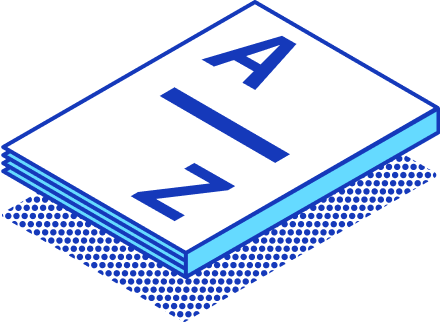
Semantic Layer
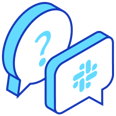
Join StarRocks Community on Slack
Connect on SlackWhat Is a Semantic Layer?
Definition and Overview
Understanding the Concept
A semantic layer serves as a bridge between raw data and business intelligence tools. This layer provides a standardized framework that organizes and abstracts data. Users can access and understand data without dealing with technical complexities. The semantic layer acts like an index in a book, simplifying the search for relevant content. Users interact with data using consistent and well-defined metadata.
Historical Context and Evolution
The concept of a semantic layer has evolved over time. Businesses realized that insights come from understanding data within an organization's context. A semantic layer connects all organizational knowledge assets. This includes structured, unstructured, and semi-structured data. The layer acts as a semantic abstraction, translating data into a structured representation. Knowledge graphs and FAIR principles have further enhanced semantic layers. These tools make data more discoverable and interoperable.
Key Components
Data Abstraction
Data abstraction is a crucial component of a semantic layer. This process hides the complexities of various data sources. Users see data as business objects. Data abstraction ensures a unified view of information. Users can focus on insights rather than technical details.
User Interface
The user interface of a semantic layer plays a vital role. This interface allows users to interact with data easily. Users access data using familiar business terms. The interface enhances data discoverability and accessibility. A well-designed user interface empowers users to make informed decisions.
The Role of Semantic Layer in Data Management
Simplifying Data Complexity
Data Integration
The Semantic Layer plays a crucial role in data integration. Organizations often deal with data from multiple sources. Each source may have its own structure and format. The Semantic Layer acts as a bridge between these diverse data sources. It provides a unified view of information. Users can access data without worrying about its origin or format. This integration simplifies data management. Businesses can focus on deriving insights rather than managing data complexities.
Consistency and Accuracy
Consistency and accuracy are vital in data management. The Semantic Layer ensures that data remains consistent across the organization. It provides standardized definitions for business metrics. Users can rely on accurate data for decision-making. The Semantic Layer reduces discrepancies in data interpretation. A unified framework helps maintain data integrity. Consistent data leads to more reliable insights.
Enhancing Data Accessibility
User Empowerment
The Semantic Layer empowers users by making data more accessible. Users interact with data using familiar business terms. This interaction does not require technical expertise. The Semantic Layer provides an intuitive interface for data exploration. Users can query data and generate reports independently. Empowered users can make informed decisions quickly. This empowerment fosters a culture of data-driven decision-making.
Data Literacy
Data literacy is essential in today's data-driven world. The Semantic Layer enhances data literacy within organizations. Users gain a better understanding of data through simplified interfaces. The Semantic Layer provides clear and consistent data definitions. Users learn to interpret data accurately. Improved data literacy leads to more effective use of data. Organizations benefit from a workforce that understands and utilizes data effectively.
Benefits of Implementing a Semantic Layer
Improved Data Consistency
Unified Data Definitions
A Semantic Layer provides a unified framework for data definitions. Organizations often face challenges with inconsistent data definitions across departments. Each department may use different terms for the same data entity. A Semantic Layer standardizes these definitions. This standardization ensures that everyone speaks the same language when discussing data. Consistent data definitions lead to more accurate analysis and reporting.
Reduced Redundancy
Redundancy in data can cause confusion and inefficiency. A Semantic Layer reduces redundancy by consolidating data from various sources. This consolidation creates a single source of truth for the organization. Users access data without encountering duplicates or conflicting information. Reduced redundancy streamlines data management processes. Organizations benefit from cleaner and more reliable data.
Enhanced Query Performance
Optimized Data Retrieval
The Semantic Layer optimizes data retrieval processes. Users often need to query large datasets for insights. Traditional methods can be slow and resource-intensive. The Semantic Layer improves query performance by abstracting complex data structures. Users retrieve data quickly and efficiently. Optimized data retrieval enhances productivity and decision-making capabilities.
Faster Insights
Faster insights are crucial in today's fast-paced business environment. The Semantic Layer accelerates the process of gaining insights from data. Users interact with data using familiar business terms. This interaction speeds up the analysis process. Faster insights enable organizations to respond quickly to market changes. Timely decisions give businesses a competitive edge.
Challenges Addressed by Semantic Layers
Data Complexity
Handling Diverse Data Sources
Organizations often manage data from various sources. Each source may have unique structures and formats. A semantic layer simplifies this complexity. It acts as an abstraction that bridges raw data storage and user-friendly interfaces. This layer enables seamless integration and exploration of information. Users can query large datasets without needing deep knowledge of data structures. The semantic layer translates data into a structured and understandable representation. This translation makes data more accessible and manageable.
Streamlining Data Processing
Data processing involves multiple steps and can be resource-intensive. A semantic layer streamlines these processes. It provides a unified view of data from disparate systems. Users can explore and query data efficiently. This efficiency reduces the time and effort required for data processing. The semantic layer brings together experimental data, clinical trial outcomes, and real-world evidence. This integration creates a research-friendly view of information. Users benefit from faster and more accurate data processing.
Data Inconsistency
Ensuring Data Integrity
Data integrity is crucial for reliable insights. A semantic layer ensures consistent terminology across all collaborators. This consistency improves data quality. Users can trust the accuracy of their data. The semantic layer defines metrics and data concepts at the entity level. This definition standardizes data usage across the organization. Consistent data leads to more reproducible results in collaborative efforts. Organizations achieve higher data integrity with a semantic layer.
Standardizing Data Formats
Different departments may use varied data formats. These variations can lead to confusion and inefficiency. A semantic layer standardizes data formats across the organization. It provides context and standardized definitions to individual data points. Users interact with data using common business terms. This interaction enhances data discoverability and usability. Standardized data formats improve collaboration and decision-making. Organizations benefit from a universal understanding of enterprise-based knowledge.
Real-World Applications of Semantic Layers
Business Intelligence
Data-Driven Decision Making
Business intelligence relies on data-driven decision making. A Semantic Layer transforms raw data into actionable insights. Users access consistent and accurate data without technical barriers. This layer provides a unified view of information across departments. Decision-makers rely on this structured data to make informed choices. The Semantic Layer enhances the quality of business decisions.
Predictive Analytics
Predictive analytics forecasts future trends and behaviors. A Semantic Layer plays a crucial role in this process. Users analyze historical data to predict outcomes. The layer organizes data for easy access and interpretation. Analysts use familiar business terms to explore data sets. This approach simplifies complex data structures. Predictive models become more accurate and reliable with a Semantic Layer.
Industry Use Cases
Healthcare
The healthcare industry benefits from Semantic Layers. Medical professionals manage vast amounts of patient data. A Semantic Layer integrates diverse data sources seamlessly. Doctors access patient records, lab results, and treatment plans efficiently. This integration improves patient care and outcomes. Healthcare providers make data-driven decisions quickly. The Semantic Layer enhances collaboration among medical teams.
Finance
The finance sector utilizes Semantic Layers for customer analytics. Financial institutions handle complex data structures daily. A Semantic Layer provides a standardized framework for data analysis. Users navigate customer and product information effortlessly. This structure links metrics from various formats in one place. Financial analysts gain insights into customer behavior and market trends. The Semantic Layer supports strategic financial planning and risk management.
Future Implications of Semantic Layers
Strategic Importance
Competitive Advantage
Semantic layers provide a competitive edge in data management. Businesses gain a universal understanding of enterprise knowledge. This understanding comes from standardized definitions and context. Companies use semantic layers to streamline data processes. This efficiency leads to quicker decision-making and better strategies.
Innovation in Data Analytics
Semantic layers drive innovation in data analytics. Organizations explore data with user-friendly interfaces. This exploration fosters creativity and new insights. The abstraction layer bridges raw data and business intelligence tools. Teams analyze data without technical barriers. This ease of access encourages innovative solutions.
Emerging Trends
AI and Machine Learning Integration
AI and machine learning benefit from semantic layers. These technologies require structured and accessible data. Semantic layers provide this structure. Data becomes more discoverable and usable. AI models improve with accurate and consistent data inputs. Machine learning algorithms deliver better predictions and insights.
Cloud-Based Solutions
Cloud-based solutions enhance the use of semantic layers. Organizations store and access data remotely. This flexibility supports scalability and efficiency. Cloud platforms integrate seamlessly with semantic layers. Businesses manage large volumes of data effortlessly. Cloud solutions ensure data remains accessible and secure.
Conclusion
Semantic layers hold immense significance in the realm of data analytics. These layers provide an integrated view of data, which facilitates seamless data-driven decision-making. Semantic layers organize and abstract data, offering a standardized framework that connects various data structures and relationships. This approach enables businesses to derive new insights and optimize data management for capturing business meaning and context. Implementing semantic layers can transform how organizations handle data, leading to more informed decisions. Businesses should consider adopting semantic layers to stay competitive and innovative in the ever-evolving data landscape.