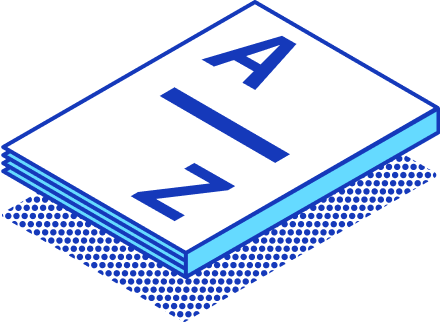
Semantic Similarity
What Is Semantic Similarity
Definition and Explanation
Understanding the Concept
Semantic Similarity measures how meanings align between words or phrases. This concept goes beyond simple word matching. Semantic Similarity focuses on the meaning behind the text. You can see how two items relate based on their semantic content. This approach provides a deeper understanding of language.
Differences from Traditional Similarity Measures
Traditional similarity measures rely on surface features. These methods look at word order or spelling. Semantic Similarity, however, examines meaning. This difference allows for more accurate analysis. You gain insights by focusing on context and relationships.
Historical Context
Evolution of Semantic Similarity
Semantic Similarity has evolved over time. Early methods focused on basic word matching. Researchers then explored deeper connections. Advances in technology enabled more complex analyses. Semantic Similarity now plays a vital role in many fields.
Key Milestones in Development
Several milestones mark the development of Semantic Similarity. Researchers introduced new algorithms to improve accuracy. Machine learning enhanced the ability to analyze meaning. These advancements transformed how you understand language. Semantic Similarity continues to grow and impact various industries.
Importance of Semantic Similarity
Enhancing Data Analysis
Improving Accuracy and Relevance
Semantic Similarity enhances data analysis by focusing on meaning. Traditional methods rely on word order or spelling. Semantic Similarity examines the semantic content. This approach improves accuracy in understanding text. Researchers gain insights by analyzing meanings rather than surface features. Semantic Similarity provides relevant results in various fields.
Facilitating Better Decision-Making
Semantic Similarity aids decision-making by offering deeper insights. Analyzing semantic relationships helps identify patterns. Businesses use these insights to make informed choices. Semantic Similarity supports strategic planning and problem-solving. Understanding meanings leads to better outcomes.
Impact on Technology
Transforming Search Engines
Semantic Similarity transforms search engines by improving result relevance. Algorithms analyze meanings instead of keywords. Users find more accurate information quickly. Search engines understand user intent through semantic analysis. This transformation enhances user experience and satisfaction.
Advancing Artificial Intelligence
Semantic Similarity advances artificial intelligence by enhancing understanding. AI systems interpret language more effectively. Machine learning models improve through semantic insights. AI applications become more intuitive and responsive. Semantic Similarity drives innovation in AI technologies.
Applications in Natural Language Processing
Text Analysis and Understanding
Semantic Similarity plays a crucial role in text analysis and understanding. This metric helps machines comprehend language by estimating how closely meanings align between different texts. Researchers and developers use Semantic Similarity to enhance various natural language processing tasks.
Sentiment Analysis
Sentiment analysis benefits greatly from Semantic Similarity. This process involves determining the emotional tone behind a body of text. Semantic Similarity allows systems to understand the context and nuances of language. Machines can identify whether a statement is positive, negative, or neutral. This capability improves the accuracy of sentiment analysis.
Machine Translation
Machine translation relies on Semantic Similarity to provide accurate translations. Traditional methods often struggle with idiomatic expressions or context-specific meanings. Semantic Similarity helps machines grasp the intended meaning behind phrases. This understanding leads to more precise translations that preserve the original message. Users experience better communication across languages.
Chatbots and Virtual Assistants
Semantic Similarity enhances the functionality of chatbots and virtual assistants. These tools interact with users by understanding and responding to queries. Semantic Similarity improves the quality of these interactions.
Enhancing User Interaction
User interaction becomes more intuitive with Semantic Similarity. Chatbots analyze user input to determine intent. Semantic Similarity helps identify the most relevant responses. Users enjoy seamless conversations that feel natural and engaging. This improvement increases user satisfaction and trust in technology.
Improving Response Accuracy
Response accuracy is vital for effective communication. Semantic Similarity ensures that chatbots and virtual assistants provide correct answers. By understanding the semantic content of queries, these systems deliver precise information. Users receive reliable assistance tailored to their needs. This accuracy enhances the overall user experience.
Scientific Research Findings:
-
Semantic text similarity estimation in NLP applications highlights the importance of Semantic Similarity in text processing tasks.
-
Importance of Semantic Similarity in NLP emphasizes its role in understanding language processing by machines.
Applications in Biomedical Informatics
Drug Discovery and Development
Identifying Similar Compounds
Researchers use semantic similarity to identify similar compounds in drug discovery. This process involves comparing chemical structures and properties. Semantic similarity helps find compounds with potential therapeutic effects. Researchers accelerate the development of new drugs by identifying these similarities.
Predicting Drug Interactions
Semantic similarity aids in predicting drug interactions. Scientists analyze the relationships between different drugs. This analysis helps foresee potential adverse effects. Semantic similarity provides valuable insights into how drugs interact at a molecular level. Researchers use this information to improve patient safety and treatment efficacy.
Genomic Research
Analyzing Genetic Data
Genomic research benefits from semantic similarity when analyzing genetic data. Scientists compare genetic sequences to understand functional similarities. Semantic similarity helps identify genes with related functions. Researchers gain insights into genetic variations and their implications for health.
Understanding Disease Mechanisms
Semantic similarity plays a crucial role in understanding disease mechanisms. Researchers study the relationships between genes, proteins, and diseases. This analysis uncovers the underlying causes of various conditions. Semantic similarity helps identify potential targets for therapeutic interventions. Scientists use this knowledge to develop effective treatments and improve patient outcomes.
Applications in Geoinformatics
Spatial Data Analysis
Mapping and Visualization
Semantic similarity enhances mapping and visualization. Geospatial texts often lack non-spatial relationship expressions. Semantic analysis fills this gap. Researchers use semantic methods to measure spatial similarity. These methods improve map accuracy and detail. Users gain clearer insights into geographic data.
Environmental Monitoring
Environmental monitoring benefits from semantic similarity. Scientists analyze spatial data for environmental changes. Semantic tools identify patterns and trends. This analysis aids in tracking climate change and pollution. Decision-makers use these insights for effective environmental policies.
Urban Planning
Infrastructure Development
Semantic similarity supports infrastructure development. Planners assess spatial relationships and needs. Semantic analysis helps identify optimal locations for new projects. This approach ensures efficient resource allocation. Communities experience improved infrastructure and services.
Resource Management
Resource management relies on semantic similarity. Urban planners evaluate land use and resource distribution. Semantic tools provide insights into efficient management strategies. This analysis helps balance development with sustainability. Cities achieve better resource utilization and planning outcomes.
Scientific Research Findings:
-
Research on Semantic Similarity in Geoinformatics highlights the role of semantic methods in spatial data analysis.
-
The Semantics of Similarity in Geographic Information Retrieval explores how semantic similarity improves geographic information systems.
Future Potential and Implications
Emerging Trends
Integration with Machine Learning
Semantic similarity integrates with machine learning to enhance data analysis. Machine learning models use semantic insights to improve accuracy. Algorithms learn from semantic relationships to provide better predictions. This integration transforms how systems understand language.
Expansion into New Domains
Semantic similarity expands into new domains beyond traditional fields. Industries like finance and education explore semantic tools. Businesses use semantic analysis for customer insights. Educational platforms enhance learning experiences through semantic understanding. This expansion opens new opportunities for innovation.
Ethical Considerations
Privacy Concerns
Privacy concerns arise with the use of semantic similarity. Data analysis involves sensitive information. Organizations must ensure data protection. Users demand transparency in how data is used. Privacy regulations guide ethical practices in semantic analysis.
Bias and Fairness
Bias and fairness present challenges in semantic similarity. Algorithms may reflect existing biases in data. Developers work to create fair models. Semantic tools must provide unbiased results. Ensuring fairness enhances trust in technology. Addressing bias promotes ethical use of semantic methods.
Conclusion
Semantic similarity transforms how you understand language and concepts. This metric measures the likeness of meanings, enhancing fields like natural language processing and biomedicine. Semantic similarity improves data analysis and decision-making. Researchers use it to predict behavior and analyze biomedical data. The potential for innovation is vast. Semantic similarity continues to expand into new domains. You can explore its applications in technology and science. Further research will unlock more possibilities. Embrace semantic similarity as a tool for understanding and discovery.
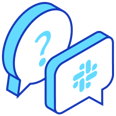
Join StarRocks Community on Slack
Connect on Slack