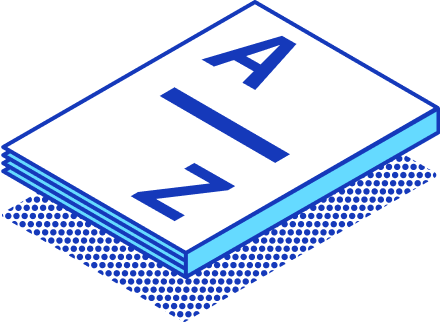
Telecom Analytics
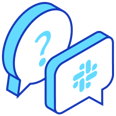
Join StarRocks Community on Slack
Connect on SlackWhat Is Telecom Analytics
Telecom Analytics involves the systematic analysis of large volumes of data generated within the telecommunications sector. This process helps communication service providers (CSPs) gather insights that drive strategic decision-making. Analytics in this field focuses on optimizing network performance, enhancing customer experience, and improving operational efficiency. The use of descriptive analytics in the telecom industry aids in understanding patterns and trends, which are crucial for maintaining competitive advantage.
Key Components of Telecom Analytics
Telecom Analytics comprises several key components that work together to extract valuable insights from data. These components include:
-
Data Collection: Gathering data from various sources such as call records, network logs, and customer interactions.
-
Data Processing: Cleaning and organizing data to ensure accuracy and consistency.
-
Data Analysis: Applying analytical techniques to identify patterns, trends, and correlations.
-
Data Visualization: Presenting data insights in an easily understandable format using graphs and charts.
-
Predictive Modeling: Using historical data to forecast future trends and behaviors.
Historical Context
Evolution of Telecom Analytics
The evolution of Telecom Analytics has been marked by significant advancements in technology and methodology. Initially, telecom companies relied on basic data collection and reporting tools. Over time, the integration of advanced analytics techniques such as machine learning and artificial intelligence transformed the landscape. These innovations enabled more sophisticated analysis and prediction capabilities, allowing telecom operators to make faster and more informed decisions.
Milestones in the Industry
Several milestones have shaped the development of Telecom Analytics. The publication of the Descriptive Analytics Guidebook by TM Forum offered best practices and tools for big data projects. This guidebook played a pivotal role in standardizing analytics processes across the industry. Additionally, the application of predictive analytics in telecom companies provided valuable insights for data-driven product development and marketing intelligence. These milestones underscore the importance of analytics in driving innovation and growth within the telecom sector.
Benefits of Telecom Analytics
Operational Efficiency
Cost Reduction
Telecom companies achieve cost reduction by utilizing data analytics to streamline operations. Analyzing telecom data helps identify inefficiencies and optimize resource allocation. Predictive analytics anticipates network failures, reducing maintenance costs. Data-driven pricing strategies ensure competitive service pricing, attracting and retaining customers. These strategies lead to increased sales volume and reduced operational expenses.
Process Optimization
Process optimization in the telecom industry relies on effective data analytics. Telecom operators use telecom data to enhance network performance and improve service delivery. Analytics tools identify traffic patterns and optimize network usage. This approach ensures efficient resource deployment and minimizes service disruptions. Prescriptive analytics recommends specific actions to enhance operational processes, leading to improved efficiency.
Customer Experience Enhancement
Personalization
Personalization plays a crucial role in enhancing customer experience within the telecom sector. Leveraging customer data allows telecom operators to tailor services to individual needs. Data analytics enables targeted marketing campaigns, increasing conversion rates. Personalized retention strategies address at-risk customers, preventing churn. These efforts result in higher customer satisfaction and loyalty.
Customer Retention
Customer retention remains a priority for telecom companies. Analyzing customer behaviors through big data helps prevent churn. Immediate resolution of satisfaction issues reduces customer attrition. Prescriptive analytics proposes customized actions based on customer data, enhancing loyalty. Telecom operators use these insights to maintain a competitive edge and ensure long-term success.
Types of Telecom Analytics
Descriptive Analytics
Descriptive analytics plays a pivotal role in the telecom industry by summarizing historical data to provide insights into past events. This type of analytics involves examining vast amounts of telecom data to identify patterns and trends. Descriptive analytics enables telecom operators to understand what has happened in their networks, offering a clear picture of past performance.
Data Aggregation
Data aggregation is a fundamental component of descriptive analytics. Telecom data analytics involves collecting and compiling data from various sources, such as call records, network logs, and customer interactions. This process allows telecom companies to create comprehensive datasets that form the basis for further analysis. Aggregated data provides a holistic view of network operations, facilitating informed decision-making.
Pattern Recognition
Pattern recognition is another crucial aspect of descriptive analytics. By analyzing aggregated telecom data, telecom data analytics enables the identification of recurring patterns and anomalies. This insight helps telecom operators understand customer behavior, network usage, and service quality. Recognizing patterns in historical data allows companies to anticipate future needs and optimize resources effectively.
Predictive Analytics
Predictive analytics uses statistical models and machine learning algorithms to forecast future events and trends based on historical data and patterns. This type of analytics involves leveraging advanced data analytics techniques to predict potential outcomes and prepare for them proactively.
Forecasting Trends
Forecasting trends is a key application of predictive analytics in the telecom sector. Telecom data analytics offers the ability to analyze historical data and predict future demand for services. This foresight helps telecom operators plan network expansions, allocate resources efficiently, and meet customer expectations. Accurate trend forecasting ensures that companies stay ahead in a competitive market.
Risk Management
Risk management is another significant benefit of predictive analytics. By analyzing past incidents and identifying risk factors, telecom data analytics enables companies to anticipate potential challenges and mitigate them before they escalate. Predictive models help in assessing the likelihood of network failures, security breaches, or customer churn. Proactive risk management enhances operational stability and customer satisfaction.
Prescriptive Analytics
Prescriptive analytics empowers organizations to make informed decisions and take proactive measures for optimization and enhancement. This type of analytics involves using historical data, predictive models, and optimization algorithms to recommend specific actions.
Decision-Making Support
Decision-making support is a primary function of prescriptive analytics. Telecom data analytics involves providing actionable insights that guide strategic choices. By analyzing various scenarios and their potential outcomes, prescriptive analytics enables telecom operators to select the best course of action. This approach ensures that decisions align with business objectives and maximize efficiency.
Strategy Formulation
Strategy formulation benefits significantly from prescriptive analytics. Telecom data analytics enables companies to develop data-driven strategies that enhance competitiveness. By considering multiple variables and constraints, prescriptive analytics offers recommendations for optimizing network performance, improving customer experience, and increasing profitability. Well-formulated strategies position telecom operators for long-term success in a dynamic industry.
Use Cases of Telecom Analytics
Network Optimization
Telecom operators rely on Analytics to optimize network performance. Analyzing vast amounts of data helps identify areas where the network can improve. Operators focus on enhancing efficiency and ensuring optimal performance.
Traffic Management
Traffic management is crucial for maintaining seamless network operations. Telecom companies use Analytics to monitor and manage data flow across the network. Analyzing traffic patterns allows operators to allocate resources effectively. This approach prevents congestion and ensures smooth data transmission.
-
Data Collection: Operators gather data from various sources, including call records and network logs.
-
Data Analysis: Analyzing this data helps identify peak usage times and potential bottlenecks.
-
Resource Allocation: Based on insights, operators adjust network capacity to handle traffic efficiently.
Fault Detection
Fault detection is essential for minimizing service disruptions. Telecom companies employ Analytics to detect and address network issues promptly. Analyzing historical data helps predict potential failures. This proactive approach reduces downtime and enhances customer satisfaction.
-
Predictive Modeling: Historical data is used to forecast potential network failures.
-
Real-Time Monitoring: Continuous monitoring allows for immediate identification of faults.
-
Preventive Measures: Based on predictions, operators implement measures to prevent issues.
Revenue Assurance
Revenue assurance ensures financial stability for telecom companies. Analytics plays a vital role in identifying and mitigating revenue leakage. By analyzing billing data and customer interactions, operators enhance profitability and reduce churn.
Fraud Detection
Fraud detection is critical for safeguarding revenue. Telecom companies use Analytics to identify fraudulent activities. Analyzing call patterns and customer behavior helps detect anomalies. This approach prevents financial losses and protects customer data.
-
Pattern Recognition: Analyzing call data reveals unusual patterns indicative of fraud.
-
Alert Systems: Automated alerts notify operators of suspicious activities.
-
Investigation and Resolution: Based on alerts, operators investigate and resolve issues promptly.
Billing Accuracy
Billing accuracy is essential for maintaining customer trust. Telecom companies leverage Analytics to ensure precise billing. Analyzing billing data helps identify discrepancies and errors. Accurate billing reduces churn and enhances customer satisfaction.
-
Data Verification: Analyzing billing data ensures consistency and accuracy.
-
Error Identification: Operators identify and rectify billing errors based on analysis.
-
Customer Communication: Clear communication with customers builds trust and loyalty.
Challenges in Implementing Telecom Analytics
Telecom analytics offers numerous benefits, but implementing these solutions presents several challenges. Understanding these challenges is crucial for telecom operators aiming to leverage data analytics effectively.
Data Privacy Concerns
Regulatory Compliance
Telecom companies must adhere to strict regulations to protect customer data. The Electronic Communications Privacy Act (ECPA) and other federal laws in the U.S. mandate the protection of electronic communications. These laws make it illegal to intercept or access communications without consent. Telecom operators need robust systems to ensure compliance with these regulations. Monitoring key performance indicators related to regulatory compliance helps identify potential gaps. Regular audits and updates to privacy policies are essential to maintain compliance.
Ethical Considerations
Ethical considerations play a significant role in telecom analytics. Companies must balance data collection with respect for customer privacy. Implementing best practices to protect personal information is crucial. Transparency in data usage builds trust with customers. Telecom operators should communicate clearly about how data is collected, stored, and used. Ethical data handling not only ensures compliance but also enhances customer satisfaction and loyalty.
Technical Barriers
Integration Issues
Integration issues pose a significant challenge in telecom analytics implementation. Telecom networks generate vast amounts of data from various sources. Integrating this data into a unified analytics platform requires advanced technical expertise. Compatibility between different systems and software can be problematic. Telecom operators need skilled professionals to manage these integrations effectively. Diagnostic analytics tools can help identify and resolve integration issues, ensuring seamless data flow across systems.
Skill Gaps
Skill gaps present another hurdle in the effective implementation of telecom analytics. The demand for skilled professionals, such as a technical content writer, who can interpret complex data and provide actionable insights is high. Many telecom companies struggle to find qualified personnel with expertise in data analytics and machine learning. Investing in training programs and hiring experienced professionals can bridge these skill gaps. Continuous learning and development initiatives ensure that staff remain updated with the latest analytical techniques and technologies.
Future Trends in Telecom Analytics
AI and Machine Learning Integration
AI and machine learning have become integral to telecom analytics. These technologies enhance the ability to process vast amounts of data efficiently. AI-Powered Analytics tools help telecom companies optimize operations and improve customer experiences.
Automation
Automation plays a crucial role in streamlining telecom processes. AI tools automate routine tasks, reducing human error and increasing efficiency. Automated systems monitor network performance and detect anomalies in real-time. This proactive approach ensures seamless service delivery and minimizes disruptions.
Advanced Predictive Models
Advanced predictive models enable telecom operators to anticipate future trends and challenges. AI-Powered Analytics uses historical data to forecast customer behaviors and network demands. Predictive models assist in capacity planning and resource allocation. Telecom companies use these insights to stay competitive and meet customer expectations.
Real-Time Analytics
Real-time analytics provides immediate insights into network performance and customer interactions. This capability allows telecom operators to make informed decisions quickly. Real-time data analysis enhances responsiveness and adaptability in dynamic environments.
Instant Insights
Instant insights from real-time analytics empower telecom companies to address issues promptly. Operators can identify and resolve network bottlenecks before they impact customers. Immediate access to data supports efficient troubleshooting and enhances service quality.
Dynamic Decision-Making
Dynamic decision-making relies on real-time data to adapt strategies swiftly. Telecom operators use real-time analytics to adjust marketing campaigns and service offerings. This agility ensures alignment with market trends and customer preferences. Amani Undru emphasizes the importance of real-time analytics in maintaining a competitive edge.
Conclusion
Telecom analytics plays a pivotal role in driving business success. Companies optimize operations and visualize data through real-time dashboards. The adoption of data-driven strategies ensures competitive advantage. Telecom analytics empowers businesses to gain insights, generate profits, and stay ahead of competitors. The evolving landscape of telecom analytics offers opportunities for strategic decision-making and operational efficiency. Embracing these advancements enhances customer experience and satisfaction. Businesses should harness the power of telecom analytics to thrive in a dynamic industry.