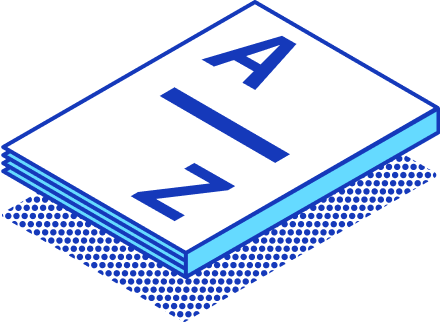
Exploring the Next Wave of Cognitive Analytics
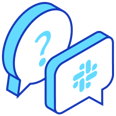
Join StarRocks Community on Slack
Connect on SlackUnderstanding Cognitive Analytics
Cognitive analytics is fundamentally reshaping the way we approach data-driven decision-making. Unlike traditional analytics, which primarily focuses on structured datasets and predefined models, cognitive analytics leverages artificial intelligence (AI), machine learning (ML), and natural language processing (NLP) to interpret both structured and unstructured data. This enables it to detect patterns, predict trends, and automate complex decision-making processes.
With the explosion of big data, organizations now face the challenge of extracting actionable insights from vast amounts of diverse information—ranging from transactional records and sensor data to social media posts and audio recordings. Cognitive analytics bridges this gap by mimicking human thought processes while operating at the speed and scale required for modern enterprises.
Core Technologies Driving Cognitive Analytics
Artificial Intelligence (AI) and Machine Learning (ML)
AI enables cognitive analytics systems to learn from data, improve over time, and make automated decisions. Machine learning models identify patterns in massive datasets, helping organizations forecast trends, detect anomalies, and make strategic recommendations.
-
Supervised Learning: Used in applications such as fraud detection, where labeled data helps the model recognize suspicious transactions.
-
Unsupervised Learning: Commonly applied in customer segmentation, where the system groups similar behavior patterns without predefined labels.
-
Deep Learning: Enhances image recognition, speech processing, and automated decision-making by using neural networks.
Real-World Example: AI in Healthcare
IBM Watson for Oncology is a prime example of AI-driven cognitive analytics in action. It processes vast volumes of medical literature, patient records, and clinical trials to recommend personalized treatment plans. By leveraging NLP and ML, Watson helps oncologists make data-driven decisions in cancer treatment, improving diagnosis accuracy and treatment effectiveness.
Natural Language Processing (NLP)
NLP allows cognitive analytics to interpret human language—whether from emails, customer reviews, chatbots, or call center transcripts.
-
Sentiment Analysis: Determines customer satisfaction levels based on reviews and social media posts.
-
Text Summarization: Condenses long reports into key takeaways.
-
Named Entity Recognition (NER): Extracts relevant entities, such as company names or product mentions, from documents.
Real-World Example: NLP in Finance
JPMorgan Chase’s COiN (Contract Intelligence) platform leverages NLP to analyze and extract information from complex legal documents, reducing document review time from 360,000 hours per year to seconds. This has significantly streamlined risk management and compliance in financial operations.
Automation and Augmented Analytics
Automation plays a crucial role in cognitive analytics by reducing manual efforts in data preparation, feature engineering, and model selection. Augmented analytics combines AI-driven automation with human expertise, allowing analysts to spend more time on interpretation rather than data wrangling.
Real-World Example: Predictive Maintenance in Aviation
General Electric (GE) Aviation uses cognitive analytics to predict potential aircraft engine failures before they occur. By analyzing sensor data from jet engines in real-time, GE helps airlines avoid costly unscheduled maintenance and enhances passenger safety.
The Business Value of Cognitive Analytics
Cognitive analytics is not just a theoretical concept—it delivers tangible business value across various industries. Let’s explore some key benefits:
-
Real-Time Insights: Enables organizations to react immediately to market changes, customer behavior shifts, and operational risks.
-
Enhanced Decision-Making: By identifying patterns that would be difficult for humans to detect manually, cognitive analytics helps businesses make data-backed strategic choices.
-
Process Optimization: Automates routine tasks such as data categorization, compliance monitoring, and anomaly detection.
-
Customer Experience Personalization: Recommends personalized content and offers based on behavioral analytics.
-
Fraud Detection and Risk Mitigation: Identifies suspicious activity in financial transactions and cybersecurity logs.
Real-World Example: AI in Retail
Amazon’s recommendation engine, powered by cognitive analytics, accounts for approximately 35% of its total sales. By analyzing user behavior, past purchases, and browsing patterns, Amazon delivers highly personalized shopping experiences, increasing customer retention and revenue.
Emerging Trends in Cognitive Analytics: 2025 and Beyond
AI-Powered Solutions
Advanced Predictive Analytics
Predictive analytics has been around for years, but AI is redefining its accuracy and application. AI-driven models now process both structured and unstructured data, allowing businesses to identify hidden trends and anticipate future developments with remarkable precision.
For example, AI-enhanced predictive models in supply chain management can now forecast demand fluctuations based on weather patterns, social sentiment, and geopolitical events, not just past sales data. This helps companies optimize inventory, prevent stockouts, and reduce excess supply, leading to cost savings and operational efficiency.
In finance, AI-powered analytics helps firms predict market trends and portfolio risks in real time. Hedge funds use deep learning to analyze alternative data sources—such as satellite imagery of retail parking lots—to predict consumer spending before sales reports are even published.
Real-Time Decision-Making
With AI and machine learning advancing rapidly, real-time analytics is becoming a requirement, not a luxury. Companies can no longer afford to wait for end-of-day reports; they need instant insights to remain competitive.
Consider how dynamic pricing engines in e-commerce function. Retailers like Amazon continuously adjust prices based on customer demand, competitor pricing, and inventory levels—all in real time. Airlines and ride-sharing platforms such as Uber use similar strategies to optimize pricing dynamically based on demand fluctuations.
In fraud detection, banks and fintech companies leverage AI-driven real-time analytics to flag suspicious transactions instantly, preventing fraudulent activity before it escalates. Rather than relying on static rule-based systems, AI adapts to evolving fraud tactics, improving detection rates and reducing false positives.
Integration of Augmented and Virtual Reality
Enhanced Data Visualization
Data visualization is shifting beyond dashboards and graphs. Augmented Reality (AR) is enabling analysts to explore complex datasets in 3D space, revealing patterns that might be missed on a 2D screen.
In financial markets, traders can use AR-powered visualizations to track global economic movements, overlaying live financial data on a digital world map. This allows for intuitive pattern recognition in stock trends, economic indicators, and geopolitical risks.
Immersive Training and Simulations
In industries like healthcare and manufacturing, Virtual Reality (VR) is transforming training programs. Instead of passive learning through lectures or manuals, professionals can now train in realistic virtual environments, improving retention and hands-on skills.
For example, surgeons use VR to practice complex procedures before operating on patients, reducing errors and improving patient outcomes. In the aviation industry, pilots train using AI-powered VR flight simulators that replicate real-world flying conditions with extreme accuracy, leading to better preparedness for emergencies.
Multi-Cloud and Edge Computing
Scalable Analytics Workloads
As data volumes continue to explode, relying solely on centralized cloud storage is no longer efficient. The rise of multi-cloud and edge computing allows organizations to process data closer to the source, reducing latency and improving responsiveness.
In IoT (Internet of Things) environments, edge computing is crucial. Consider a smart manufacturing plant where thousands of sensors continuously monitor machine performance. Instead of sending all data to a distant cloud server, edge devices process data locally, identifying potential equipment failures in real time and triggering preventive maintenance—saving millions in downtime costs.
Real-Time Data Processing
Autonomous vehicles are one of the most extreme examples of real-time data processing at the edge. Self-driving cars must instantly process data from multiple sources—LiDAR, GPS, cameras, and onboard sensors—to navigate safely. Waiting for cloud-based processing would be too slow; AI must make split-second decisions locally to avoid accidents.
Similarly, smart cities are using edge computing to manage traffic flow. AI-driven traffic light systems analyze congestion in real time and adjust signal timings dynamically to reduce bottlenecks and improve commute efficiency.
Self-Service and Augmented Analytics
Empowering Non-Technical Users
Historically, advanced analytics was the domain of data scientists. However, modern self-service analytics platforms are bridging the gap, enabling business professionals to generate insights without needing a PhD in data science.
Take, for instance, drag-and-drop data visualization tools like Tableau or Microsoft Power BI. Marketing teams can now analyze campaign performance, detect customer trends, and adjust strategies without writing a single line of code.
Automating Insight Generation
Augmented analytics—where AI assists in data interpretation—is taking automation even further. Platforms like Google’s Looker and Salesforce Einstein use AI to automatically detect anomalies, suggest optimizations, and generate natural-language insights, turning complex datasets into understandable business recommendations.
For example, a retail manager could receive an automated AI alert saying, “Sales of Product X have dropped 15% in the last two weeks. This correlates with increased customer complaints about shipping delays.” Instead of manually sifting through multiple reports, decision-makers receive actionable insights in real-time.
Industry Applications of Cognitive Analytics
Healthcare: Personalized Medicine & Predictive Diagnostics
AI is revolutionizing healthcare by moving away from one-size-fits-all treatments toward personalized medicine. Cognitive analytics processes genomic data, patient history, and lifestyle factors to recommend customized treatment plans.
For example, IBM Watson Health analyzes medical literature and individual patient data to assist doctors in diagnosing cancer and suggesting the most effective treatment options. In predictive diagnostics, AI-enhanced imaging tools detect early signs of diseases like Alzheimer’s years before symptoms appear, improving early intervention outcomes.
Retail: Customer Insights & Inventory Optimization
Retailers are using cognitive analytics to enhance customer experience and supply chain efficiency.
-
AI-powered recommendation engines personalize shopping experiences—Amazon generates 35% of its sales through AI-driven product recommendations.
-
Retailers like Walmart use RFID and AI analytics to monitor buying patterns in real time, ensuring shelves stay stocked with high-demand products while avoiding overstocking low-selling items.
Finance: Fraud Detection & Risk Management
Financial institutions use cognitive analytics to combat fraud and manage risk more effectively.
-
Visa and Mastercard leverage AI-driven fraud detection to analyze millions of transactions per second, blocking fraudulent transactions while minimizing false positives.
-
JPMorgan Chase’s COiN platform uses AI to analyze legal contracts, reducing document review time from 360,000 hours per year to seconds, streamlining compliance and risk assessment.
Final Thoughts: The Road Ahead
Cognitive analytics is not just a trend—it’s a paradigm shift in how data is processed, analyzed, and applied. From real-time AI-driven decision-making to augmented analytics that democratizes data access, organizations that embrace these advancements will lead their industries.
With technologies like edge computing, VR-driven analytics, and predictive AI models becoming more sophisticated, the future of cognitive analytics is one where data-driven decision-making is instantaneous, intuitive, and more accessible than ever before.
For businesses, the key takeaway is clear: invest in AI-driven analytics now or risk falling behind. The organizations that successfully integrate these technologies will not only optimize operations but also uncover new revenue opportunities and competitive advantages in an increasingly data-driven world.