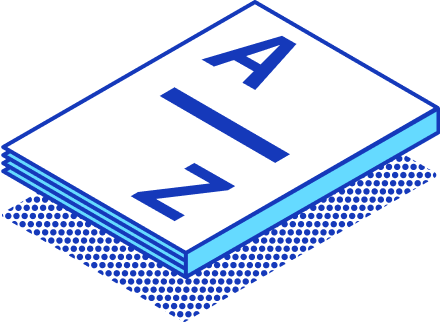
Manufacturing Analytics
What Is Manufacturing Analytics
Manufacturing Analytics involves the systematic use of data to enhance manufacturing processes. This approach focuses on collecting, analyzing, and interpreting data to improve decision-making. Manufacturers use this data-driven strategy to optimize production, reduce costs, and increase efficiency.
Key Components
Manufacturing Analytics consists of several key components:
-
Data Collection: Gathering data from various sources, including machines and sensors.
-
Data Analysis: Using statistical methods and algorithms to interpret data.
-
Visualization: Presenting data insights through dashboards and reports.
-
Decision Support: Providing actionable insights for informed decision-making.
How It Differs from Traditional Analytics
Traditional analytics often relies on historical data and manual processes. Manufacturing Analytics, however, emphasizes real-time data and automated systems. This approach enables faster responses to changes in production and market demands.
Importance in Modern Manufacturing
Manufacturing Analytics plays a vital role in today's manufacturing landscape. The integration of data analytics into manufacturing processes leads to significant improvements in efficiency and productivity.
Role in Industry 4.0
Industry 4.0 represents a new era of manufacturing characterized by smart factories and interconnected systems. Manufacturing Analytics enables manufacturers to leverage big data for enhanced operational efficiency. This approach supports decentralized production and resource optimization.
Impact on Efficiency and Productivity
Manufacturers achieve higher efficiency and productivity through Manufacturing Analytics. Real-time insights allow for quick adjustments in production processes. This leads to reduced downtime and improved product quality. Accurate forecasting and inventory management become achievable, driving innovation and competitiveness.
How Manufacturing Analytics Work
Data Collection and Analysis
Manufacturing Analytics Explained involves a systematic approach to data collection and analysis. Manufacturers gather data from various sources to enhance production processes. The data collected includes information from machines, sensors, and production lines. This comprehensive data collection forms the foundation for effective analytics.
Techniques Used
Manufacturers employ several techniques to analyze data. Statistical methods play a crucial role in interpreting the data collected. Algorithms process large volumes of data to identify patterns and trends. Machine learning models predict outcomes based on historical data. These techniques enable manufacturers to make informed decisions.
Tools and Technologies
Advanced tools and technologies support data analysis in manufacturing. Software platforms like MachineMetrics automate data collection and analysis. These platforms provide real-time insights into production processes. Visualization tools present data in an accessible format. Dashboards and reports offer stakeholders a clear view of operational performance.
Real-Time Data Utilization
Real-time data utilization transforms manufacturing workflows. Manufacturers access live data to make immediate adjustments. This approach improves efficiency and product quality. Real-time insights optimize supply chain management.
Benefits of Real-Time Insights
Real-time insights offer significant benefits. Manufacturers reduce machine downtime by responding quickly to issues. Production processes become more efficient with timely interventions. Real-time data enhances quality control by identifying defects early. Manufacturers achieve better resource allocation and inventory management.
Case Examples
Predictive Maintenance in Manufacturing: Predictive maintenance uses data analytics to prevent disruptions. Manufacturers analyze data to predict equipment failures. This proactive approach reduces downtime and maintenance costs. Case studies demonstrate how predictive maintenance improves operational efficiency.
Data Analytics Transforming Workflows: Data analytics plays a vital role in transforming manufacturing workflows. Manufacturers use real-time data to streamline operations. Improved efficiency leads to higher productivity and better quality products. Supply chain management benefits from accurate demand forecasting.
Benefits of Manufacturing Analytics
Manufacturing analytics plays a crucial role in enhancing operational efficiency and reducing costs in manufacturing processes. By leveraging data analytics, manufacturers can optimize equipment performance, minimize waste, and improve quality control.
Operational Efficiency
Operational efficiency is a key benefit of manufacturing analytics. Data analytics enables manufacturers to monitor equipment performance in real-time, leading to significant improvements in operational efficiency.
Reducing Downtime
Reducing downtime is essential for maximizing productivity in manufacturing processes. Data analytics provides insights into equipment health and performance. Manufacturers can predict potential failures and schedule maintenance proactively. This approach minimizes unexpected breakdowns and reduces downtime.
Enhancing Quality Control
Enhancing quality control is another critical aspect of operational efficiency. Data analytics allows manufacturers to monitor production processes closely. Equipment sensors collect data on various parameters, ensuring consistent product quality. Real-time analysis helps identify defects early, enabling timely corrective actions.
Cost Reduction
Cost reduction is a significant advantage of implementing manufacturing analytics. By optimizing equipment usage and resource allocation, manufacturers can achieve substantial cost savings.
Waste Minimization
Waste minimization is a primary goal in manufacturing processes. Data analytics helps identify inefficiencies and areas of waste. Manufacturers can analyze production data to optimize material usage and reduce scrap. This approach leads to cost savings and environmental benefits.
Energy Management
Energy management is crucial for reducing operational costs. Data analytics provides insights into energy consumption patterns. Manufacturers can optimize equipment settings and schedules to minimize energy usage. This strategy not only reduces costs but also supports sustainability initiatives.
Practical Applications and Business Cases for Manufacturing Analytics
Manufacturing analytics transforms operations through practical applications and real-world business cases. Manufacturers use data analytics to enhance efficiency and improve decision-making. The integration of analytics projects into manufacturing processes leads to significant advancements.
Predictive Maintenance
Predictive maintenance represents a key application in manufacturing analytics. Manufacturers use data analytics to reduce unexpected equipment failures. This approach optimizes maintenance schedules and minimizes costs.
Techniques and Tools
Manufacturers employ various techniques and tools for predictive maintenance. Machine learning algorithms analyze historical data to predict equipment failures. Automated systems generate maintenance requests based on these predictions. Manufacturers use analytics systems to monitor equipment health in real-time. These tools ensure optimal decision-making and resource allocation.
Case Studies
Automated Equipment Diagnostics: Manufacturers implemented predictive analytics to automate equipment diagnostics. This approach led to a reduction in maintenance costs and improved operational efficiency. Automated systems anticipated equipment failures, allowing timely interventions.
Sector-Specific Applications: Manufacturers applied predictive analytics in sector-specific scenarios. In the food industry, analytics identified the ripeness of fruits and optimized sorting processes. Cleaning equipment benefited from predictive maintenance, reducing downtime and enhancing productivity.
Supply Chain Optimization
Supply chain optimization is another critical application of manufacturing analytics. Manufacturers use data analytics to streamline inventory management and improve demand forecasting. This approach enhances customer satisfaction and operational efficiency.
Inventory Management
Manufacturers leverage analytics projects to optimize inventory management. Data analytics identifies patterns in inventory usage and demand fluctuations. Manufacturers adjust inventory levels based on these insights, reducing excess stock and minimizing waste. Real-time monitoring ensures efficient resource allocation and cost savings.
Demand Forecasting
Demand forecasting is a vital component of supply chain optimization. Manufacturers use data analytics to predict customer demand accurately. Analytics systems analyze historical sales data and market trends to forecast future demand. This approach enables manufacturers to align production schedules with customer needs, enhancing satisfaction and competitiveness.
Best Practices for Implementing Data Analytics in Manufacturing
Implementing Data Analytics in manufacturing requires a strategic approach. Manufacturers must focus on data collection and management to ensure success. Effective data management forms the foundation for reliable insights.
Data Collection and Management
Data collection serves as the first step in the analytics journey. Manufacturers gather data from machines, sensors, and production lines. This comprehensive approach captures valuable information for analysis.
Ensuring Data Quality
Data quality remains crucial for accurate insights. Manufacturers must implement rigorous data validation processes. Regular audits help maintain data integrity. High-quality data leads to reliable analytics outcomes.
Integrating Data Sources
Integrating data sources enhances the value of analytics. Manufacturers combine data from various systems for a holistic view. This integration supports comprehensive analysis and informed decision-making.
Choosing the Right Tools
Selecting appropriate tools is essential for successful analytics implementation. Manufacturers must evaluate software solutions that align with their needs. The right tools facilitate efficient data processing and visualization.
Software Solutions
LineView Smart Factory Software offers robust solutions for manufacturing analytics. This software unlocks efficiency and innovation in production processes. Reliable tools like LineView enhance data-driven decision-making.
Custom vs. Off-the-Shelf
Manufacturers face a choice between custom and off-the-shelf solutions. Custom solutions offer tailored features for specific needs. Off-the-shelf options provide quick deployment and cost-effectiveness. Manufacturers must assess their requirements to make an informed choice.
Implement Data Analytics effectively to transform manufacturing operations. Data Insights for Efficient Manufacturing empower organizations to run efficiently. These insights prepare manufacturers for a competitive future. Predictive Analytics for Manufacturing Operations extract valuable insights from complex data. This approach identifies future opportunities in production. Data Visualization for Manufacturing Insights enhances understanding through dashboards. Visualization unlocks insights about manufacturing processes.
Future of Manufacturing Analytics
AI and Machine Learning Integration
Artificial Intelligence (AI) and Machine Learning (ML) are revolutionizing the manufacturing industry. These technologies enhance predictive analytics, enabling manufacturers to anticipate equipment failures and optimize maintenance schedules. Predictive analytics also supports quality control automation and material procurement decision-making. AI-driven systems analyze vast amounts of data to identify patterns and inefficiencies in production processes. This capability leads to improved efficiency and product consistency.
Emerging Technologies
Emerging technologies in AI and ML continue to transform manufacturing analytics. Advanced algorithms process real-time data from machines and sensors. This data provides insights into equipment performance and potential issues. Manufacturers use these insights to make informed decisions about production and maintenance. AI-powered systems also facilitate early defect identification and predictive quality issue resolution.
Potential Challenges
Despite the benefits, integrating AI and ML into manufacturing analytics presents challenges. Data security and privacy concerns arise due to the vast amount of information collected. Manufacturers must ensure that data is protected from unauthorized access. Additionally, the complexity of AI systems requires skilled personnel for implementation and maintenance. Training employees to Work with these technologies is essential for successful integration.
Real-Time Analytics
Real-time analytics plays a crucial role in modern manufacturing. This approach allows manufacturers to monitor production processes continuously. Real-time data provides immediate insights into equipment performance and supply chain operations. Manufacturers use these insights to optimize production schedules and resource allocation.
Benefits and Limitations
Real-time analytics offers several benefits. Manufacturers achieve higher efficiency by responding quickly to production issues. Real-time data enhances quality control by identifying defects early. This approach also improves supply chain management through accurate demand forecasting. However, real-time analytics requires significant investment in technology and infrastructure. Manufacturers must balance the costs with the potential benefits.
Industry Examples
Several industries benefit from real-time analytics in manufacturing. The automotive sector uses real-time data to monitor assembly lines and ensure product quality. This approach reduces downtime and increases productivity. In the electronics industry, manufacturers use real-time analytics to optimize supply chain operations. Accurate demand forecasting leads to better inventory management and customer satisfaction.
Conclusion
Manufacturing analytics holds transformative potential for the industry. This approach empowers manufacturers to optimize supply chain management, streamline production flow, and enhance product quality. Predictive analytics provides valuable insights from complex data, enabling future opportunities. Machine learning and statistical modeling play crucial roles in this process. Adopting manufacturing analytics leads to increased efficiency, innovation, and quality. Manufacturers gain a competitive advantage by integrating these advanced tools into their operations. Embracing analytics positions companies for success in the evolving manufacturing landscape.