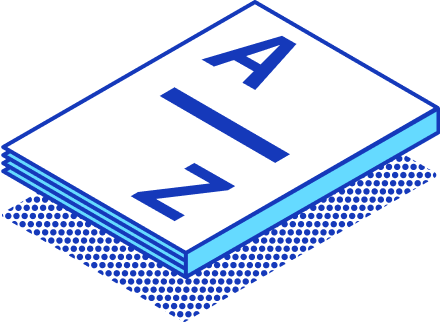
Hypothesis Testing
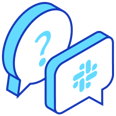
Join StarRocks Community on Slack
Connect on SlackWhat Is Hypothesis Tests?
Definition and Purpose
Understanding the concept of a hypothesis is essential in statistics. A hypothesis is an assumption about a population parameter. Researchers use hypothesis tests to evaluate these assumptions with sample data. This method provides a structured way to make decisions based on evidence.
Hypothesis testing plays a vital role in statistics. Analysts use it to determine if sample data supports a particular theory. The process involves comparing observed results with expected outcomes. This comparison helps assess the validity of the hypothesis.
Types of Hypotheses
Null Hypothesis
The null hypothesis represents a statement of no effect or no difference. Researchers assume that any observed effect in the sample data occurs by chance. The goal is to test this assumption against the alternative hypothesis. For example, in medicine, researchers might test if a new drug has no effect compared to a placebo.
Alternative Hypothesis
The alternative hypothesis contradicts the null hypothesis. It suggests that there is an effect or a difference. Researchers aim to gather evidence to support this claim. In market research, companies might test if a new marketing strategy affects consumer behavior. The alternative hypothesis drives the research objective.
Scientific Research Findings:
-
Hypothesis Testing in Medicine and Healthcare: Researchers use hypothesis testing to determine the effectiveness of new treatments.
-
Hypothesis Testing in Market Research: Companies validate assumptions about consumer preferences through hypothesis tests.
Jim Frost highlights the importance of understanding both parametric and nonparametric tests. Nonparametric tests vs. parametric tests offer flexibility when traditional assumptions do not hold. This flexibility ensures accurate results even when data does not fit standard models.
The Process of Hypothesis Testing
Steps Involved
Formulating Hypotheses
Start by creating a clear hypothesis. A hypothesis is an assumption about a population parameter. You need both a null hypothesis and an alternative hypothesis. The null hypothesis suggests no effect or difference. The alternative hypothesis proposes an effect or difference. These hypotheses guide your research.
Choosing the Significance Level
Select a significance level for your hypothesis testing. This level shows how much risk you accept when rejecting the null hypothesis. Common levels include 0.05 or 0.01. A lower significance level means stricter criteria for rejecting the null hypothesis. This choice impacts the reliability of your results.
Selecting the Appropriate Test
Choose the right test based on your data. Different tests suit different types of data and research questions. A Z-test works well for large sample sizes. A T-test fits smaller samples. Use a Chi-square test for categorical data. Each test has specific assumptions and applications.
Making a Decision
Analyze the sample data and make a decision. Compare the results to the significance level. If the results fall within the critical region, reject the null hypothesis. If not, fail to reject it. This decision helps determine if the sample supports your hypothesis.
Common Tests Used
Z-test
Use a Z-test for large sample sizes. This test assumes normal distribution in the population. It compares the sample mean to the population mean. A Z-test helps evaluate if the sample data significantly differs from the population.
T-test
A T-test compares mean scores between two groups. This test suits smaller sample sizes. It checks if the sample means differ significantly. The T-test requires assumptions like normality and equal variances in the population.
Chi-square Test
The Chi-square test explores relationships between categorical variables. This test checks if observed frequencies differ from expected frequencies. Use it to analyze data in contingency tables. The Chi-square test helps understand associations in categorical data.
Assumptions in Hypothesis Testing
Importance of Assumptions
Assumptions form the backbone of hypothesis testing. Each assumption ensures that the results of a test are valid and reliable. Ignoring these assumptions can lead to incorrect conclusions about a population.
Normality
Normality assumes that the data follows a normal distribution. This assumption is crucial for many statistical tests. A normal distribution means most data points cluster around the mean. Tests like the T-test rely on this assumption to compare sample means accurately. If the data does not meet this assumption, the results may not reflect the true characteristics of the population.
Independence
Independence means that each data point in a sample is not influenced by others. This assumption ensures that the sample represents the population without bias. In clinical trials, researchers must ensure that one treatment does not affect another. Independence helps maintain the integrity of the hypothesis being tested. Without it, the data might show misleading patterns.
Homogeneity of Variance
Homogeneity of variance assumes that different samples have similar variances. This assumption is vital when comparing groups. For example, in a study comparing two treatments, both groups should have similar variability. If the variances differ significantly, the test results might be skewed. Homogeneity ensures that the comparison between groups is fair and accurate.
Understanding these assumptions helps you conduct hypothesis testing effectively. By respecting these principles, you ensure that your data analysis leads to meaningful insights.
Applications of Hypothesis Testing
Hypothesis testing plays a vital role in various real-world applications. You can use it to make informed decisions based on data. The following examples illustrate how hypothesis testing impacts different fields.
Real-world Examples
Business Decision Making
Businesses rely on hypothesis testing to make strategic decisions. Companies often test assumptions about market trends or consumer behavior. For instance, a company might want to know if a new advertising campaign increases sales. The null hypothesis would state that the campaign has no effect on sales. Analysts collect sample data from sales before and after the campaign. If the data shows a significant increase, the company may reject the null hypothesis. This process helps businesses make data-driven decisions.
Medical Research
Medical researchers use hypothesis testing to evaluate new treatments. A common scenario involves testing the effectiveness of a new drug. Researchers start with a null hypothesis stating that the drug has no effect on patients. They then administer the drug to a sample population and observe the results. If the data indicates a significant improvement in patients, researchers may reject the null hypothesis. This method ensures that medical decisions are based on empirical evidence.
Social Sciences
Social scientists apply hypothesis testing to study human behavior and societal trends. Researchers might explore whether a new educational program improves student performance. The null hypothesis would suggest no difference in performance between students who participate in the program and those who do not. Researchers gather data from both groups and analyze the results. If the data supports a significant difference, the null hypothesis is rejected. This approach allows social scientists to draw conclusions about the population based on study results.
Hypothesis testing provides a structured framework for making inferences about a population. You can use it to validate assumptions and draw conclusions from data. This method is essential for generating reliable insights across various fields.
Limitations and Challenges
Potential Pitfalls
Misinterpretation of results
Misinterpretation of results can lead to incorrect conclusions. Analysts often misjudge statistical significance as practical importance. Statistical significance only indicates a low probability of the null hypothesis being true. Analysts must consider the context and real-world implications. Misinterpretation can also occur when analysts ignore assumptions. Analysts must ensure that data meets all necessary criteria before drawing conclusions.
Over-reliance on p-values
Over-reliance on p-values can mislead decision-making. P-values indicate the probability of observing data given the null hypothesis is true. A small p-value suggests strong evidence against the null hypothesis. However, p-values do not measure effect size or practical significance. Solely relying on p-values can result in overlooking important factors. Analysts should use p-values alongside other statistical measures for comprehensive analysis.
Addressing Challenges
Ensuring proper data collection
Proper data collection ensures accurate hypothesis testing. Analysts must design studies carefully to avoid bias. Random sampling helps achieve representative data. Analysts should also account for confounding variables. Proper data collection minimizes errors and enhances reliability. Analysts must document methods clearly for transparency and reproducibility.
Understanding the context of results
Understanding the context of results aids in meaningful interpretation. Analysts should consider the research question and objectives. Contextual factors influence the applicability of findings. Analysts must relate statistical results to real-world scenarios. Understanding context helps bridge the gap between theory and practice. This approach leads to informed decisions and actionable insights.
Conclusion
Hypothesis testing serves as a vital tool for making data-driven decisions. Researchers and practitioners use hypothesis testing to validate assumptions and support claims with statistical evidence. The logic of hypothesis testing enables you to critically analyze test results. You can apply hypothesis testing in various fields to enhance decision-making processes. Understanding the scientific method strengthens your ability to challenge expert analysis. Embrace hypothesis testing to draw meaningful conclusions and improve research outcomes.