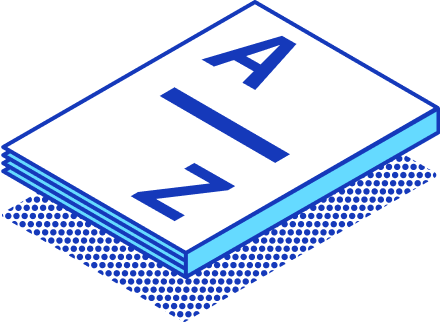
Real-Time Fraud Detection: Key Trends to Watch
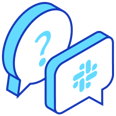
Join StarRocks Community on Slack
Connect on SlackReal-time fraud detection plays a crucial role in safeguarding financial assets. As fraudsters employ sophisticated methods, organizations must stay updated with key trends to protect their interests. The evolving landscape of fraud detection demands continuous adaptation. Financial services companies lose tens of billions of dollars annually to fraud attacks, highlighting the urgency for advanced systems. A Case Study reveals that contactless fraud rose by 82% in the UK in 2023. Moreover, card ID theft increased by 97%, causing significant losses. This Article underscores the importance of proactive measures in combating fraud.
Technological Advancements in Fraud Detection
Machine Learning and AI
Machine learning and AI have revolutionized the fraud detection workflow by enhancing predictive analytics. These technologies analyze vast datasets to identify patterns that indicate potential fraud. AI-powered fraud detection systems leverage historical data to predict and prevent fraud attempts earlier in the payment process. This proactive approach allows organizations to respond swiftly to threats, reducing the risk of financial loss.
Scientific Research Findings:
-
AI's Role in Financial Fraud Detection Advancements: AI advancements with blockchain and biometrics promise more accurate and secure fraud prevention methods.
-
AI Fraud Detection vs. Traditional Methods: AI is generally more effective than traditional methods, leveraging data patterns and predictive analytics for faster and more accurate detection.
Anomaly detection plays a crucial role in this process. By continuously monitoring transactions, AI-ML event processing can identify deviations from normal behavior, flagging them as suspicious. This capability enhances the overall fraud detection workflow, ensuring that organizations remain vigilant against evolving threats.
Blockchain Technology
Blockchain technology offers significant benefits for transaction verification and data integrity. Its decentralized nature ensures that all transactions are transparent and immutable, making it difficult for fraudsters to alter records. This technology enhances data sharing in fraud detection, allowing institutions to collaborate more effectively.
Scientific Research Findings:
-
Investing in Advanced Technologies for Fraud Prevention: Investing in advanced technologies like AI and machine learning can help institutions analyze vast amounts of data quickly and accurately, enabling them to respond and detect fraudulent activities more effectively.
Data sharing in fraud detection becomes more secure with blockchain, as it provides a reliable platform for exchanging information without compromising privacy. This innovation supports the AIM of creating a robust defense against fraud by facilitating seamless data and intelligence sharing among stakeholders.
Biometric Authentication
Biometric authentication has emerged as a powerful tool in fraud prevention. Various types of biometric technologies, such as fingerprint and facial recognition, offer enhanced security by verifying identities based on unique physical traits. These technologies reduce the risk of identity theft and unauthorized access, providing an additional layer of protection.
The impact of biometric authentication on fraud prevention is profound. By integrating these technologies into their systems, organizations can ensure that only authorized individuals gain access to sensitive information. This approach not only strengthens security measures but also improves user experience by streamlining authentication processes.
Emerging Threats in Fraud Detection
Sophisticated Cyber Attacks
Fraudsters continuously evolve their tactics, making sophisticated cyber attacks a significant threat. Phishing and social engineering represent two of the most prevalent methods. Attackers manipulate individuals into revealing sensitive information, often through deceptive emails or messages. These tactics exploit human psychology, making them particularly effective.
Ransomware and malware further complicate the landscape of cybercrime. These malicious programs infiltrate systems, encrypting data and demanding ransom for its release. Organizations face immense pressure to protect their data from such threats. The FBI Internet Crime Complaint center reports a surge in these attacks, emphasizing the need for robust defenses.
Case Study: Mastercard's Response to Fraudulent Transactions
-
Mastercard employs dynamic detection and prevention strategies.
-
They adapt to evolving fraud techniques, showcasing the importance of predictive approaches.
Insider Threats
Insider threats pose unique challenges in fraud detection. Employees with access to sensitive information can exploit their positions for personal gain. Detecting these threats requires a nuanced approach. Organizations must implement detection strategies that monitor employee behavior without infringing on privacy.
Mitigation techniques play a crucial role in addressing insider threats. Companies should establish clear policies and conduct regular audits to identify potential risks. Training programs can also educate employees about the dangers of insider threats, fostering a culture of vigilance.
Challenges in Fraud Detection arise from the complexity of modern systems. Data interconnectivity and system silos can facilitate fraud, as demonstrated by a personal encounter with a fraud attack on an Amazon account. This incident highlights the speed at which fraudsters operate and the need for comprehensive security measures.
Cybercrime Report findings indicate a rise in contactless fraud. As contactless payment methods become more popular, fraudsters exploit vulnerabilities in these systems. The phrase "contactless fraud rises" captures the urgency of addressing this issue. Organizations must invest in technologies like bot detection to safeguard against these threats.
Frank McKenna and Mary, experts in fraud prevention, emphasize the importance of behavioral biometrics. By collecting comprehensive behavioral insights, companies can enhance their fraud detection capabilities. This approach involves analyzing user behavior patterns to identify anomalies, providing an additional layer of security.
Strategic Approaches to Fraud Detection
Real-Time Monitoring Systems
Real-time monitoring systems serve as a cornerstone in the fight against fraud. These systems continuously analyze transactions, identifying suspicious activities as they occur. However, implementing such systems presents several challenges.
Implementation Challenges
Organizations face numerous hurdles when deploying real-time monitoring systems. The integration of advanced fraud detection solutions requires significant investment in technology and infrastructure. Additionally, maintaining these systems demands ongoing updates to keep pace with evolving fraud types and prevention methods. Institutions must also address the complexity of managing fraud risk across diverse platforms and channels.
Moreover, the sheer volume of data processed by these systems can overwhelm existing infrastructure. Companies must ensure their systems can handle large-scale data processing without compromising performance. This challenge necessitates a robust IT framework capable of supporting real-time data analysis.
Best Practices for Effectiveness
To maximize the effectiveness of real-time monitoring systems, organizations should adopt several best practices. First, leveraging anti fraud technology, such as AI and machine learning, enhances the accuracy of fraud detection and investigation. These technologies enable systems to learn from historical data, improving their ability to identify potential threats.
Second, institutions should prioritize intelligence sharing among stakeholders. Collaborative efforts between financial entities, law enforcement, and regulatory bodies strengthen the overall defense against fraudsters. By sharing insights and data, organizations can develop more comprehensive strategies for fraud prevention with real-time capabilities.
Finally, regular audits and system evaluations ensure that monitoring systems remain effective. Continuous assessment allows companies to identify weaknesses and implement necessary improvements. This proactive approach helps maintain a robust defense against emerging fraud threats.
Regulatory Compliance
Regulatory compliance plays a crucial role in shaping fraud detection strategies. Adhering to legal requirements not only ensures the effectiveness of fraud prevention measures but also builds trust among customers and partners.
Key Regulations to Consider
Organizations must navigate a complex landscape of regulations to maintain compliance. The revised Payment Services Directive (PSD2) in Europe mandates specific reporting requirements for fraud detection and prevention. Similarly, the Fair Credit Reporting Act (FCRA) in the United States outlines guidelines for handling consumer credit information.
Compliance with these regulations requires institutions to implement stringent data privacy measures. Fraud detection solutions must adhere to stricter standards, ensuring that sensitive information remains secure. Companies like LexisNexis Risk Solutions provide valuable resources for navigating these regulatory challenges.
Impact on Fraud Detection Strategies
Regulatory compliance significantly influences fraud detection strategies. Adhering to legal requirements ensures that organizations can effectively manage fraud risk while maintaining customer trust. Compliance-driven strategies often incorporate advanced fraud technology, such as AI and machine learning, to enhance detection capabilities.
Furthermore, regulations encourage institutions to adopt comprehensive approaches to fraud prevention. By aligning their strategies with legal standards, companies can develop more effective fraud prevention measures. This alignment not only reduces the risk of non-compliance but also strengthens the overall defense against fraud.
Future Trends in Fraud Detection
Increasing Fraud Volume and Types
Fraudsters constantly adapt, leading to an increasing fraud volume and types. The Fraud and Identity landscape continues to evolve, presenting new challenges for organizations. Contactless payment methods, while convenient, have become a target for fraudsters. They exploit vulnerabilities in these systems, resulting in a surge of fraudulent activities. As the Digital Identity Network expands, the complexity of fraud and identity issues grows. Institutions must remain vigilant, continuously updating their strategies to address these threats.
Fraud Volume and Types have diversified, with contactless fraud becoming more prevalent. This trend underscores the need for robust security measures. Organizations must invest in advanced technologies to detect and prevent these activities. AI and machine learning play a crucial role in this process. They analyze large datasets, identifying patterns that indicate potential fraud. By leveraging these technologies, institutions can stay ahead of fraudsters, ensuring the safety of their systems.
Fraud Prevention and Detection Strategies
Cutting-Edge Fraud Prevention Trends focus on proactive measures. AI's predictive capability allows organizations to anticipate new fraud trends. By analyzing vast amounts of data, AI identifies behaviors that suggest fraudulent activity. This approach reduces false positives, enhancing the accuracy of fraud detection systems. Institutions must embrace these technologies to future-proof their fraud prevention strategies.
Transformative Fraud Prevention involves a dual role in fraud detection and prevention. Organizations must not only detect fraudulent activities but also prevent them from occurring. This requires a comprehensive approach, integrating various technologies and strategies. Contactless payment systems, for example, must incorporate advanced security features to protect against fraud. By adopting a holistic approach, institutions can effectively combat the increasing fraud volume.
Future Proofing Fraud Prevention necessitates continuous learning and adaptation. Organizations must stay informed about the latest trends and insights in fraud detection. Regular updates to their systems ensure they remain effective against emerging threats. By fostering a culture of vigilance, institutions can safeguard their assets and maintain customer trust.
Conclusion
Real-time fraud detection continues to evolve, driven by technological advancements and emerging threats. Key trends, such as AI and machine learning, play a pivotal role in enhancing predictive analytics and anomaly detection. The AIM Leaders Council emphasizes the importance of continuous adaptation and learning to stay ahead of fraudsters. Institutions must harness tools that predict and prevent fraud attempts earlier in the payment process. The AIM Research highlights the need for flexible, adaptive security solutions to address the dynamic nature of cyber threats. Professionals should engage with AIM Council Posts and the AIM Leaders Council to remain informed and proactive. By doing so, they can effectively safeguard against fraud and foster a culture of responsibility and trust. Organizations must tailor their strategies to specific needs, ensuring ongoing effectiveness. As fraudsters evolve tactics, real-time systems must adjust algorithms accordingly. This proactive approach ensures prevention strategies remain robust, preparing institutions to meet current and future challenges in fraud detection. Add to cart the latest insights from AIM Research to enhance your organization's fraud prevention capabilities.