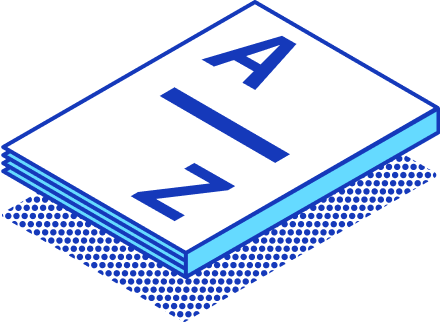
Descriptive Statistics
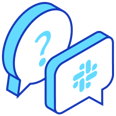
Join StarRocks Community on Slack
Connect on SlackWhat Is Descriptive Statistics
Definition and Overview
Basic Concepts
Descriptive Statistics involves methods to summarize and describe data. These methods include measures of central tendency and variability. Central tendency measures like the mean, median, and mode show average values. Variability measures like range and standard deviation reveal data spread. Descriptive Statistics provides a clear picture of data characteristics.
Historical Context
The roots of Descriptive Statistics trace back to early civilizations. Ancient societies used basic statistical methods for census and trade. The 17th century saw the formal development of statistical concepts. Scholars began to refine techniques for summarizing data. Modern Descriptive Statistics evolved from these foundational ideas.
Importance in Data Analysis
Role in Summarizing Data
Descriptive Statistics plays a crucial role in data analysis. These methods help condense large data sets into understandable formats. Analysts use summary statistics to identify patterns and trends. This process aids in making informed decisions based on data insights.
Applications in Various Fields
Descriptive Statistics finds applications across diverse fields. In education, teachers analyze student performance using averages. Businesses assess sales data to understand market trends. Healthcare professionals evaluate patient data for treatment outcomes. Descriptive Statistics provides valuable insights in every sector.
Measures of Central Tendency in Descriptive Statistics
Mean
Calculation and Interpretation
The mean represents the average of a data set. To calculate the mean, add all the numbers together. Then, divide the total by the number of values. The mean provides a central value for the data. This helps to understand the overall trend. Analysts often use the mean to compare different data sets.
Examples in Real-World Data
In real-world scenarios, the mean offers valuable insights. For example, businesses calculate the mean sales figures. This helps to determine average performance over time. Schools use the mean to assess average student scores. This assists in identifying areas needing improvement. The mean serves as a useful tool in various fields.
Median
When to Use Median
The median is the middle value in a data set. Arrange the numbers in order first. If there is an odd number of values, the median is the center one. If even, calculate the average of the two middle numbers. Use the median when data contains outliers. Outliers can skew the mean, making the median more reliable.
Examples and Significance
The median proves significant in many situations. In real estate, the median home price reflects market trends. This avoids distortion from extremely high or low prices. Income data often uses the median to show typical earnings. This method provides a clearer picture of economic conditions. The median offers a balanced view of data.
Mode
Identifying the Mode
The mode is the most frequently occurring value in a data set. To find the mode, count how often each number appears. The number with the highest count is the mode. Some data sets may have multiple modes. Others might not have any mode at all. The mode highlights common patterns in data.
Practical Applications
The mode has practical applications in various areas. Retailers analyze the mode to identify popular products. This helps in inventory management and marketing strategies. In surveys, the mode reveals the most common responses. This assists in understanding public opinion. The mode provides insights into frequent occurrences.
Measures of Variability in Descriptive Statistics
Range
Understanding Range
The range measures the difference between the highest and lowest values in a data set. To find the range, subtract the smallest number from the largest number. The range provides a quick snapshot of data spread. A larger range indicates more variability among data points. A smaller range suggests that data points are closer together.
Limitations and Uses
The range has limitations in descriptive statistics. Outliers can greatly affect the range, making it less reliable. The range does not show how data points distribute between the extremes. Despite these drawbacks, the range remains useful for initial data analysis. It helps identify potential outliers and gives a basic sense of variability.
Variance and Standard Deviation
Calculation Methods
Variance measures the average squared deviation from the mean. To calculate variance, subtract the mean from each data point, square the result, and then average these squared differences. Standard deviation is the square root of variance. This measure returns data to the original units, making interpretation easier.
Importance in Data Analysis
Variance and standard deviation provide insight into data consistency. A low standard deviation indicates that data points cluster around the mean. A high standard deviation suggests that data points spread out over a wider range. These measures help analysts understand data reliability and predictability. Variance and standard deviation are crucial for comparing different data sets.
Interquartile Range
Explanation and Calculation
The interquartile range (IQR) measures the spread of the middle 50% of data. To calculate the IQR, find the first quartile (Q1) and third quartile (Q3). Subtract Q1 from Q3 to get the IQR. This measure excludes outliers and focuses on the central portion of the data set.
Use Cases
The IQR proves valuable in identifying data distribution patterns. Analysts use the IQR to detect outliers and assess data symmetry. In fields like finance, the IQR helps evaluate investment risks by examining return variability. The IQR offers a robust measure of variability, especially when dealing with skewed data.
Frequency Distribution in Descriptive Statistics
Definition and Purpose
Frequency distribution organizes data to show how often each value occurs. This method helps identify patterns and relationships within a dataset. Analysts use frequency distribution to summarize large amounts of information.
Types of Frequency Distributions
Different types of frequency distributions exist. Discrete frequency distribution deals with distinct values. Continuous frequency distribution involves ranges of values. Each type serves specific data analysis needs.
Examples in Data Sets
Frequency distributions appear in various data sets. A survey might show how many people prefer different brands. A classroom test could reveal the number of students achieving certain grades. These examples illustrate the practical use of frequency distributions.
Histograms and Frequency Polygons
Histograms and frequency polygons visualize frequency distributions. These tools help you understand data more clearly.
Creating and Interpreting
Creating a histogram involves plotting data on a bar graph. Each bar represents the frequency of a value or range. Frequency polygons connect midpoints of histogram bars with lines. These visuals make data interpretation straightforward.
Benefits in Data Visualization
Histograms and frequency polygons offer several benefits. These tools highlight trends and outliers effectively. Visuals provide a clear picture of data distribution. Analysts use these methods to communicate findings efficiently.
Bivariate Analysis in Descriptive Statistics
Understanding Relationships
Correlation vs. Causation
Bivariate analysis explores relationships between two variables. Correlation measures how two variables move together. A positive correlation means both increase or decrease together. A negative correlation means one increases while the other decreases. Correlation does not imply causation. Two variables may correlate without one causing the other. Understanding this distinction prevents incorrect conclusions.
Examples of Bivariate Analysis
Bivariate analysis appears in many fields. In education, researchers study the relationship between study time and grades. Businesses examine the link between advertising spend and sales. Healthcare professionals analyze connections between exercise and health outcomes. These examples show how bivariate analysis provides insights into variable relationships.
Scatter Plots
How to Create and Analyze
Scatter plots visualize relationships between two variables. Each point represents a pair of values. The x-axis shows one variable, and the y-axis shows the other. To create a scatter plot, plot each pair of values on a graph. Look for patterns or trends in the plotted points. A line of best fit can help identify correlations.
Insights from Scatter Plots
Scatter plots offer valuable insights. Patterns in the data reveal relationships between variables. Clusters of points may indicate strong correlations. Outliers stand out and may require further investigation. Analysts use scatter plots to communicate findings clearly. Scatter plots provide a visual way to understand complex data relationships.
Limitations and Broader Context of Descriptive Statistics
Limitations of Descriptive Statistics
Lack of Predictive Power
Descriptive statistics provide a snapshot of data. These methods summarize and describe data characteristics. However, descriptive statistics do not predict future outcomes. Analysts cannot use descriptive statistics to forecast trends. Predictive analysis requires inferential statistics. Descriptive statistics focus on past and present data. This limitation restricts their use in future planning.
Potential Misinterpretations
Misinterpretations can occur with descriptive statistics. Data summaries may lead to incorrect conclusions. Analysts must interpret results carefully. Averages can hide important details. Outliers can skew data interpretations. Clear communication of statistical findings is crucial. Graphs and tables should accompany numerical reports. Visual aids help readers understand data nuances.
Descriptive vs. Inferential Statistics
Key Differences
Descriptive and inferential statistics serve different purposes. Descriptive statistics summarize existing data. Inferential statistics make predictions about larger populations. Descriptive statistics report facts from collected data. Inferential statistics use samples to draw conclusions. Each type of statistics has unique applications. Understanding these differences aids in data analysis.
Complementary Roles in Data Analysis
Descriptive and inferential statistics complement each other. Descriptive statistics provide a foundation for analysis. Inferential statistics build on this foundation. Analysts use descriptive statistics to understand data sets. Inferential statistics extend findings to broader contexts. Together, these methods enhance research insights. Comprehensive data analysis relies on both approaches.
Conclusion
Descriptive statistics play a vital role in data analysis. These methods provide tools to summarize and interpret complex data efficiently. Descriptive statistics serve as a foundation for understanding data characteristics. Analysts use these methods to identify patterns and trends. Descriptive statistics help make informed decisions based on research findings. Explore further statistical methods to enhance data analysis skills.