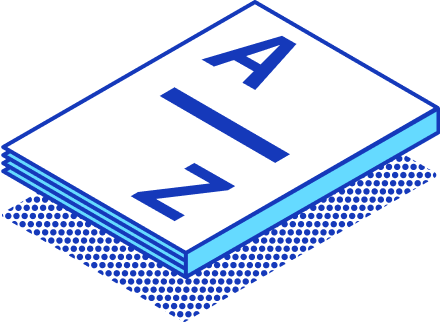
Understanding Text Analytics
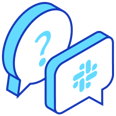
Join StarRocks Community on Slack
Connect on SlackWhat Is Text Analytics
Definition and Explanation
Text analytics refers to the process of converting unstructured text into structured data. This transformation allows businesses to derive meaningful insights from vast amounts of text. Text analysis software plays a crucial role in this process by using advanced algorithms to interpret and categorize text. The software identifies patterns, trends, and relationships within the text, making it easier for organizations to make informed decisions.
Key Components of Text Analytics
Text analytics comprises several key components that work together to analyze text effectively:
-
Natural Language Processing (NLP): NLP enables machines to understand and interpret human language. It breaks down text into smaller parts, such as words and phrases, to analyze their meaning and context.
-
Machine Learning: Machine learning algorithms help in identifying patterns and trends within text data. These algorithms learn from existing data to improve their accuracy over time.
-
Statistical Analysis: Statistical methods are used to quantify the relationships between different elements within the text. This helps in identifying significant patterns and trends.
-
Linguistic Techniques: Linguistic techniques focus on understanding the structure and meaning of language. They help in identifying the syntax and semantics of text.
Historical Background
The history of text analytics dates back to the early days of computing. Initially, researchers focused on developing algorithms to process and analyze text data. Over time, advancements in technology led to the development of sophisticated text analysis software. Today, text analytics is an integral part of many industries, helping organizations make sense of large volumes of text data.
Importance of Text Analytics
Role in Data Analysis
Text analytics plays a vital role in data analysis by providing insights that are not easily accessible through traditional methods. It allows businesses to analyze customer feedback, social media posts, and other text data sources. By doing so, organizations can identify trends and patterns that inform their strategies and decision-making processes.
Impact on Business Decision-Making
The impact of text analytics on business decision-making is profound. By analyzing text data, companies can gain a deeper understanding of customer sentiments and preferences. This information helps businesses tailor their products and services to meet customer needs. Additionally, text analytics enables organizations to respond quickly to market changes, giving them a competitive edge.
Techniques in Text Analytics
Text analytics employs various techniques to transform unstructured text into meaningful insights. These methods enable businesses to understand and utilize text data effectively.
Natural Language Processing (NLP)
NLP is a crucial component of text analytics. It allows machines to interpret human language, making it possible to analyze large volumes of text data.
Sentiment Analysis
Sentiment analysis identifies emotions within text. It helps businesses understand customer opinions by categorizing text as positive, negative, or neutral. This technique enables companies to respond to customer feedback promptly.
Named Entity Recognition
Named Entity Recognition (NER) locates and classifies key elements in text. It identifies names, dates, and locations, providing valuable context. NER enhances the ability to extract specific information from text data.
Machine Learning Approaches
Machine learning plays a vital role in text analytics. It uses algorithms to detect patterns and trends in text data, improving accuracy over time.
Supervised Learning
Supervised learning involves training models with labeled data. These models learn to predict outcomes based on input text. Businesses use this approach to classify text data efficiently.
Unsupervised Learning
Unsupervised learning analyzes text without predefined labels. It discovers hidden patterns and structures within text data. This method helps in clustering similar text documents, revealing insights that might not be immediately apparent.
Text analytics continues to evolve, offering powerful tools for understanding complex text data. By leveraging these techniques, organizations can gain a competitive edge in today's data-driven world.
Applications of Text Analytics
Text analytics plays a crucial role in various industries. It transforms unstructured text into actionable insights, enhancing decision-making processes.
Business Intelligence
Businesses leverage text analytics to gain a competitive edge. They extract valuable information from customer interactions and market trends.
Customer Feedback Analysis
Companies use text analysis to understand customer sentiments. They analyze reviews, emails, and social media posts. This process helps identify areas for improvement and enhances customer satisfaction. By integrating text analytics into their data analytics project, businesses can tailor their services to meet customer needs.
Market Research
Text analytics aids in market research by uncovering trends and patterns. Businesses analyze competitor strategies and consumer preferences. This insight supports strategic planning and innovation. Big data analytics tools enable companies to process vast amounts of text data efficiently.
Healthcare
In healthcare, text analytics offers transformative benefits. It improves patient care and operational efficiency.
Patient Data Analysis
Healthcare providers use text analytics to analyze patient records and feedback. This analysis identifies health trends and improves treatment plans. By incorporating text analytics into their data analytics project plan, healthcare organizations enhance patient outcomes.
Predictive Analytics
Predictive analytics in healthcare relies on text data to forecast patient needs. It helps in early diagnosis and personalized treatment. Text analytics software processes large volumes of data, providing valuable insights for healthcare professionals.
Text analytics continues to evolve, offering innovative solutions across industries. By integrating text analytics into data analytics projects, organizations can harness the power of big data. This approach leads to improved decision-making and strategic growth.
Comparing Text Analytics with Related Concepts
Text analytics often gets confused with related concepts like text mining and data analytics. Understanding the differences and similarities among these concepts helps in choosing the right approach for specific needs.
Text Mining vs. Text Analytics
Differences in Techniques
Text mining and text analytics share similarities but differ in techniques. Text mining focuses on extracting information from large volumes of text. It uses methods like natural language processing and machine learning to identify patterns. Text analytics, on the other hand, involves analyzing text to derive insights. It uses statistical and linguistic techniques to interpret text data. While text mining emphasizes information retrieval, text analytics aims at understanding and interpreting the text.
Use Cases
Text mining and text analytics serve different purposes. Text mining is useful in scenarios where the goal is to extract specific information. For example, businesses use text mining to gather customer feedback from social media. Text analytics, however, is more suitable for understanding customer sentiments. Companies employ text analytics tools to analyze reviews and improve customer satisfaction. Both approaches play crucial roles in handling text data, but their applications vary based on objectives.
Data Analytics vs. Text Analytics
Scope and Focus
Data analytics encompasses a broader scope than text analytics. It involves analyzing various types of data, including numerical and categorical data. Text analytics, however, focuses specifically on text data. While data analytics uses traditional statistical methods, text analytics employs natural language processing and machine learning. The focus of data analytics lies in understanding overall data trends. Text analytics, in contrast, aims at interpreting human language and extracting insights from text.
Integration of Techniques
Integrating text analytics with data analytics enhances decision-making. Businesses combine these techniques to gain comprehensive insights. For instance, they analyze customer feedback using text analytics and correlate it with sales data. This integration provides a holistic view of customer behavior. Text analytics tools facilitate this process by offering advanced features for analyzing text data. By integrating these techniques, organizations can make informed decisions and drive growth.
Understanding the distinctions between text mining, text analytics, and data analytics is essential. Each approach offers unique benefits and serves different purposes. By leveraging the right techniques, businesses can unlock the full potential of their data.
Future Implications of Text Analytics
Text analytics continues to evolve, offering new possibilities and challenges. Understanding these future implications helps businesses stay ahead in a competitive landscape.
Emerging Trends
AI Integration
Artificial Intelligence (AI) plays a crucial role in the future of text analytics. AI enhances the ability to process and interpret text data. It enables more accurate sentiment analysis and pattern recognition. Businesses leverage AI to automate complex tasks, improving efficiency and accuracy. AI integration allows for more personalized customer interactions, enhancing user experiences.
Real-Time Analytics
Real-time analytics transforms how organizations respond to data. It enables immediate insights from text data, allowing quick decision-making. Companies monitor social media and customer feedback in real time. This capability helps them address issues promptly and adapt strategies. Real-time analytics provides a competitive edge by keeping businesses informed and responsive.
Challenges and Opportunities
Data Privacy Concerns
Data privacy remains a significant concern in the future of text analytics. Organizations must handle sensitive information responsibly. They need to comply with regulations and protect customer data. Ensuring privacy builds trust and maintains ethical standards. Companies must invest in secure systems and transparent practices to address these concerns.
Advancements in Technology
Technological advancements drive the future of text analytics. New tools and techniques enhance data processing capabilities. Machine learning models become more sophisticated, offering deeper insights. These advancements open opportunities for innovation and growth. Businesses can explore new applications and improve existing processes. Staying updated with technology ensures they harness the full potential of text analytics.
The future of text analytics promises exciting developments. By embracing emerging trends and addressing challenges, organizations can unlock new opportunities. This approach leads to improved decision-making and strategic success.
Conclusion
Text analytics transforms unstructured data into actionable insights. It empowers businesses to make informed decisions by analyzing text data. Key techniques include natural language processing and machine learning. These methods uncover patterns and trends, enhancing business strategies. The future of text analytics promises exciting developments. AI integration and real-time analytics will revolutionize data processing. Organizations must address data privacy concerns to maintain trust. By embracing advancements, businesses can unlock new opportunities and drive growth. Text analytics remains a vital tool in the data-driven world, offering endless possibilities for innovation.