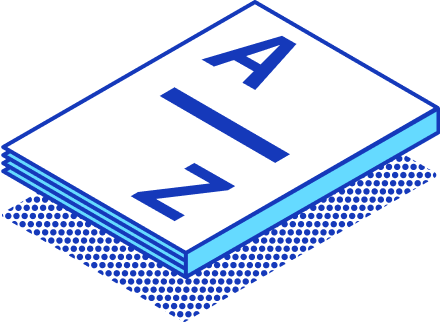
Knowledge Graphs
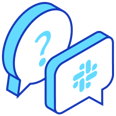
Join StarRocks Community on Slack
Connect on SlackWhat Is Knowledge Graphs
Definition and Origin
Knowledge Graphs have transformed how data is organized and understood. The roots of Knowledge Graphs trace back to the 1960s with semantic networks and frame languages in the 1970s. These early developments laid the groundwork for today's advanced systems. The evolution of Knowledge Graphs has paralleled advancements in fields like artificial intelligence and information retrieval. The concept has grown significantly in academia, revolutionizing interactions with AI.
Historical Background
The historical journey of Knowledge Graphs began with early semantic networks. These networks aimed to represent relationships between entities. Frame languages in the 1970s further developed this idea. The progression continued alongside the Semantic Web's growth. This evolution has led to the sophisticated Knowledge Graphs used today. Jason Barnard, a notable figure in Digital Marketing, emphasizes the importance of understanding these structures for enhancing online presence.
Key Characteristics
A Knowledge Graph represents a network of real-world entities. These entities include objects, events, situations, or concepts. The relationships between them form the core of the Graph. A Knowledge Graph organizes data from multiple sources, enabling integration and analytics. The Google Knowledge Graph changed how information is retrieved by analyzing word meanings in queries. This system allows Google to answer factual questions effectively. The Google Knowledge Graph represents a powerful tool for enhancing search experiences.
Core Components
Understanding the core components of a Knowledge Graph is essential. These components define how data is structured and accessed.
Nodes and Edges
Nodes and edges form the backbone of a Knowledge Graph. Nodes represent entities such as people, places, or things. Edges illustrate the relationships between these nodes. This structure allows for a clear representation of interconnected data. A Graph Database stores these relationships natively. This storage method enhances data retrieval and integration.
Ontologies and Taxonomies
Ontologies and taxonomies provide a framework for organizing data within a Knowledge Graph. Ontologies define the types of entities and their relationships. Taxonomies classify these entities into hierarchical structures. This organization facilitates efficient data access and analysis. Google uses these principles to understand your content better. The Google Knowledge Graph helps Google understand your content contextually. This understanding improves search result relevance and accuracy.
How Knowledge Graphs Work
Data Integration
Data Sources
Knowledge Graphs integrate diverse data sources to create a unified view of information. Google uses this approach to gather data from various platforms and databases. The integration process involves collecting structured and unstructured data. This data includes text, images, and videos. Google ensures that the data remains accurate and up-to-date. The integration of multiple data sources enhances the richness of the Knowledge Graph.
Data Linking
Data linking plays a crucial role in Knowledge Graphs. Google connects related data points to form meaningful relationships. This process involves identifying entities and their associations. Google uses algorithms to link data accurately. The connections between data points create a network of information. This network allows users to explore related content easily. Data linking enhances the ability of Google to provide relevant search results.
Querying and Retrieval
SPARQL and Other Query Languages
SPARQL serves as a query language for retrieving information from Knowledge Graphs. Google employs SPARQL to access specific data within the graph. This language allows users to perform complex searches efficiently. Google also uses other query languages to enhance retrieval capabilities. These languages enable precise data extraction from the Knowledge Graph. The use of query languages improves the search experience for users.
Semantic Search
Semantic search enhances the way Google retrieves information. This approach focuses on understanding the meaning behind user queries. Google analyzes the context of words to deliver accurate results. Semantic search leverages the structure of the Knowledge Graph. This structure helps Google interpret the intent of user queries. The use of semantic search improves the relevance of search results. Google continues to refine this technology to provide better user experiences.
Enterprise Knowledge Graph and Its Applications
Industry Use Cases
Healthcare
The healthcare industry benefits significantly from the Enterprise Knowledge Graph. Hospitals and clinics use these graphs to manage patient records efficiently. The Enterprise Knowledge Graph platform organizes patient data, treatment histories, and medication details. This organization allows healthcare providers to make informed decisions quickly. The relationships between different medical entities become clear through the graph structure. The Enterprise Knowledge Graph enhances the ability to track disease outbreaks by linking various data sources. Researchers utilize these graphs to identify patterns in patient data. This identification aids in developing new treatments and improving patient care.
E-commerce
E-commerce platforms leverage the Enterprise Knowledge Graph to enhance customer experiences. Online retailers like Madave Shoes use these graphs to organize product information. The Enterprise Knowledge Graph platform connects product details, customer reviews, and purchase histories. This connection enables personalized recommendations for shoppers. Retailers analyze data to understand customer preferences better. The Enterprise Knowledge Graph improves inventory management by linking sales data with supply chain information. This improvement ensures that products remain available when customers need them. The graph structure helps businesses like Madave Shoes optimize their marketing strategies.
Enhancing AI and Machine Learning
Natural Language Processing
Natural Language Processing (NLP) benefits greatly from the Enterprise Knowledge Graph. NLP algorithms use the graph to understand the context of words and phrases. The Enterprise Knowledge Graph platform provides a structured framework for analyzing text data. This framework enhances the accuracy of language models. Google and Bing employ knowledge graphs to improve search engine capabilities. These engines interpret user queries more effectively using organizing principles. The Enterprise Knowledge Graph assists in translating languages by identifying semantic relationships. This assistance leads to more accurate translations and better communication.
Benefits and Challenges of Knowledge Graphs
Knowledge Graphs have transformed how organizations manage and utilize data. These systems offer significant advantages, but they also present challenges that require careful consideration.
Advantages
Improved Data Accessibility
Knowledge Graphs enhance data accessibility by organizing information in a structured manner. Google uses these graphs to connect various data points, making it easier for users to access relevant information quickly. The interconnected nature of Knowledge Graphs allows users to navigate through complex datasets with ease. This improved accessibility supports better decision-making processes across different industries.
Enhanced Decision Making
Knowledge Graphs provide a comprehensive view of data relationships, which aids in decision-making. Google leverages these graphs to analyze vast amounts of data and extract meaningful insights. By understanding the connections between entities, organizations can make informed decisions based on accurate and up-to-date information. The ability to visualize data relationships enhances strategic planning and operational efficiency.
Limitations
Complexity in Implementation
Implementing Knowledge Graphs involves significant complexity. Organizations must design ontologies and integrate heterogeneous data sources effectively. Google faces challenges in ensuring data quality and scalability within its Knowledge Graph systems. The complexity of implementation requires specialized expertise and resources to maintain the integrity of the graph structure.
Data Privacy Concerns
Data privacy remains a critical concern when utilizing Knowledge Graphs. Google must address privacy issues related to the collection and integration of sensitive information. Ensuring compliance with data protection regulations is essential to maintain user trust. Organizations must implement robust security measures to safeguard data within Knowledge Graphs and prevent unauthorized access.
Knowledge Graphs continue to shape the future of data management and analysis. Despite the challenges, their potential to revolutionize industries remains undeniable. The ongoing advancements in AI and machine learning will further enhance the capabilities of Knowledge Graphs, offering new opportunities for innovation.
AI-Augmented Knowledge Graphs and Future Directions
Emerging Trends
Integration with IoT
The integration of Knowledge Graphs with the Internet of Things (IoT) represents a significant trend in data management. IoT devices generate vast amounts of data. Knowledge Graphs organize this data efficiently. The interconnected nature of IoT devices aligns with the structure of a Graph. Each device acts as a node within the Graph. Relationships between devices form edges. This integration enhances real-time data processing. AI-Augmented Knowledge Graphs facilitate dynamic interactions among IoT devices. The ability to process data in real-time improves decision-making capabilities.
Advances in AI
Advances in artificial intelligence drive the evolution of Knowledge Graphs. AI technologies enhance the construction and functionality of these Graphs. Machine learning algorithms analyze data patterns within the Graph. This analysis uncovers hidden relationships among entities. AI augments the capability of Knowledge Graphs to adapt to new information. The dynamic nature of AI-Augmented Knowledge Graphs allows for continuous learning. These advancements improve the accuracy of data retrieval. Future Knowledge Graphs will integrate seamlessly with AI systems. This integration will enable more sophisticated data analysis.
Research and Development
Ongoing Projects
Research and development efforts focus on enhancing Knowledge Graphs. Current projects aim to create more dynamic and cross-lingual Graphs. Researchers explore methods to integrate scientific workflows into Knowledge Graphs. These efforts seek to improve the representation of complex data structures. The goal is to develop Knowledge Graphs that can adapt to changing data environments. Ongoing projects emphasize the importance of real-time data processing. The ability to update Graphs dynamically enhances their relevance.
Potential Innovations
Potential innovations in Knowledge Graphs include the development of more intuitive interfaces. These interfaces will simplify the process of Knowledge Graph Construction. Users will interact with Graphs in more natural ways. Innovations will also focus on improving data privacy measures. Secure data handling will become a priority in Knowledge Graph listings. The future of Knowledge Graphs involves creating systems that can understand context better. These systems will provide more accurate insights by analyzing data relationships. The potential for innovation in Knowledge Graphs remains vast.
Conclusion
Knowledge Graphs have revolutionized how organizations manage and utilize data. These structures offer a flexible and intuitive way to represent complex knowledge. The ability to store information about entities and their relationships enhances the understanding of real-world knowledge. Knowledge Graphs provide a promising tool for academia, business, and various industries. The potential to shape the future of academic research remains significant. Exploring further resources and developments in Knowledge Graph technology is essential. The evolution of these systems will continue to influence AI interactions and SERP performance.