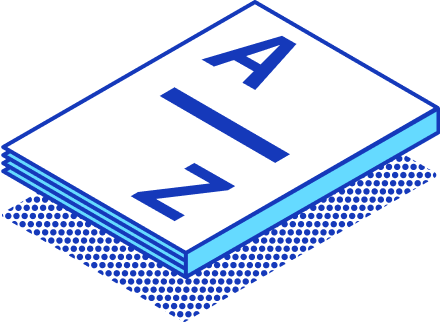
Data Volume
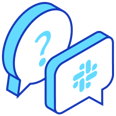
Join StarRocks Community on Slack
Connect on SlackWhat Is Data Volume?
Definition of Data Volume
The definition of data volume refers to the vast quantity of data generated and processed by organizations. This concept encompasses the size and amount of data that businesses must manage. The definitions of data volume highlight its role in big data, where the volume is a critical factor. For example, all credit card transactions in Europe on a single day represent a high-volume data set. The increasing volume of data requires specialized processing technologies, as traditional methods cannot handle such large quantities.
Measuring Data Volume
Units of Measurement for Data Volume
Data volume is measured using specific units that indicate the size of data sets. Common units include bytes, terabytes, and petabytes. These measurements help quantify the amount of collected data stored in databases. As the global datasphere grows, reaching an estimated 175 zettabytes by 2025, understanding these units becomes essential. Organizations must track the quantity of data to ensure efficient storage and processing.
Tools and Techniques for Measuring Data Volume
Various tools and techniques assist in measuring data volume. Software solutions provide insights into the quantity of data stored and processed. These tools help businesses monitor their service volume and optimize data management strategies. Applicable ESS documentation offers guidelines for using these tools effectively. Companies rely on these resources to handle the growing volume of data efficiently. Regular analysis of collected data ensures that organizations can adapt to changing demands.
The Importance of Data Volume
Impact on Data Storage
Challenges in storing large volumes of data
Organizations face significant challenges when storing large volumes of data. The exponential growth of data volume requires scalable storage solutions. Traditional storage methods cannot handle the massive scale of collected data. Businesses must address the legal requirements and operational issues associated with data storage. The increasing volume of data demands efficient management strategies. Companies need to consider the cost implications of storing vast amounts of data.
Solutions for efficient data storage
Efficient data storage solutions are essential for managing large data volumes. Businesses can utilize data lakes to store unstructured and structured data at a low cost. Data lakes offer scalability, accommodating the growing volume of collected data. Organizations can implement data volume management strategies to optimize storage resources. These strategies help reduce the time and resources needed for data migration. Companies can choose from block, file, and object storage types for scalable solutions.
Influence on Data Processing
Processing large data sets
Processing large data sets presents unique challenges for businesses. The sheer volume of data requires specialized processing technologies. Big data sets often exceed terabytes and petabytes, necessitating advanced solutions. Companies must ensure that their software can handle the complexity of large data volumes. Efficient data processing is crucial for extracting valuable insights from collected data. Businesses need to adapt their systems to manage the increasing data volume effectively.
Technologies for handling big data
Technologies for handling big data play a vital role in managing data volume. Scalable and efficient software solutions are necessary for processing large data sets. Companies can leverage big data technologies to analyze and interpret collected data. These technologies enable businesses to gain insights from vast amounts of information. Organizations must invest in software that supports the processing of high data volumes. Advanced analytics tools help companies make data-driven decisions based on customer insights.
Data Volume in Business Applications
Role in Business Intelligence
Data volume plays a crucial role in business intelligence. The vast amount of data collected daily enables organizations to gain insights into customer behavior and market trends. Businesses analyze big data to identify patterns and make informed decisions. High data volume allows companies to perform comprehensive analytics, leading to improved strategies and outcomes.
Data volume's effect on analytics
The impact of data volume on analytics is significant. Large datasets provide a more accurate representation of customer usage data and market conditions. Organizations utilize advanced software to process big data efficiently. This processing leads to actionable insights that drive business growth. Companies with access to extensive data volume can better understand customer needs and preferences.
Data Volume and Decision Making
Data volume significantly influences decision-making processes. Organizations rely on big data to inform strategic decisions. The ability to analyze large datasets provides a competitive advantage. Companies that leverage data volume effectively can anticipate market changes and adapt accordingly.
How data volume informs strategic decisions
Data volume informs strategic decisions by providing a comprehensive view of the business environment. Companies use data volume to evaluate market opportunities and risks. The insights gained from big data enable organizations to develop effective strategies. Businesses can align their goals with customer needs and market demands.
Examples of data-driven decision making
Examples of data-driven decision-making demonstrate the value of data volume. A manufacturing company used data volume to optimize production processes. The analysis of raw data identified inefficiencies and led to cost savings. An advertising agency utilized data volume to enhance campaign effectiveness. The insights gained from customer data resulted in higher engagement rates.
Challenges Associated with Data Volume
Data Management Issues
Data management presents significant challenges due to the sheer volume of information generated daily. Businesses collect vast amounts of data, often exceeding terabytes and petabytes. The exponential growth of data requires scalable and efficient big data technologies. Traditional storage methods cannot handle such large volumes effectively. Organizations must adopt new strategies to manage this data efficiently.
Data Quality and Integrity Concerns
Ensuring data quality and integrity becomes increasingly difficult as data volume grows. Large datasets can contain errors or inconsistencies that affect analysis outcomes. Maintaining high-quality data is crucial for accurate decision-making. Organizations must implement rigorous data validation processes to ensure integrity. Regular audits help identify and rectify issues within datasets.
Strategies for Effective Data Management
Effective data management strategies are essential for handling large volumes. Businesses can utilize big data technologies to optimize storage and processing. Implementing data governance frameworks ensures consistency and reliability. Organizations should invest in advanced software solutions for data management. These tools help streamline operations and improve data accessibility.
Security and Privacy Concerns
Security and privacy concerns arise when handling large data volumes. The vast amount of information collected increases the risk of breaches. Protecting sensitive data requires robust security measures. Organizations must prioritize data security to prevent unauthorized access.
Risks of Handling Large Data Volumes
Handling large data volumes poses significant risks to organizations. Data breaches can result in financial losses and reputational damage. The complexity of big data environments increases vulnerability to cyberattacks. Businesses must remain vigilant to protect their data assets. Implementing comprehensive security protocols mitigates these risks.
Best Practices for Data Security
Adopting best practices for data security is vital for safeguarding information. Organizations should implement encryption to protect sensitive data. Regular security audits help identify potential vulnerabilities. Training employees on data security protocols enhances awareness. Investing in advanced security software provides additional protection. Businesses must stay updated on emerging threats to maintain security.
Future Trends in Data Volume
Growth of Data Volume
Predictions for data volume increase
The growth of data volume continues to accelerate. Experts predict that the global datasphere will reach unprecedented levels. The increase in data volume results from the proliferation of digital devices and the expansion of the internet. Organizations collect vast amounts of data from various sources. The rise of the Internet of Things (IoT) contributes significantly to this trend. IoT devices generate continuous streams of data. The demand for real-time analytics drives further data collection. Businesses must prepare for the challenges associated with managing large data volumes.
Implications for businesses and technology
The implications of increasing data volume are profound. Businesses face new challenges in storing and processing big data. Traditional storage solutions may not suffice. Companies need scalable and efficient systems. Advanced software plays a crucial role in handling large datasets. The ability to process big data quickly becomes a competitive advantage. Organizations must invest in robust infrastructure. Data management strategies require adaptation to accommodate growth. The integration of artificial intelligence enhances data processing capabilities. AI-driven analytics provide deeper insights into customer behavior. Businesses can make informed decisions based on comprehensive data analysis.
Innovations in Managing Data Volume
Emerging technologies for data management
Emerging technologies revolutionize data management. Cloud computing offers scalable storage solutions. Companies can store vast amounts of data without physical limitations. Blockchain technology ensures data integrity and security. Decentralized networks provide transparency and traceability. Machine learning algorithms enhance data processing efficiency. These algorithms identify patterns within large datasets. Businesses gain valuable insights through predictive analytics. Edge computing reduces latency in data processing. Data processing occurs closer to the source, improving response times. These innovations transform how organizations manage big data.
Future solutions for data volume challenges
Future solutions address the challenges of data volume. Data-as-a-Service (DaaS) models gain popularity. DaaS provides flexible access to data resources. Organizations can scale data usage according to needs. Data storytelling emerges as a powerful tool. Companies combine data, narrative, and visuals. This approach communicates insights effectively. Decision-makers understand complex data through compelling stories. Data visualization tools enhance comprehension. Interactive dashboards present data in user-friendly formats. Businesses leverage these tools to drive positive change. The future of data volume management holds great promise.
Conclusion
Understanding data volume is crucial for businesses navigating the digital landscape. Efficient management of big data ensures organizations can leverage insights for strategic advantage. Companies must adopt scalable technologies to handle the increasing volume of big data. Implementing effective data management strategies reduces redundancy and optimizes processing. Legal requirements also drive the need for robust data volume management. Businesses must stay informed about emerging trends and technologies in big data. AI advancements will play a pivotal role in transforming big data into actionable insights. Organizations should embrace these innovations to maintain competitiveness.