volumes. Proper Data Lifecycle management becomes essential for maintaining efficiency and compliance.
Historical Context
Evolution of Data Lifecycle Concepts
The concept of the Data Lifecycle has evolved significantly over time. Early data management focused on simple storage and retrieval. Technological advancements introduced new stages and complexities. The integration of artificial intelligence transformed data handling processes. Modern practices emphasize automation and security.
Impact on Modern Data Practices
Modern data practices rely heavily on the Data Lifecycle framework. Organizations implement comprehensive approaches to manage data throughout its lifecycle. Data Lifecycle Management (DLM) involves planning and executing data migrations. Businesses use policies and software to address each phase of the Data Lifecycle. This approach maximizes data value while minimizing risks and costs.
Stages of the Data Lifecycle
Data Creation
Data creation marks the beginning of the data lifecycle. Organizations generate data from various sources. These sources include user inputs, sensors, and digital transactions. Each source contributes unique data types. The initial data collection involves gathering raw data. This stage requires careful planning. Proper collection ensures data quality and relevance.
Sources of Data
Data originates from diverse sources. User inputs provide valuable insights. Sensors capture environmental data. Digital transactions record business activities. Each source plays a crucial role. Understanding these sources aids in effective data management.
Initial Data Collection
Initial data collection involves systematic gathering. Organizations must ensure accuracy during this phase. Effective collection methods enhance data quality. High-quality data supports better decision-making. The data management team oversees this process. Proper oversight minimizes errors and inconsistencies.
Data Storage
Data storage represents a critical stage in the data lifecycle. Organizations store large data volumes securely. Storage solutions vary based on needs. Options include databases and cloud services. Each solution offers unique benefits. Security measures protect stored data. Data privacy remains a top priority.
Storage Solutions
Organizations choose storage solutions carefully. Databases provide structured storage. Cloud services offer scalability. Each option suits different requirements. Consideration of cost and accessibility is essential. The data management team evaluates these factors.
Data Security Measures
Data security measures safeguard stored data. Encryption protects sensitive information. Access controls limit unauthorized access. Regular audits ensure compliance with standards. Data privacy laws guide security practices. Adhering to these laws prevents breaches.
Data Usage
Data usage involves analyzing and applying data. Organizations leverage data for insights. Analysis techniques reveal patterns and trends. These insights drive business processes. Effective usage maximizes data value. Proper application enhances operational efficiency.
Data Analysis Techniques
Data analysis techniques uncover valuable insights. Statistical methods identify trends. Machine learning models predict outcomes. Each technique offers distinct advantages. Choosing the right method depends on goals. The data management team selects appropriate techniques.
Application in Business Processes
Data application transforms business processes. Insights inform strategic decisions. Operations become more efficient. Data-driven strategies improve outcomes. The data lifecycle stages support continuous improvement. Organizations benefit from informed decision-making.
Data Sharing
Data sharing plays a crucial role in the data lifecycle. Organizations distribute data across departments or with external partners. Effective data distribution enhances collaboration and decision-making.
Methods of Data Distribution
Organizations employ various methods to distribute data. Email remains a common method for sharing small datasets. Cloud-based platforms facilitate access to large data volumes. APIs enable seamless integration between systems. Each method offers unique advantages. The data management team evaluates these options based on needs.
Ensuring Data Integrity
Maintaining data integrity is essential during sharing. Data integrity ensures accuracy and consistency. Validation checks prevent errors during transfer. Encryption protects data from unauthorized access. Regular audits verify data accuracy. Adhering to data protection standards safeguards data privacy.
Data Archiving
Data archiving involves moving older data to long-term storage. Archiving preserves data for future reference or compliance. Proper archiving practices ensure data remains accessible and secure.
Long-term Storage Solutions
Organizations choose long-term storage solutions carefully. Tape storage provides cost-effective options for large data volumes. Cloud archiving offers scalability and accessibility. Each solution suits different requirements. Consideration of data retrieval needs is essential. The data management team assesses these factors.
Archiving Best Practices
Adopting best practices enhances data archiving. Metadata documentation aids in future retrieval. Regular audits ensure archived data remains intact. Encryption protects archived data from breaches. Compliance with data protection regulations prevents legal issues. Organizations benefit from efficient archiving processes.
Data Deletion
Data deletion marks the final stage of the data lifecycle. Secure deletion methods ensure data removal. Compliance with regulations governs data disposal practices.
Secure Deletion Methods
Organizations implement secure deletion methods to protect data. Overwriting data prevents recovery. Degaussing erases magnetic storage media. Physical destruction ensures complete data removal. Each method offers varying levels of security. The data management team selects appropriate techniques.
Compliance with Regulations
Compliance with regulations guides data deletion. Data protection laws mandate secure disposal. GDPR and HIPAA set standards for data handling. Adhering to these regulations prevents legal consequences. Regular audits verify compliance with standards. Organizations maintain data privacy through proper deletion practices.
Importance of Data Lifecycle Management
Data lifecycle management plays a crucial role in modern organizations. Effective management of data from creation to deletion ensures optimal use and security. Organizations must understand the benefits and challenges associated with data lifecycle management.
Benefits of Effective Management
Effective data lifecycle management provides several advantages. Organizations can improve data quality and enhance decision-making processes.
Improved Data Quality
Data lifecycle management employs structured processes to maintain high data quality. Proper management reduces errors and inconsistencies. Organizations can ensure data integrity through regular audits and validation checks. High-quality data supports accurate analysis and reliable insights. Businesses benefit from improved operational efficiency and customer satisfaction.
Enhanced Decision Making
Organizations rely on data-driven decisions for strategic planning. Data lifecycle management ensures timely access to relevant information. Analysts can apply advanced techniques to uncover valuable insights. Decision-makers gain confidence in their choices with accurate data. Enhanced decision-making leads to better business outcomes and competitive advantage.
Challenges in Data Lifecycle Management
Organizations face several challenges in conducting data lifecycle management. Identifying common obstacles and implementing strategies to overcome them is essential.
Common Obstacles
Organizations encounter various obstacles in data lifecycle management. Increasing data volumes pose storage and processing challenges. Compliance with data privacy laws requires careful handling of sensitive information. Data breaches and unauthorized access threaten data security. Inadequate resources and expertise hinder effective management.
Strategies to Overcome Challenges
Organizations can adopt strategies to address challenges in data lifecycle management. Investing in scalable storage solutions accommodates growing data volumes. Implementing robust security measures protects sensitive information. Regular training enhances staff expertise in data management practices. Compliance with regulations, such as GDPR and HIPAA, ensures legal adherence. Organizations can optimize data management by following best practices.
Data Lifecycle vs. Information Lifecycle Management
Understanding the differences and similarities between Data Lifecycle Management (DLM) and ILM can help you make informed decisions about managing data and information within your organization. Both frameworks aim to optimize processes, but they focus on different aspects of data and information handling.
Key Differences
Scope and Focus
Data Lifecycle Management (DLM) focuses on the journey of data from creation to disposal. DLM addresses data quality, security, and compliance throughout its lifecycle. Organizations use DLM to ensure data integrity and maximize value. DLM involves policies and practices that govern data handling.
ILM, on the other hand, encompasses a broader scope. ILM manages not only data but also information assets. ILM considers the value of information in business contexts. The focus of ILM extends beyond data to include documents, emails, and other information types. ILM aims to align information management with business goals.
Application in Different Contexts
Organizations apply DLM in contexts where data-driven decisions are crucial. DLM enhances data quality and supports efficient processes. Businesses use DLM to control costs and minimize risks. DLM solutions include data backup, replication, and archiving.
ILM finds application in environments where information governance is essential. ILM aligns with IT governance and management strategies. Organizations use ILM to address business goals and drivers. ILM ensures that information remains accessible and valuable throughout its lifecycle.
Similarities
Overlapping Stages
Both DLM and ILM share overlapping stages in their frameworks. Data creation, storage, and usage are common to both. Archiving and deletion also appear in both lifecycles. These stages ensure that data and information remain useful and secure.
Shared Objectives
DLM and ILM share objectives that enhance organizational efficiency. Both aim to optimize the management of data and information. Ensuring data integrity and confidentiality is a shared goal. Both frameworks seek to improve decision-making and operational outcomes. Organizations benefit from adopting either framework to achieve strategic objectives.
Compliance with Data Lifecycle
Regulatory Requirements
Understanding regulatory requirements is crucial for effective DLM. Organizations must adhere to specific regulations to ensure data security and integrity. Compliance with data lifecycle practices helps organizations avoid legal issues.
Key Regulations to Consider
Several key regulations impact data management practices. The General Data Protection Regulation (GDPR) governs data protection in the European Union. The Health Insurance Portability and Accountability Act (HIPAA) regulates healthcare data in the United States. These regulations set standards for data handling and protection.
Impact on Data Management Practices
Regulations influence how organizations manage data. Compliance requires implementing robust security measures. Organizations must conduct regular audits to verify adherence. Failure to comply can result in significant penalties. DLM ensures data security by aligning practices with regulatory standards.
Ensuring Compliance
Ensuring compliance involves adopting best practices and utilizing appropriate tools. Organizations must focus on maintaining data integrity and confidentiality throughout its lifecycle.
Best Practices
Implementing best practices enhances data compliance management. Regular training keeps staff informed about regulatory changes. Organizations should establish clear data handling policies. Regular audits help identify potential compliance gaps. Encryption protects sensitive data during storage and transfer.
Tools and Technologies
Various tools and technologies support compliance with data lifecycle requirements. Data management software automates compliance checks. Encryption tools safeguard data from unauthorized access. Audit tools monitor data access and usage. These technologies streamline compliance efforts and enhance data security.
Conclusion
Understanding the data lifecycle is crucial for any organization. Effective management of data from creation to deletion ensures optimal use and security. Organizations can enhance operational efficiency and maintain compliance with regulations through augmented data lifecycle management. This approach enables businesses to handle data efficiently and securely. The future of data lifecycle management promises further integration of advanced technologies. These advancements will continue to optimize processes and ensure data integrity. Organizations must stay informed and adapt to these changes to remain competitive.