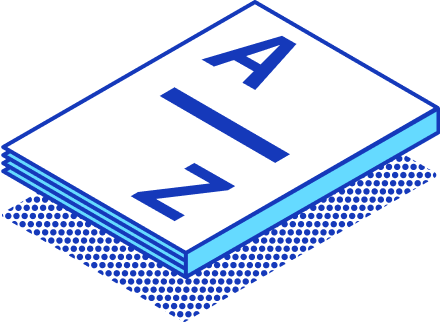
Semantic Search vs Full-Text Search: A Detailed Comparison
Understanding Full-Text Search
Full Text Search plays a crucial role in retrieving information from vast collections of documents. It focuses on matching exact keywords, making it an efficient tool for structured queries. Let's delve into how this search method works and its limitations.
Mechanics of Full-Text Search
How Full-Text Search Works
When you use a generic search engine, it scans through the text of documents to find matches for your query. This process involves indexing, where the search engine creates a list of words found in each document. When you enter a search term, the engine quickly retrieves documents containing those words. This method ensures fast and precise results, especially for straightforward queries.
Common Algorithms Used
Several algorithms enhance the efficiency of Full Text Search. Among them, BM25 and TF-IDF stand out. BM25 ranks documents based on the frequency and distribution of terms, while TF-IDF evaluates the importance of a word in a document relative to a collection. These algorithms help in ranking search results, ensuring that the most relevant documents appear first.
Limitations of Full-Text Search
Challenges with Keyword Matching
Despite its efficiency, Full Text Search faces challenges with keyword matching. It relies heavily on exact matches, which can lead to irrelevant results if the search terms do not precisely match the document's content. This limitation becomes evident when dealing with synonyms or variations in phrasing.
Issues with Context and Relevance
Another significant limitation is its struggle with context and relevance. Full Text Search does not understand the intent behind your query. It treats each word independently, often missing the broader meaning. This can result in retrieving documents that contain the keywords but lack the context you seek. For instance, searching for "Data Integration" might yield results that mention the term but do not address its application or significance.
Exploring Semantic Search
In the realm of search technologies, Exploring Semantic Search reveals a transformative approach that goes beyond mere keyword matching. This method focuses on understanding the intent behind your queries and the context in which they are made. By doing so, it delivers results that are not only relevant but also meaningful.
How Semantic Search Works
Understanding User Intent
Semantic search excels at deciphering the context and meaning behind a search query. It uses advanced technologies like machine learning and AI to match your search intent with semantic meaning. This capability allows the search engine to provide more relevant results by understanding what you truly seek, rather than just the words you type. For instance, when you search for "best Italian restaurant," a good semantic search system considers your location, preferences, and past searches to suggest the most suitable options.
Use of Natural Language Processing
Natural Language Processing (NLP) plays a pivotal role in semantic search. It empowers search engines to comprehend and interpret human language, leading to more precise results. NLP techniques analyze the relationships between words, representing them as vectors in a high-dimensional space. This representation helps the search engine understand the meaning behind words and their connections, enhancing the accuracy of search results. By utilizing user data, NLP-based search extracts precise meanings from your queries, ensuring that the results align with your expectations.
Benefits of Semantic Search
Improved Relevance and Accuracy
Semantic search significantly improves the relevance and accuracy of search results. By understanding the semantic nuances of text, it delivers results that match your intent rather than just the keywords. This approach reduces the time you spend sifting through irrelevant documents, allowing you to find the information you need more efficiently. The integration of semantic enrichment further enhances this process by adding layers of meaning to the data, ensuring that the search results are both comprehensive and precise.
Enhanced User Experience
A great semantic search engine enhances your user experience by providing more intuitive and personalized results. It considers various factors such as context, user behavior, and preferences to tailor the search experience to your needs. This personalization makes the search process smoother and more satisfying, as you receive results that are not only relevant but also aligned with your interests. By focusing on the entities within your queries, semantic search ensures that you receive information that is both accurate and meaningful.
Direct Comparison: Full-Text vs Semantic Search
When deciding between full-text and semantic search, understanding their distinct use cases is crucial. Each method excels in different scenarios, offering unique strengths and challenges.
Use Cases for Each Method
When to Use Full-Text Search
Full-text search shines when you need to find specific keywords within large datasets. This method is ideal for applications like log analysis or legal document retrieval, where exact keyword matches are essential. You can quickly locate documents containing precise terms, making it a reliable choice for structured queries. For instance, if you need to search through a database of medical records to find mentions of a specific drug, full-text search provides fast and accurate results.
Ideal Scenarios for Semantic Search
Semantic search offers a more nuanced approach, understanding the intent and context behind your queries. This method is perfect for complex searches where keyword similarity alone isn't enough. A good semantic search engine can interpret user intent, making it suitable for applications like customer service chatbots or recommendation systems. For example, when searching for "best treatment for headaches," a semantic search engine considers various factors, such as user preferences and past interactions, to provide personalized and relevant suggestions.
Advantages and Challenges
Strengths of Full-Text Search
Full-text search provides speed and precision, especially for straightforward queries. It excels in environments where keyword search simply needs to be fast and efficient. The system quickly indexes and retrieves documents, ensuring you find the information you need without delay. This method is particularly effective for keyword similarity tasks, where exact matches are crucial.
Strengths of Semantic Search
Semantic search offers improved relevance and accuracy by understanding the context and meaning behind queries. It reduces the time spent sifting through irrelevant documents, enhancing the user experience. By leveraging NLP similarity search techniques, semantic search provides results that align with your intent, making it a powerful tool for complex queries. This approach is beneficial in scenarios where understanding the broader context is essential, such as finding the best drug interactions based on patient history.
Limitations and Challenges of Each
While full-text search is efficient, it struggles with context and relevance. It relies heavily on keyword matches, which can lead to irrelevant results if the search terms don't precisely match the document's content. On the other hand, semantic search requires more computational resources and sophisticated algorithms, which can be challenging to implement. However, the benefits of a semantic search engine often outweigh these challenges, offering a more intuitive and personalized search experience.
Hybrid Search Techniques
In the evolving landscape of search technologies, Hybrid Search emerges as a powerful approach. By combining full-text and semantic search, you can achieve a more comprehensive and accurate retrieval of information. This technique leverages the strengths of both methods, offering a balanced solution for diverse search needs.
Combining Full-Text and Semantic Search
Hybrid search integrates the precision of full-text search with the contextual understanding of semantic search. This combination allows you to benefit from the fine-grained control of keyword matching while also capturing the broader meaning behind queries. The result is a search experience that is both efficient and insightful.
Benefits of a Hybrid Approach
-
Enhanced Relevance: By merging full-text and semantic search, hybrid search improves the relevance of results. You can find documents that not only contain the exact keywords but also align with the intent behind your query.
-
Improved Accuracy: Hybrid search techniques enhance accuracy by considering both keyword matches and semantic similarity. This dual approach ensures that you receive results that are both precise and contextually appropriate.
-
Broader Coverage: With hybrid search, you can access a wider range of information. The integration of vector semantic similarity search allows you to explore connections between concepts, providing a more holistic view of the data.
-
Personalized Experience: By understanding user intent and preferences, hybrid search delivers a more personalized search experience. You can receive recommendations and insights tailored to your specific needs.
Examples of Hybrid Search Implementations
-
Pinecone: This platform supports hybrid search by combining sparse and dense vectors. You can perform searches that integrate semantic and keyword elements, resulting in more relevant outcomes.
-
Similarity Search Models: These models utilize hybrid search to enhance the retrieval process. By incorporating both full-text and semantic similarity search, they offer a robust solution for complex queries.
-
GSK's Drug Development Pipeline: In the pharmaceutical industry, hybrid search plays a crucial role. By integrating semantic similarity search, companies like GSK can efficiently navigate vast datasets, identifying potential drug interactions and accelerating the development process.
-
Erectile Dysfunction Research: Researchers use hybrid search to explore the relationship between proteins and treatments. By combining keyword and semantic search, they can uncover insights into the role of glycogen synthase and phosphodiesterase class proteins in erectile dysfunction.
In summary, hybrid search represents a significant advancement in search technology. By unifying full-text and semantic search, it offers a versatile and effective solution for a wide range of applications. Whether you’re exploring NLP similarity search techniques or implementing a similarity search product, hybrid search provides the tools you need to succeed.
Conclusion
In your journey to understand search technologies, you have explored the key differences and similarities between Lexical Search and Semantic search. Lexical Search focuses on exact keyword matches, while Semantic search delves into understanding intent and context. When choosing the appropriate search method, consider your specific needs. Lexical search suits structured queries, whereas Semantic search excels in complex scenarios. As search technologies evolve, Hybrid Search emerges as a promising solution, combining the strengths of both methods. This approach enhances Similarity and relevance, offering a more comprehensive search experience. Ethical considerations also play a role, as discussed in various articles and papers. Embrace these advancements and stay informed about future developments.