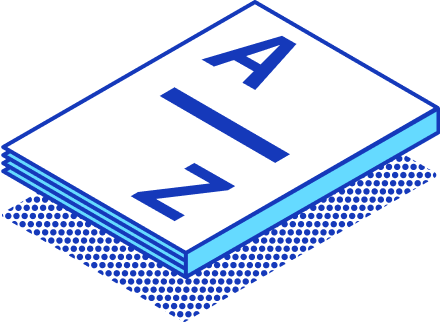
Advanced Analytics
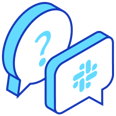
Join StarRocks Community on Slack
Connect on SlackWhat Is Advanced Analytics?
Definition and Overview
Understanding the Basics
Advanced analytics refers to the use of sophisticated techniques and tools to analyze data. These methods go beyond traditional business intelligence. Organizations employ advanced analytics to gain deeper insights and make accurate predictions. This approach involves machine learning, predictive modeling, and other complex processes. Advanced analytics helps businesses transform raw data into actionable strategies.
Differentiating from Traditional Analytics
Traditional analytics relies on a team of IT analysts. These analysts manually examine data, develop theories, test insights, and report findings. In contrast, advanced analytics automates these processes. Advanced analytics enables real-time forecasting, predictive modeling, and visualizations. This automation allows business users to access and utilize data directly. Advanced analytics offers a more dynamic and efficient approach compared to traditional methods.
Key Components
Data Collection and Processing
Data collection forms the foundation of advanced analytics. Organizations gather vast amounts of data from various sources. This data includes structured and unstructured information. Advanced analytics requires efficient data processing. Techniques like data cleaning, integration, and transformation prepare data for analysis. Proper data management ensures accuracy and reliability in advanced analytics.
Analytical Techniques and Tools
Advanced analytics employs a range of techniques and tools. Machine learning algorithms play a crucial role in analyzing data. These algorithms identify patterns and trends within datasets. Predictive modeling forecasts future outcomes based on historical data. Visualization tools present data insights in an understandable format. Sisense, a leading platform, offers comprehensive solutions for advanced analytics. Sisense for free provides users with powerful tools to explore data and gain insights.
Types of Advanced Analytics
Descriptive Analytics
Techniques and Applications
Descriptive analytics focuses on summarizing past data to understand trends and patterns. Businesses use descriptive analytics to analyze historical data and generate reports. Techniques include data aggregation, data mining, and data visualization. These methods help organizations identify patterns in sales data, customer behavior, and operational performance. For example, retailers use descriptive analytics to analyze sales data and understand customer preferences. This analysis assists in inventory management and marketing strategies.
Benefits and Limitations
Descriptive analytics provides a clear view of past performance. Organizations gain insights into what happened and why it happened. This understanding helps in making informed business decisions. However, descriptive analytics does not predict future outcomes. The focus remains on historical data without offering predictive insights. Businesses must combine descriptive analytics with other advanced techniques for comprehensive analysis.
Predictive Analytics
Techniques and Applications
Predictive analytics uses statistical models and machine learning algorithms to predict future outcomes. Organizations apply predictive analytics to forecast sales, customer behavior, and market trends. Techniques include regression analysis, decision trees, and neural networks. These methods help businesses predict customer needs and optimize operations.
Benefits and Limitations
Predictive analytics offers significant advantages by forecasting future trends. Businesses can anticipate customer demands and adjust strategies accordingly. This proactive approach improves decision-making and operational efficiency. However, predictive analytics relies heavily on data quality and model accuracy. Inaccurate data or flawed models can lead to incorrect predictions. Organizations must ensure data integrity and model validation for effective predictive analytics.
Prescriptive Analytics
Techniques and Applications
Prescriptive analytics goes beyond prediction by recommending actions based on data analysis. This type of advanced analytics uses optimization and simulation techniques. Businesses apply prescriptive analytics to determine the best course of action in various scenarios. Techniques include linear programming, Monte Carlo simulation, and heuristic algorithms. These methods help organizations make data-driven decisions in complex situations. For example, supply chain managers use prescriptive analytics to optimize inventory levels and reduce costs.
Benefits and Limitations
Prescriptive analytics provides actionable recommendations for decision-making. Organizations benefit from improved efficiency and reduced operational costs. This approach enables businesses to explore "what-if" scenarios and evaluate potential outcomes. However, prescriptive analytics requires sophisticated tools and expertise. Implementing these techniques can be resource-intensive and complex. Companies must invest in advanced analytics capabilities to fully leverage prescriptive analytics.
Technologies Used in Advanced Analytics
Machine Learning
Algorithms and Models
Machine learning plays a pivotal role in advanced analytics. Organizations use machine learning to process large volumes of data efficiently. Machine learning algorithms learn from data patterns and make predictions. These algorithms include decision trees, neural networks, and support vector machines. Each algorithm has unique strengths for specific tasks. For example, neural networks excel in image recognition tasks. Decision trees are useful for classification problems. Machine learning models adapt and improve over time. This adaptability enhances the accuracy of predictions. Advanced analytics tools leverage machine learning to provide insights.
Use Cases in Various Industries
Various industries benefit from machine learning in advanced analytics. In healthcare, machine learning improves patient diagnosis accuracy. Financial institutions use machine learning for fraud detection. Retailers analyze customer behavior to personalize shopping experiences. Manufacturing companies optimize production processes with predictive maintenance. Machine learning models identify patterns in complex datasets. These patterns reveal valuable insights for decision-making. Big data analytics combined with machine learning transforms industries. The speed and scale of data processing increase significantly.
Artificial Intelligence
Role in Advanced Analytics
Artificial intelligence enhances advanced analytics by automating tasks. AI processes unstructured data and extracts meaningful insights. AI-driven analytics accelerates the speed-to-insights process. Organizations rely on AI to handle complex data analysis tasks. AI models predict future trends and recommend actions. Artificial intelligence integrates seamlessly with machine learning. This integration creates powerful advanced analytics solutions. AI enables organizations to become more data-driven. Advanced analytics software incorporates AI for improved performance.
Examples of AI-driven Analytics
AI-driven analytics finds applications across various sectors. In finance, AI predicts stock market trends and optimizes portfolios. Healthcare providers use AI for personalized treatment plans. Retailers implement AI to enhance customer engagement strategies. AI models simulate different scenarios for supply chain optimization. AI identifies potential risks and suggests mitigation strategies. Advanced analytics tools utilize AI for real-time data analysis. Artificial intelligence transforms raw data into actionable insights. Businesses gain a competitive edge through AI-driven analytics.
Benefits of Advanced Analytics
Enhanced Decision Making
Data-Driven Insights
Advanced analytics offers a powerful toolset for organizations seeking to enhance decision-making processes. Access to data significantly improves the ability to make informed decisions. Business leaders recognize the value of data-driven insights. A survey revealed that 80% of business leaders believe access to data enhances decision-making capabilities. Organizations utilize analytics to transform raw data into actionable insights. These insights guide strategic planning and operational improvements. Advanced analytics on AWS provides businesses with sophisticated tools to analyze vast datasets. AWS enables organizations to uncover hidden patterns and trends within their data.
Real-Time Analysis
Real-time analysis is a critical component of advanced analytics. Organizations require timely insights to respond swiftly to changing market conditions. Advanced analytics offers real-time data processing capabilities. Businesses can monitor operations and make adjustments as needed. Real-time analysis empowers organizations to stay agile and competitive. AWS supports real-time data capture and analysis through its IoT services. These services connect billions of devices, providing continuous data streams. Organizations leverage AWS to gain immediate insights and drive operational efficiency.
Competitive Advantage
Market Trends and Predictions
Advanced analytics offers a competitive edge by enabling organizations to anticipate market trends. Businesses use analytics to forecast future developments and adapt strategies accordingly. Predictive modeling plays a crucial role in identifying emerging trends. Organizations rely on AWS to perform complex analyses and generate accurate predictions. Advanced analytics on AWS helps businesses stay ahead of competitors. Companies can proactively address market shifts and capitalize on new opportunities.
Customer Behavior Analysis
Understanding customer behavior is essential for maintaining a competitive advantage. Advanced analytics provides valuable insights into customer preferences and buying patterns. Organizations analyze customer data to personalize experiences and improve satisfaction. AWS offers robust analytics tools for customer behavior analysis. Businesses use these tools to segment customers and tailor marketing efforts. Advanced analytics offers a deeper understanding of customer needs and expectations. Companies can enhance customer engagement and loyalty through targeted strategies.
Techniques and Tools for Implementing Advanced Analytics
Data Mining
Methods and Processes
Data mining serves as a crucial component in advanced analytics techniques. Organizations employ data mining to extract valuable insights from large datasets. The process involves several key steps, including data collection, cleaning, and transformation. Data mining identifies patterns and relationships within the data, providing a foundation for further analysis. Techniques such as clustering, classification, and association rule learning are commonly used in data mining. These methods enable businesses to uncover hidden trends and make informed decisions.
Tools and Software
Various analytics tools facilitate data mining processes. Apache Spark and Hadoop are popular platforms for handling big data analytics. These tools support real-time streaming and data processing, making them ideal for large-scale data mining tasks. Alteryx offers user-friendly data analytics software that simplifies data mining for end-users. Tableau provides robust data visualization options, allowing users to present mined data effectively. These tools empower organizations to perform comprehensive data mining and gain actionable insights.
Statistical Analysis
Techniques and Applications
Statistical analysis plays a vital role in advanced analytics. Organizations use statistical methods to interpret data and draw meaningful conclusions. Techniques such as regression analysis, hypothesis testing, and variance analysis are fundamental to statistical analysis. These methods help businesses understand relationships between variables and predict future outcomes. Statistical models, including linear and logistic regression, provide a framework for analyzing complex data sets. Organizations apply statistical analysis in various fields, such as finance, healthcare, and marketing, to enhance decision-making.
Tools and Software
Several analytics tools support statistical analysis. R and Python are widely used programming languages for statistical computing. These languages offer extensive libraries for performing statistical analysis and building statistical models. SAS provides comprehensive software solutions for statistical analysis, catering to diverse industry needs. Apache Kafka and Cloudera Data Platform offer data ingestion and cleansing capabilities, ensuring accurate data for statistical analysis. These tools enable organizations to conduct thorough statistical analysis and derive valuable insights.
Evaluating and Implementing Advanced Analytics Solutions
Steps for Successful Implementation
Planning and Strategy
Organizations must begin with a clear plan when implementing advanced analytics solutions. A comprehensive strategy outlines the objectives and goals of the analytics initiative. Data scientists play a crucial role in defining these objectives. Businesses need to assess their current data capabilities. Understanding whether the organization is at a descriptive, predictive, or prescriptive analytics stage is essential. This assessment helps in setting realistic goals and expectations. The planning phase should also include resource allocation. Identifying the necessary tools and services is vital for success. Advanced analytics requirements must align with the organization's overall business strategy.
Execution and Monitoring
Execution involves putting the plan into action. Data scientists must ensure that data collection and processing meet the advanced analytics requirements. The advanced analytics process requires continuous monitoring. Organizations need to track progress and make adjustments as needed. Regular evaluations help in identifying any issues or bottlenecks. Monitoring ensures that the analytics initiative remains on track. Businesses must focus on speed-to-insights and insights-to-action. Quick access to insights allows organizations to respond swiftly to changes. Effective execution and monitoring lead to successful implementation of advanced analytics solutions.
Evaluating Tools and Vendors
Criteria for Selection
Evaluating advanced analytics tools and vendors requires careful consideration. Organizations must identify criteria that align with their specific needs. Data scientists should assess the scalability and flexibility of the tools. Compatibility with existing systems is another critical factor. The tools must support the organization's data volume and complexity. Evaluating the ease of use and user interface is essential. Businesses should consider the level of support and training offered by vendors. Cost-effectiveness plays a significant role in the decision-making process. Organizations must ensure that the selected tools meet their advanced analytics requirements.
Case Studies and Examples
Case studies provide valuable insights into the effectiveness of analytics tools and services. Organizations can learn from real-world examples of successful implementations. Data scientists can analyze how other businesses have leveraged advanced analytics solutions. These examples highlight best practices and potential challenges. Case studies demonstrate the impact of analytics on business performance. Organizations can use these insights to refine their strategies. Evaluating advanced analytics tools becomes more informed through case studies. Businesses gain confidence in their choices by understanding proven successes.
Conclusion
Advanced analytics has transformed the way businesses operate. Organizations now leverage analytics for forecasting, modeling, and visualizations. The integration of machine learning and AI enhances predictive capabilities. Businesses gain a competitive edge by adopting these advanced techniques. The demand for skilled talent in analytics continues to rise. Future trends indicate further growth in this field. Businesses should embrace analytics to stay ahead in the market. Real-time insights from analytics streamline operations and improve decision-making. Advanced analytics remains crucial for business success.