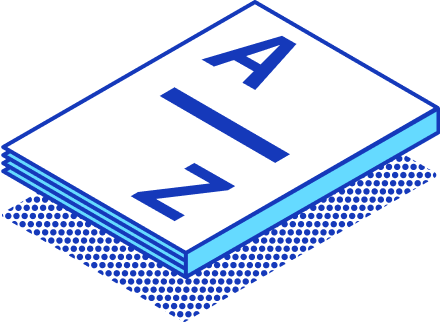
How Fraud Analytics Works
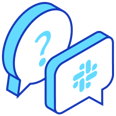
Join StarRocks Community on Slack
Connect on SlackWhat Is Fraud Analytics?
Fraud analytics is the application of data analysis techniques to detect, investigate, and prevent fraudulent behavior. At its core, it involves sifting through vast volumes of transactional, behavioral, and contextual data to identify anomalies, suspicious trends, and emerging fraud patterns. These insights help organizations act proactively—reducing losses, safeguarding systems, and ensuring compliance with financial and regulatory requirements.
Definition and Overview
Fraud analytics is a subset of data analytics that focuses on identifying activities that deviate from expected behavior with the intention to deceive or manipulate systems, especially for financial gain. It blends statistical techniques, machine learning, data mining, and real-time monitoring to build systems that not only detect fraud as it happens but also anticipate it before it occurs.
Examples of fraud types include credit card fraud, identity theft, insurance claim fraud, payroll fraud, insider trading, and healthcare fraud.
Historical Context and Evolution
Early fraud detection systems were manual or rule-based—e.g., flagging transactions over a certain amount or from high-risk geographies. These approaches were brittle and slow to adapt to evolving fraud techniques.
By the 2000s, advancements in data warehousing and business intelligence enabled the first generation of analytics-driven fraud detection. However, high false positive rates remained a problem. The modern era introduced machine learning and AI models capable of adapting to new patterns, dramatically improving accuracy and reducing detection latency. Real-time fraud detection at massive scale became feasible with the adoption of streaming platforms and MPP engines.
Core Techniques in Fraud Analytics
1. Descriptive Analytics: Understanding Historical Fraud Patterns
Descriptive analytics involves analyzing historical data to identify patterns and trends in fraudulent activities. This retrospective analysis helps organizations understand the nature of past fraud incidents, which is crucial for developing effective prevention strategies.
Real-World Application:
In the insurance industry, companies often examine past claims to detect common fraud indicators. For instance, analyzing claims data can reveal patterns such as repeated claims from the same provider for similar injuries, which may indicate fraudulent behavior. By identifying these patterns, insurers can implement more stringent verification processes for suspicious claims.
2. Predictive Analytics: Forecasting Potential Fraud
Predictive analytics uses historical data to forecast future fraudulent activities. By employing machine learning models, organizations can assess the likelihood of a transaction being fraudulent before it occurs, enabling proactive measures.
Real-World Application:
Credit card companies utilize predictive analytics to evaluate transactions in real-time. For example, machine learning models analyze various factors such as transaction amount, location, and time to determine the probability of fraud. If a transaction deviates significantly from a cardholder's typical behavior, it can be flagged for further verification before approval.
3. Prescriptive Analytics: Recommending Preventive Actions
Prescriptive analytics goes beyond prediction by suggesting specific actions to prevent or mitigate fraud based on data insights. This approach enables organizations to implement targeted strategies to combat fraudulent activities effectively.
Real-World Application:
E-commerce platforms employ prescriptive analytics to enhance their fraud prevention measures. For instance, if a transaction is flagged as high-risk based on predictive models, the system may recommend additional verification steps, such as two-factor authentication or manual review, before processing the order. This proactive approach helps in reducing fraudulent transactions while maintaining a seamless customer experience.
4. Anomaly Detection: Identifying Deviations from Norms
Anomaly detection focuses on identifying unusual patterns or behaviors that deviate from established norms, which may indicate fraudulent activity. This technique is particularly useful in detecting new or evolving fraud tactics that do not fit known patterns.
Real-World Application:
Banks monitor account activities for anomalies such as large withdrawals in atypical locations or at unusual times. For example, if a customer's account, which typically has small, local transactions, suddenly shows a large withdrawal in a foreign country, the system can flag this as a potential fraud case for immediate investigation.
5. Network Analysis: Uncovering Fraudulent Connections
Network analysis examines relationships between entities to uncover fraud networks or collusion. By analyzing connections among individuals, accounts, or transactions, organizations can detect complex fraud schemes that may not be evident through individual data points.
Real-World Application:
In combating synthetic identity fraud, financial institutions analyze connections between multiple accounts that share common information, such as phone numbers or addresses. By mapping these relationships, they can identify clusters of accounts likely controlled by a single fraudster, enabling them to take collective action against the fraudulent network.
By integrating these core techniques—descriptive, predictive, prescriptive analytics, anomaly detection, and network analysis—organizations can build robust fraud detection and prevention systems. Each method contributes uniquely to understanding, predicting, and mitigating fraudulent activities, thereby enhancing overall security and trust.
Techniques and Tools
Machine Learning in Fraud Detection
Machine learning (ML) is pivotal in identifying and preventing fraudulent activities. It enables systems to learn from data patterns and make informed decisions.
Supervised Learning
Supervised learning involves training models on labeled datasets, where the outcome (fraudulent or legitimate) is known. Common algorithms include:
-
Logistic Regression: Predicts the probability of a transaction being fraudulent.
-
Decision Trees: Splits data into branches to classify transactions.
-
Random Forests: An ensemble of decision trees to improve accuracy.
-
Gradient Boosting Machines: Focuses on correcting errors from previous models.
-
Neural Networks: Captures complex patterns in data.
Real-World Application: Financial institutions use supervised learning to detect credit card fraud by analyzing transaction histories and identifying patterns associated with fraudulent behavior.
Unsupervised Learning
Unsupervised learning deals with unlabeled data, identifying hidden patterns without predefined outcomes. Techniques include:
-
Clustering (e.g., K-Means): Groups similar transactions to identify anomalies.
-
Principal Component Analysis (PCA): Reduces data dimensionality to highlight unusual patterns.
-
Autoencoders: Neural networks that learn data representations, useful for anomaly detection.
Real-World Application: Unsupervised learning is employed to detect new types of fraud that lack historical data, such as emerging phishing schemes.
Data Mining Techniques
Data mining involves extracting meaningful patterns from large datasets, crucial for uncovering fraudulent activities.
Frequent Pattern Mining
Identifies recurring patterns or associations in data.
Real-World Application: Retailers analyze purchase histories to detect unusual buying patterns that may indicate fraudulent transactions.
Sequence Mining
Discovers sequential patterns, helping to identify the order of events leading to fraud.
Real-World Application: Banks monitor sequences of transactions to detect money laundering activities.
Real-Time Analytics
Real-time analytics processes data instantly, enabling immediate detection and response to fraudulent activities.
Tools:
-
Apache Flink: Processes data streams for real-time analytics.
-
Kafka Streams: Builds real-time applications and microservices.
-
StarRocks: Provides real-time data warehousing capabilities.
Real-World Application: Payment processors use real-time analytics to detect and block fraudulent transactions as they occur.
Feature Engineering
Feature engineering transforms raw data into meaningful features that enhance model performance in fraud detection.
Key Features:
-
Transaction Velocity: Number of transactions in a given time frame.
-
Frequency: Regularity of transactions over a period.
-
Historical Risk Scores: Past behavior metrics indicating risk levels.
Real-World Application: E-commerce platforms analyze transaction velocity to identify rapid purchases that may signify fraudulent activity.
Key Applications Across Industries
Financial Services: Real-Time Fraud Detection
Financial institutions are at the forefront of employing advanced fraud analytics to safeguard against financial crimes.
Case Study: JP Morgan Chase
JP Morgan Chase has developed advanced AI models to enhance its fraud detection capabilities. These models analyze vast datasets to identify suspicious activities, enabling the bank to proactively address potential fraud.
Case Study: Mastercard's Decision Intelligence
Mastercard utilizes its Decision Intelligence technology to analyze cardholders' historical spending habits, establishing behavioral baselines. New transactions are evaluated against these baselines to detect anomalies, enhancing fraud detection accuracy.
Insurance: Combating Fraudulent Claims
The insurance industry faces significant challenges with fraudulent claims, prompting the adoption of predictive analytics and AI-driven solutions.
Case Study: LV Insurance
LV Insurance has reported a 300% increase in cases where apps were used to distort real images and documents for fraudulent claims. To combat this, LV has implemented voice analytics tools to detect signs of fraud in speech, enhancing their ability to identify and prevent fraudulent activities.
Case Study: Alibaba's InfDetect System
Alibaba developed InfDetect, a large-scale graph-based fraud detection system for its e-commerce insurance services. This system processes big graphs containing up to 100 million nodes and billions of edges, successfully detecting thousands of fraudulent claims and saving significant financial resources daily.
E-commerce: Securing Online Transactions
E-commerce platforms are increasingly targeted by fraudsters, necessitating robust fraud detection mechanisms.
Case Study: PayPal
PayPal employs machine learning algorithms to analyze billions of transactions, detecting potentially fraudulent activities in milliseconds. This rapid detection capability guides significant savings and improves customer satisfaction.
Case Study: Forter
Forter, a fraud prevention technology company, applies AI and machine learning to unify identity protection, payments optimization, and fraud prevention. Their technology ensures that legitimate consumers can complete transactions while blocking fraudsters, having decisioned more than $1 trillion in digital commerce transactions.
Business Impact
Financial Loss Mitigation
Effective fraud analytics plays a pivotal role in reducing financial losses by enabling real-time detection and response to fraudulent activities. By identifying suspicious transactions promptly, organizations can prevent significant monetary losses and reduce the costs associated with manual reviews.
Real-World Example:
Alkami Technology implemented Appgate's fraud detection solutions, enhancing its ability to detect and prevent fraudulent activities. This proactive approach strengthened the credit union's fraud prevention measures, safeguarding both its members and financial assets.
Regulatory Compliance
Fraud analytics is instrumental in helping organizations adhere to various regulatory requirements, including Anti-Money Laundering (AML), Know Your Customer (KYC), Payment Card Industry Data Security Standard (PCI DSS), and Health Insurance Portability and Accountability Act (HIPAA). By systematically analyzing transactions and customer data, businesses can detect and report suspicious activities, thereby maintaining compliance and avoiding hefty fines.
Real-World Example:
Socure's compliance solutions assist businesses in meeting regulatory standards by providing comprehensive identity verification and fraud detection services. These tools enable organizations to fulfill their AML and KYC obligations effectively.
Customer Trust
Maintaining customer trust is paramount for any business. Effective fraud prevention measures reassure customers that their data and financial assets are secure, thereby enhancing brand reputation and customer loyalty.
Real-World Example:
A study revealed that nearly two-thirds of consumers believe that fraud incidents damage brand trust and loyalty. This underscores the importance of robust fraud prevention strategies in maintaining customer confidence.
Future Trends in Fraud Analytics
Adaptive AI
Adaptive AI refers to systems that continuously learn and evolve by retraining models based on new fraud patterns and data inputs. This dynamic approach ensures that fraud detection mechanisms remain effective against emerging threats.
Real-World Example:
OmniAI employs continuous retraining through automated feedback loops, refining models to stay effective against rapidly changing fraud tactics. The AI Journal
Federated Learning
Federated learning enables multiple organizations to collaboratively train machine learning models without sharing sensitive data. This approach enhances fraud detection capabilities while preserving data privacy.Vogue Business+1authenticate.com+1IAEME
Real-World Example:
Financial institutions are adopting federated learning to detect financial crimes collaboratively, improving detection rates without compromising data privacy. Amazon Web Services, Inc.
Graph Neural Networks (GNNs)
GNNs are advanced machine learning models that analyze relationships and interactions within data, making them particularly effective in detecting complex fraud schemes involving networks of fraudulent activities.
Real-World Example:
Research indicates that GNNs are exceptionally adept at capturing complex relational patterns within financial networks, significantly outperforming traditional fraud detection methods.
Explainable AI (XAI)
XAI focuses on making AI decisions transparent and understandable, which is crucial for regulatory compliance and building trust with stakeholders. By providing clear explanations for decisions, organizations can ensure accountability and meet regulatory requirements.
Real-World Example:
Explainable AI improves regulatory and compliance processes by making AI systems' decisions transparent and auditable, aiding organizations in meeting transparency mandates.
Data Quality & Governance
High-quality, well-governed data is essential for accurate fraud detection. Ensuring data accuracy, consistency, and integrity enhances the reliability of fraud analytics models.
Real-World Example:
Mastercard emphasizes the importance of data quality in fraud prevention, noting that incomplete or inaccurate data can negatively impact results and lead to revenue loss.
Conclusion
Fraud analytics has become an indispensable tool in the modern digital economy. By leveraging advanced data analysis techniques, organizations can proactively detect and prevent fraudulent activities, safeguarding financial assets and maintaining customer trust. The integration of machine learning, real-time analytics, and explainable AI ensures that fraud detection systems are both effective and transparent. As fraudsters continue to evolve their tactics, staying ahead requires continuous innovation and collaboration across industries.
Frequently Asked Questions (FAQ)
Q1: What is fraud analytics?
A1: Fraud analytics involves the use of data analysis techniques to detect, investigate, and prevent fraudulent activities. It encompasses methods like statistical analysis, machine learning, and real-time monitoring to identify anomalies and suspicious patterns in data.
Q2: How does machine learning enhance fraud detection?
A2: Machine learning algorithms can learn from historical data to identify patterns associated with fraudulent behavior. They can adapt to new fraud tactics over time, improving detection accuracy and reducing false positives.
Q3: What is the difference between supervised and unsupervised learning in fraud detection?
A3: Supervised learning uses labeled datasets to train models to recognize known fraud patterns, while unsupervised learning identifies anomalies in data without predefined labels, making it useful for detecting new or evolving fraud schemes.
Q4: Why is real-time analytics important in fraud prevention?
A4: Real-time analytics allows organizations to detect and respond to fraudulent activities as they occur, minimizing potential losses and preventing further fraudulent transactions.
Q5: How does explainable AI (XAI) contribute to fraud analytics?
A5: Explainable AI provides transparency into how AI models make decisions, which is crucial for regulatory compliance and building trust with stakeholders. It helps organizations understand the rationale behind fraud detection outcomes.
Q6: What role does data quality play in fraud analytics?
A6: High-quality, accurate, and well-governed data is essential for effective fraud detection. Poor data quality can lead to inaccurate models, increased false positives or negatives, and ultimately, financial losses.
Q7: How is federated learning used in fraud detection?
A7: Federated learning enables multiple organizations to collaboratively train machine learning models without sharing sensitive data. This approach enhances fraud detection capabilities while preserving data privacy.
Q8: What are Graph Neural Networks (GNNs), and how are they applied in fraud detection?
A8: GNNs are advanced machine learning models that analyze relationships and interactions within data. They are particularly effective in detecting complex fraud schemes involving networks of fraudulent activities.
Q9: How does fraud analytics help in regulatory compliance?
A9: Fraud analytics assists organizations in adhering to regulations like AML, KYC, PCI DSS, and HIPAA by systematically analyzing transactions and customer data to detect and report suspicious activities, thereby maintaining compliance and avoiding penalties.
Q10: What is the future of fraud analytics?
A10: The future of fraud analytics lies in adaptive AI systems that continuously learn from new data, the adoption of federated learning for collaborative model training, the use of GNNs for complex network analysis, and a strong emphasis on data quality and explainability to meet evolving regulatory and security challenges.
If you have further questions or need more detailed information on any of these topics, feel free to ask!