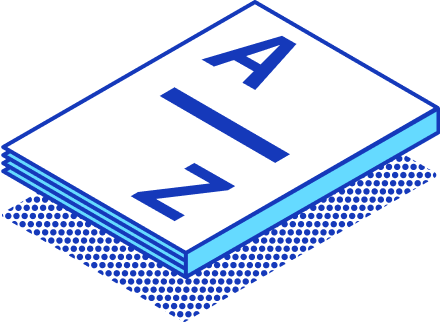
Data Accuracy vs Integrity: Avoid Common Pitfalls
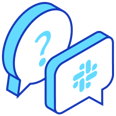
Join StarRocks Community on Slack
Connect on Slack- Understanding Data Accuracy
- Definition of Data Accuracy
- Importance of Data Accuracy
- Understanding Data Integrity
- Definition of Data Integrity
- Role of Data Integrity in Reliable Systems
- Key Differences Between Data Accuracy and Data Integrity
- Comparison of Characteristics
- Interdependencies
- Common Pitfalls and Strategies to Avoid Them
- Common Mistakes in Data Accuracy
- Common Mistakes in Data Integrity
- Strategies for Avoidance
- Conclusion
Understanding Data Accuracy
Definition of Data Accuracy
Data accuracy refers to the precision and correctness of data. It ensures that the information you rely on is free from errors and accurately represents real-world entities. When you think about data accuracy, consider it as a measure of how closely your data aligns with the truth. This alignment is crucial because it forms the foundation for any analysis or decision-making process. Without accurate data, you risk basing your conclusions on flawed information.
Importance of Data Accuracy
The importance of data accuracy cannot be overstated. Accurate data is essential for making informed decisions, whether you're managing a business, conducting research, or analyzing trends. When your data is accurate, you can trust the insights it provides, leading to more reliable and effective strategies.
-
Decision-Making: Accurate data plays a pivotal role in decision-making. It ensures that the insights and conclusions you draw are valid and reliable. Inaccurate data, on the other hand, can lead to flawed conclusions and misguided strategies. For instance, if a business relies on incorrect sales data, it might make poor inventory decisions, resulting in financial losses.
-
Operational Efficiency: In the realm of operations, data accuracy is crucial for maintaining efficiency. When your data accurately reflects the current state of affairs, you can optimize processes and allocate resources effectively. Errors in data can lead to inefficiencies, causing delays and increased costs.
-
Research and Analysis: In research, data accuracy affects the validity, reliability, and generalizability of findings. Accurate data ensures that your research results are trustworthy and can be applied to broader contexts. Various methods, such as data validation and cleansing, can help maintain data accuracy in research projects.
-
Customer Satisfaction: For businesses, accurate data is vital for customer satisfaction. It allows you to understand customer needs and preferences, enabling you to tailor your products and services accordingly. Inaccurate data can lead to poor customer experiences, damaging your brand reputation.
-
Compliance and Risk Management: Accurate data is also crucial for compliance with regulatory requirements. It helps you avoid legal issues and penalties by ensuring that your records are correct and up-to-date. Moreover, accurate data aids in risk management by providing a clear picture of potential threats and opportunities.
Understanding Data Integrity
Definition of Data Integrity
Data integrity refers to the consistency, accuracy, and reliability of data throughout its lifecycle. It ensures that your data remains unaltered and trustworthy from the moment it is created until it is archived or deleted. When you maintain data integrity, you protect your data from unauthorized changes, corruption, or loss. This protection is crucial because it allows you to rely on your data for decision-making and compliance with regulations.
Role of Data Integrity in Reliable Systems
Data integrity plays a vital role in creating reliable systems. It ensures that the data you use is consistent and dependable, which is essential for making informed decisions. When data integrity is compromised, the consequences can be severe. For example, during the 2008 financial crisis, bad data overstated the value of mortgage-backed securities and other derivatives, leading to catastrophic financial decisions and widespread economic turmoil.
To maintain data integrity, you should implement robust processes and controls. These may include:
-
Access Controls: Limit who can view or modify your data to prevent unauthorized changes.
-
Backups: Regularly back up your data to protect against loss or corruption.
-
Audits: Conduct regular audits to ensure that your data remains consistent and accurate over time.
By prioritizing data integrity, you create a foundation of trust in your data. This trust enables you to make strategic decisions with confidence, knowing that your data accurately reflects reality. In industries like healthcare, finance, and manufacturing, where the stakes are high, maintaining data integrity is not just beneficial—it's essential for success.
Key Differences Between Data Accuracy and Data Integrity
Comparison of Characteristics
Understanding the differences between data accuracy and data integrity is crucial for effective data management. Data accuracy focuses on how correct and precise the data is. It ensures that the information you rely on is free from errors and accurately represents real-world entities. When you think about data accuracy, consider it as a measure of how closely your data aligns with the truth.
In contrast, data integrity emphasizes whether the data has remained unchanged and intact over time. It ensures that your data remains consistent, reliable, and trustworthy throughout its lifecycle. Data integrity protects your data from unauthorized changes, corruption, or loss, allowing you to rely on it for decision-making and compliance with regulations.
Here are some key differences:
-
Nature: Data accuracy deals with the correctness of data values, while data integrity focuses on maintaining the data's reliability and consistency over time.
-
Objective: The goal of data accuracy is to ensure error-free data, whereas data integrity aims to preserve the data's original state and prevent unauthorized alterations.
-
Scope: Data accuracy is concerned with the precision of individual data points, while data integrity encompasses the overall trustworthiness of the data set.
Interdependencies
While data accuracy and data integrity have distinct characteristics, they are interdependent. You cannot achieve one without the other. Accurate data forms the foundation for maintaining data integrity. If your data is not accurate, it cannot be considered reliable or trustworthy. Similarly, without data integrity, even accurate data can become compromised, leading to incorrect conclusions and decisions.
Consider these interdependencies:
-
Foundation: Accurate data is essential for maintaining data integrity. If your data is not accurate, it cannot be trusted, regardless of how well you protect it from unauthorized changes.
-
Protection: Data integrity safeguards accurate data from corruption or loss. By implementing robust processes and controls, you ensure that your accurate data remains reliable and trustworthy over time.
-
Reliability: Both data accuracy and data integrity contribute to the overall reliability of your data. When you maintain both, you create a foundation of trust in your data, enabling you to make informed decisions with confidence.
By understanding these key differences and interdependencies, you can better navigate the complexities of data management. Prioritizing both data accuracy and data integrity ensures that your data remains a valuable asset for your organization.
Common Pitfalls and Strategies to Avoid Them
In the journey of managing data, you might encounter several pitfalls that can compromise both data accuracy and integrity. Recognizing these common mistakes and implementing strategies to avoid them will help you maintain high-quality data.
Common Mistakes in Data Accuracy
-
Data Entry Errors: Manual data entry often leads to inaccuracies. You might input incorrect figures or misspell names, which can distort the data's true meaning. For instance, in healthcare, a simple typo in patient records can lead to incorrect treatment plans.
-
Outdated Information: Relying on outdated data can mislead your analysis. In sectors like e-commerce, using old customer preferences might result in ineffective marketing strategies.
-
Lack of Validation: Skipping data validation processes can allow errors to persist. Without checks, you risk basing decisions on flawed data. In banking, unvalidated data can lead to incorrect financial assessments.
-
Inconsistent Data Sources: Using multiple data sources without ensuring consistency can create discrepancies. In real estate, inconsistent property data can affect market analysis.
-
Ignoring Data Profiling: Failing to profile data regularly can hide underlying issues. In manufacturing, unprofiled data might lead to production inefficiencies.
Common Mistakes in Data Integrity
-
Unauthorized Access: Allowing unrestricted access to data increases the risk of unauthorized changes. In transportation, unauthorized data alterations can disrupt logistics.
-
Poor Backup Practices: Neglecting regular backups can result in data loss. In academia, losing research data can set back projects significantly.
-
Inadequate Auditing: Without regular audits, you might miss signs of data corruption. In meteorology, corrupted data can lead to inaccurate weather forecasts.
-
Lack of Version Control: Not maintaining version control can lead to confusion over data changes. In research, this can compromise the reliability of findings.
-
Human Error: Simple mistakes, like accidental deletions, can compromise data integrity. In finance, such errors can have significant financial implications.
Strategies for Avoidance
-
Implement Automated Systems: Use automated data entry and validation systems to minimize human error. Automation ensures that data remains accurate and up-to-date.
-
Regular Updates and Cleansing: Schedule regular updates and cleansing sessions to keep your data current. This practice is crucial in sectors like banking, where timely information is key.
-
Consistent Data Standards: Establish and enforce consistent data standards across all sources. This consistency is vital in industries like real estate, where data uniformity enhances analysis.
-
Access Controls and Encryption: Implement strict access controls and encrypt sensitive data to protect it from unauthorized changes. In healthcare, this ensures patient data remains confidential and accurate.
-
Routine Backups and Audits: Conduct routine backups and audits to safeguard data integrity. Regular audits help identify and rectify potential issues before they escalate.
By understanding these pitfalls and adopting these strategies, you can enhance both data accuracy and integrity. This proactive approach ensures that your data remains a reliable asset, supporting informed decision-making across various sectors.
Conclusion
Understanding the distinction between data accuracy and integrity is crucial for effective data management. You must adopt a balanced approach to ensure that your data remains both precise and reliable. This balance not only supports informed decision-making but also upholds ethical standards in data handling.
To stay ahead, you should engage in ongoing education and adapt to new data management practices. This proactive stance will help you navigate the evolving landscape of data ethics and maintain high-quality data.