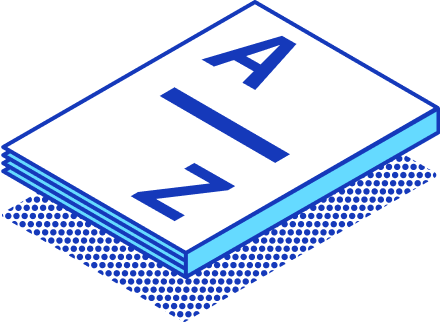
Descriptive Analytics
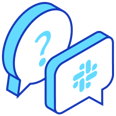
Join StarRocks Community on Slack
Connect on SlackWhat Is Descriptive Analytics
Definition and Explanation
Descriptive Analytics involves the interpretation of historical data to identify patterns and trends. This form of analytics answers the question, "What happened?" by examining past events. Businesses use Descriptive Analytics to gain insights into their operations and customer behaviors. The analysis provides a clear picture of past performance, helping organizations make informed decisions.
Key Characteristics
Descriptive Analytics focuses on summarizing large volumes of data. The process involves transforming raw data into meaningful insights. Key characteristics include the use of statistical techniques and data visualization tools. These methods help in identifying trends, patterns, and anomalies. ThoughtSpot is a powerful tool that assists businesses in conducting comprehensive Descriptive Analytics. ThoughtSpot enables users to explore data through intuitive search-driven analytics.
Historical Context and Evolution
The evolution of Descriptive Analytics can be traced back to early statistical methods. Businesses have long relied on data to understand market trends. Over time, advancements in technology have enhanced the capabilities of Descriptive Analytics. Modern tools like ThoughtSpot provide real-time insights and interactive dashboards. These innovations have made data analysis more accessible and efficient for businesses.
The Process of Descriptive Analytics
Data Gathering
Sources of Data
Businesses collect data from various sources. Customer transactions provide valuable insights into purchasing behaviors. Social media interactions reveal customer preferences and trends. Internal systems track operational performance. Each source contributes to a comprehensive understanding of business activities.
Data Cleaning and Preparation
Data cleaning ensures accuracy and consistency. Businesses remove duplicates and correct errors. Organizing data into a usable format is essential. This preparation sets the stage for effective analysis. Clean data leads to more reliable insights.
Data Analysis
Techniques and Methods
Descriptive analysis employs various techniques. Statistical methods identify patterns and trends. Data visualization tools create clear representations of findings. These techniques transform raw data into actionable insights. Businesses use these insights to guide strategic decisions.
Interpretation of Results
Interpreting results involves understanding the implications of data. Businesses look for trends that indicate opportunities or challenges. Patterns in customer behavior inform marketing strategies. Operational data highlights areas for improvement. Effective interpretation drives informed decision-making.
Case Studies:
-
Adobe's Use of Advanced Analytics: Adobe maximized ROI in marketing campaigns. The company enhanced customer satisfaction by analyzing customer journey patterns. This strategic use of analytics sustained competitive benefits.
-
Utilization of Descriptive Analytics in Marketing Campaigns: Businesses uncover information on new leads and customer preferences. Descriptive analytics guides overall business decisions. Performance, targets, and trends shape marketing strategies.
-
Descriptive Analysis in Business Operations: Reviewing historical data helps businesses ask the right questions. This process supports predictive analysis and forecasting future events. Descriptive analysis is crucial for making data-driven decisions.
Benefits of Descriptive Analytics
Improved Decision-Making
Descriptive Analytics enhances decision-making by providing a clear view of past events. Businesses can identify trends and patterns that reveal valuable insights. These insights guide strategic planning and improve outcomes.
Identifying Trends and Patterns
Businesses use Descriptive Analytics to uncover trends in customer behavior. Analyzing historical data helps in recognizing recurring patterns. This understanding allows companies to anticipate market demands and adjust strategies accordingly.
Enhancing Business Strategies
Descriptive Analytics informs strategic decisions by highlighting strengths and weaknesses. Companies can refine business strategies based on past performance data. This approach leads to more effective marketing campaigns and product development.
Operational Efficiency
Operational efficiency improves through the application of Descriptive Analytics. Businesses optimize resources and reduce costs by analyzing operational data. This process ensures that companies operate at peak efficiency.
Resource Optimization
Descriptive Analytics enables businesses to allocate resources effectively. Analyzing data on resource usage identifies areas for improvement. Companies can streamline operations and enhance productivity through informed decisions.
Cost Reduction
Businesses achieve cost reduction by using Descriptive Analytics to analyze financial data. Identifying inefficiencies in spending helps in cutting unnecessary expenses. This analysis supports sustainable growth and profitability.
Real-World Applications of Descriptive Analytics
Descriptive Analytics plays a crucial role in various industries by transforming raw data into actionable insights. Businesses leverage this form of analytics to enhance decision-making and operational efficiency. The following examples illustrate how different sectors utilize Descriptive Analytics effectively.
Industry-Specific Examples
Healthcare
Healthcare organizations use Descriptive Analytics to improve patient care and operational efficiency. Hospitals analyze emergency room admissions to understand peak times and patient demographics. This analysis helps in resource allocation and staff scheduling. Real-time data on admission statistics provides insights into patient flow and treatment outcomes. Healthcare providers can identify trends in patient conditions and adjust treatment protocols accordingly.
Retail
Retailers rely on Descriptive Analytics to understand consumer behavior and optimize inventory management. Analyzing sales data helps retailers identify popular products and seasonal trends. This information guides purchasing decisions and marketing strategies. Retailers also track customer preferences through loyalty programs and online interactions. Insights from this analysis enable personalized marketing campaigns and improved customer experiences.
Case Studies
Successful Implementations
Netflix's Use of Descriptive Analytics: Netflix employs Descriptive Analytics to tailor content offerings and enhance viewer engagement. The company analyzes viewing patterns and audience preferences to decide on new content creation. This data-driven approach influences marketing strategies and production partnerships. Netflix's success demonstrates the power of Descriptive Analytics in the entertainment industry.
How Descriptive Analytics Shapes Marketing Campaigns: Businesses use Descriptive Analytics to evaluate marketing campaign performance. Companies track conversion rates, customer preferences, and marketing spending. This analysis reveals successful tactics and areas for improvement. By understanding campaign strengths and weaknesses, businesses can refine their marketing strategies and maximize return on investment.
Lessons Learned
Descriptive Analytics offers valuable lessons for businesses aiming to harness data effectively. Successful implementations highlight the importance of accurate data collection and thorough analysis. Organizations must ensure data quality to derive meaningful insights. Continuous monitoring and adaptation are essential for maintaining competitive advantages. Businesses that embrace Descriptive Analytics can make informed decisions and drive growth.
Comparing Descriptive Analytics with Other Types of Analytics
Descriptive vs. Predictive Analytics
Key Differences
Descriptive Analytics focuses on understanding past events. Analysts use historical data to identify patterns and trends. This approach answers the question, "What happened?" Predictive Analytics, on the other hand, forecasts future events. Analysts use statistical models and machine learning techniques. This method predicts potential outcomes based on current and historical data. Descriptive Analytics provides a foundation for understanding past performance. Predictive Analytics builds on this foundation to anticipate future possibilities.
Use Cases
Businesses use Descriptive Analytics to analyze customer behavior. Retailers examine sales data to identify popular products. Healthcare providers study patient records to improve care delivery. Predictive Analytics finds applications in risk management. Financial institutions predict credit risks using predictive models. Marketing teams forecast customer responses to new campaigns. Both types of analytics play crucial roles in strategic planning.
Descriptive vs. Prescriptive Analytics
Key Differences
Descriptive Analytics summarizes data and performance metrics. Analysts transform raw data into meaningful insights. This process helps businesses understand past performance. Prescriptive Analytics goes a step further. This approach suggests actions to achieve desired outcomes. Analysts provide specific recommendations based on data analysis. Descriptive Analytics offers a clear view of historical data. Prescriptive Analytics guides decision-makers toward optimal solutions.
Use Cases
Businesses rely on Descriptive Analytics for performance evaluation. Companies assess financial health by analyzing historical data. Operational efficiency improves through data-driven insights. Prescriptive Analytics supports decision-making in complex scenarios. Supply chain managers optimize logistics using prescriptive models. Healthcare providers enhance treatment plans with actionable recommendations. Both analytics types contribute to informed business decisions.
Challenges and Limitations of Descriptive Analytics
Descriptive Analytics offers valuable insights into historical data, yet it faces several challenges and limitations. Understanding these challenges is crucial for businesses aiming to maximize the potential of data analysis.
Data Quality Issues
Data quality significantly impacts the effectiveness of Descriptive Analytics. Businesses often encounter challenges related to incomplete or inaccurate data.
Incomplete or Inaccurate Data
Incomplete data sets hinder comprehensive analysis. Missing information leads to gaps in understanding past events. Inaccurate data skews results, leading to misleading conclusions. Ensuring data accuracy is vital for reliable Descriptive Analytics.
Data Silos
Data silos pose another challenge. Different departments store data separately, creating barriers to comprehensive analysis. Lack of integration limits the ability to gain a holistic view of business operations. Breaking down silos enhances the effectiveness of Descriptive Analytics.
Interpretation Challenges
Interpreting results from Descriptive Analytics requires careful consideration. Misinterpretation leads to incorrect conclusions and misguided decisions.
Misleading Conclusions
Misleading conclusions arise from incorrect data interpretation. Analysts may identify patterns that do not exist. Overemphasizing certain trends leads to skewed business strategies. Accurate interpretation ensures meaningful insights from Descriptive Analytics.
Over-reliance on Historical Data
Over-reliance on historical data presents another limitation. Descriptive Analytics focuses on past events, which may not always predict future outcomes. Businesses must combine Descriptive Analytics with other analytical approaches for a comprehensive strategy. Relying solely on historical data limits the ability to adapt to changing market conditions.
Scientific Research Findings:
-
Descriptive Analytics cannot test theories or predict future events. It provides insights into past interactions and decisions.
-
Businesses should use Descriptive Analytics as a foundation for further analysis and strategic planning.
Conclusion
Descriptive analytics plays a vital role in understanding historical data. Businesses gain insights into past events and make informed decisions. This type of analysis transforms raw data into actionable insights. Companies can identify trends and optimize strategies. Descriptive methods enhance operational efficiency and drive growth. Leveraging descriptive tools empowers organizations to thrive in a data-driven world.