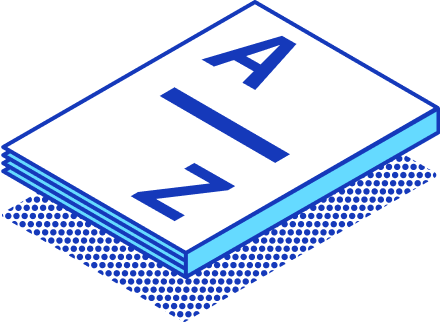
Data Mesh
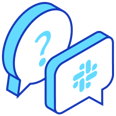
Join StarRocks Community on Slack
Connect on SlackTABLE OF CONTENTS
Publish date: Jul 18, 2024 11:01:24 AM
Data Mesh represents a groundbreaking approach to modern data architecture. This concept decentralizes data ownership, empowering domain teams to manage their data assets independently. The importance of Data Mesh lies in its ability to enhance data quality, improve accessibility, and foster collaboration across organizations. Companies adopting this architecture enjoy faster decision-making and greater innovation. Data Mesh promises to reshape data strategies by decentralizing data management and promoting a data-empowered culture.
What is Data Mesh?
Definition and Core Principles
Data Mesh represents a paradigm shift in data architecture. This approach decentralizes data ownership, allowing domain teams to manage their data independently. The core principles of Data Mesh include decentralized data ownership, treating data as a product, self-serve data infrastructure, and federated computational governance.
Decentralized Data Ownership
Decentralized data ownership assigns responsibility for data to the domain teams that generate and use it. This principle enhances data quality and relevance by placing data management in the hands of those with the most context. Domain teams can make decisions quickly, leading to faster innovation and more accurate insights.
Data as a Product
Treating data as a product involves managing data with the same rigor and care as any other product. Data products must be discoverable, trustworthy, and interoperable. This approach ensures that data meets the needs of its consumers, fostering a culture of accountability and continuous improvement.
Self-serve Data Infrastructure
A self-serve data infrastructure provides tools and platforms that enable domain teams to manage their data autonomously. This infrastructure reduces dependency on central data teams, promoting agility and scalability. Tools and technologies such as data catalogs, data pipelines, and data governance frameworks are essential components of a self-serve data infrastructure.
Federated Computational Governance
Federated computational governance ensures that data management practices are consistent across the organization. This principle balances autonomy with standardization, allowing domain teams to operate independently while adhering to global standards. Federated governance includes policies for data quality, security, and compliance, ensuring that data remains reliable and secure.
Historical Context and Evolution
Understanding the historical context of Data Mesh helps to appreciate its significance. Traditional data architectures have faced challenges that Data Mesh aims to address.
Traditional Data Architectures
Traditional data architectures often rely on centralized data warehouses or data lakes. These architectures can lead to data silos, where data is isolated within specific departments. Centralized data management can also create bottlenecks, slowing down decision-making and innovation. The limitations of traditional architectures have driven the need for a more flexible and scalable approach.
Emergence of Data Mesh
The concept of Data Mesh emerged as a response to the shortcomings of traditional data architectures. Zhamak Dehghani introduced Data Mesh in 2019, highlighting its potential to transform data management. Companies like Zalando, Netflix, Intuit, and PayPal have successfully implemented Data Mesh, demonstrating its effectiveness. Data Mesh offers a forward-thinking framework that aligns with the needs of modern, data-driven organizations. By decentralizing data ownership and fostering a culture of collaboration, Data Mesh empowers domain experts and promotes decentralized decision-making.
Key Components of Data Mesh
Data Mesh Architecture. Source: Data Mesh Architecture website
Domain-oriented Decentralization
Domain-oriented decentralization stands as a cornerstone of the Data Mesh architecture. This principle assigns data ownership to individual domain teams, allowing them to manage and govern their data independently. By aligning data management with domain expertise, organizations can enhance the relevance and utility of their data.
Benefits and Challenges
Domain-oriented decentralization offers several benefits:
-
Enhanced Data Quality: Domain teams possess deep knowledge of their data, leading to more accurate and relevant data management.
-
Improved Efficiency: Decentralized ownership reduces bottlenecks, enabling faster decision-making and innovation.
-
Greater Accountability: Domain teams take responsibility for their data products, fostering a culture of ownership and continuous improvement.
However, this approach also presents challenges:
-
Coordination Complexity: Ensuring consistent data practices across multiple domains requires robust governance mechanisms.
-
Resource Allocation: Domain teams need adequate resources and support to manage their data effectively.
-
Cultural Shift: Organizations must foster a culture that embraces decentralized data ownership and collaboration.
Data Product Thinking
Data Mesh promotes the concept of treating data as a product. This approach involves managing data with the same rigor and care as any other product, ensuring it meets the needs of its consumers.
Characteristics of Data Products
Effective data products share several key characteristics:
-
Discoverability: Data products must be easily discoverable by users within the organization. Tools like data catalogs can facilitate this process.
-
Trustworthiness: Data products should be reliable and accurate, instilling confidence in users who rely on them for decision-making.
-
Interoperability: Data products must be compatible with other data sources and systems, enabling seamless integration and analysis.
-
User-Centric Design: Data products should be designed with the end-user in mind, ensuring they meet specific business needs and use cases.
Self-serve Data Infrastructure
A self-serve data infrastructure is essential for empowering domain teams to manage their data autonomously. This infrastructure provides the tools and platforms necessary for independent data management.
Tools and Technologies
Several tools and technologies play a crucial role in a self-serve data infrastructure:
-
Data Catalogs: These tools help users discover and understand available data products within the organization.
-
Data Pipelines: Automated data pipelines streamline the process of data ingestion, transformation, and delivery.
-
Data Governance Frameworks: These frameworks ensure that data management practices adhere to organizational policies and standards.
-
Self-service Analytics Platforms: These platforms enable users to perform data analysis and visualization without relying on central data teams.
A well-designed self-serve data infrastructure promotes agility and scalability, allowing domain teams to respond quickly to changing business needs.
Federated Governance
Federated governance serves as a critical component of Data Mesh architecture. This principle ensures consistent data management practices across an organization. Federated governance balances autonomy with standardization, allowing domain teams to operate independently while adhering to global standards.
Ensuring Data Quality and Compliance
Ensuring data quality and compliance remains paramount in a federated governance model. Organizations must establish robust policies and frameworks to maintain high standards. These policies cover various aspects, including data quality, security, and regulatory compliance.
Data Quality
Data quality involves several key dimensions:
-
Accuracy: Data must accurately represent the real-world entities or events it describes.
-
Completeness: Data should include all necessary information to meet business requirements.
-
Consistency: Data must remain consistent across different systems and datasets.
-
Timeliness: Data should be available when needed for decision-making processes.
Domain teams play a crucial role in maintaining these dimensions. Teams possess deep knowledge of their specific data, enabling precise and efficient data handling. This approach enhances the relevance and utility of data by aligning with domain needs and expertise.
Data Compliance
Compliance with regulatory requirements is essential for any organization. Federated governance includes policies that ensure adherence to relevant laws and regulations. These policies cover data privacy, security, and ethical considerations.
Organizations must implement mechanisms to monitor and enforce compliance. Automated tools can help track data usage and identify potential violations. Regular audits and reviews ensure ongoing adherence to policies.
Collaborative Governance
Collaborative governance involves cooperation between domain teams and central governance bodies. This collaboration ensures that local practices align with organizational standards. Regular communication and feedback loops foster a culture of continuous improvement.
Tools and Technologies
Several tools and technologies support federated governance:
-
Data Catalogs: These tools help users discover and understand available data products within the organization.
-
Data Quality Tools: Automated tools assess and improve data quality across different domains.
-
Compliance Monitoring Tools: These tools track data usage and ensure adherence to regulatory requirements.
-
Collaboration Platforms: Platforms facilitate communication and collaboration between domain teams and central governance bodies.
Case Study: Delivery Hero
Delivery Hero adopted a Data Mesh approach to manage its vast data landscape. The company implemented decentralized data ownership, with domain teams responsible for their data products. Delivery Hero established a federated governance model to ensure consistent data practices across the organization. This approach enabled the company to maintain high data quality and compliance standards while empowering domain teams to innovate and make data-driven decisions.
Conclusion
Federated governance plays a vital role in the successful implementation of Data Mesh. By ensuring data quality and compliance, organizations can harness the full potential of their data assets. Collaborative governance and the use of advanced tools and technologies further enhance the effectiveness of this approach. Embracing federated governance allows organizations to create a robust and scalable data architecture that meets the demands of modern data-driven environments.
Implementing Data Mesh in Your Organization
Steps to Get Started
Assessing Readiness
Organizations must evaluate their current data architecture before implementing Data Mesh. This assessment involves understanding existing data workflows, identifying data silos, and determining the maturity of data governance practices. Leaders should engage with domain teams to gauge their readiness for decentralized data ownership. A thorough readiness assessment helps identify gaps and areas needing improvement.
Building a Roadmap
A clear roadmap is essential for a successful Data Mesh implementation. This roadmap should outline key milestones, timelines, and resource allocation. Organizations must prioritize initiatives that align with business goals. The roadmap should also include training programs to educate domain teams on Data Mesh principles. Regular reviews and updates to the roadmap ensure alignment with evolving business needs.
Best Practices
Collaboration and Communication
Effective collaboration and communication are crucial for Data Mesh success. Domain teams must work closely with central governance bodies to ensure consistency in data practices. Regular meetings and workshops foster a culture of knowledge sharing. Tools like collaboration platforms facilitate seamless communication across teams. Organizations should establish feedback loops to continuously improve data management practices.
Continuous Improvement
Continuous improvement is a core principle of Data Mesh. Organizations must regularly review and refine their data processes. Metrics and KPIs should track the performance of data products. Domain teams should conduct regular audits to ensure data quality and compliance. A culture of continuous improvement drives innovation and enhances data reliability.
Common Pitfalls and How to Avoid Them
Overcoming Resistance to Change
Resistance to change is a common challenge in Data Mesh implementation. Organizations must address this by fostering a culture that embraces change. Leaders should communicate the benefits of Data Mesh clearly and consistently. Training programs can help domain teams understand the new responsibilities and tools. Engaging stakeholders early in the process ensures buy-in and reduces resistance.
Ensuring Scalability
Scalability is critical for the long-term success of Data Mesh. Organizations must design their data infrastructure to handle increasing data volumes and complexity. This involves selecting scalable tools and technologies. Regular performance assessments help identify potential bottlenecks. Ensuring scalability requires a proactive approach to infrastructure management.
Advanced Topics in Data Mesh
Case Studies and Real-world Examples
Case Study: Zalando
Zalando, a leading European e-commerce company, adopted Data Mesh to address data scalability issues. Zalando's traditional data architecture relied on centralized data warehouses, which created bottlenecks. The company faced challenges in data accessibility and quality. Zalando transitioned to a Data Mesh architecture to decentralize data ownership.
Domain teams at Zalando took responsibility for their data products. This shift enabled faster decision-making and improved data quality. Zalando implemented a self-serve data infrastructure, providing tools like data catalogs and automated data pipelines. These tools empowered domain teams to manage data autonomously.
Federated governance played a crucial role in Zalando's Data Mesh implementation. The company established policies for data quality, security, and compliance. Regular audits ensured adherence to these standards. Zalando's approach to Data Mesh resulted in enhanced data accessibility and reliability, fostering innovation across the organization.
Case Study: Netflix
Netflix, a global streaming giant, leveraged Data Mesh to enhance its data management practices. The company's vast data landscape required a scalable and flexible architecture. Netflix adopted Data Mesh to decentralize data ownership and promote collaboration among domain teams.
Domain-oriented decentralization allowed Netflix's teams to manage their data independently. This approach reduced bottlenecks and improved data relevance. Netflix treated data as a product, ensuring discoverability, trustworthiness, and interoperability. The company used self-serve data infrastructure tools to enable autonomous data management.
Federated computational governance ensured consistent data practices across Netflix. Policies for data quality and compliance maintained high standards. Collaboration between domain teams and central governance bodies fostered continuous improvement. Netflix's Data Mesh implementation led to faster innovation and better decision-making.
Case Study: Intuit
Intuit, a financial software company, adopted Data Mesh to overcome data silos and improve data accessibility. The company's traditional data architecture hindered data sharing and collaboration. Intuit transitioned to a Data Mesh framework to decentralize data ownership and enhance data quality.
Domain teams at Intuit managed their data products independently. This approach aligned data management with domain expertise, improving data relevance. Intuit implemented a self-serve data infrastructure, providing tools like data catalogs and self-service analytics platforms. These tools enabled domain teams to manage data autonomously.
Federated governance ensured consistent data management practices at Intuit. The company established robust policies for data quality, security, and compliance. Regular reviews and audits maintained high standards. Intuit's Data Mesh implementation resulted in improved data accessibility and reliability, driving innovation and efficiency.
Case Study: PayPal
PayPal, a global payment platform, leveraged Data Mesh to enhance its data management capabilities. The company's traditional data architecture faced challenges in scalability and data quality. PayPal adopted Data Mesh to decentralize data ownership and promote collaboration among domain teams.
Domain-oriented decentralization allowed PayPal's teams to manage their data independently. This approach reduced bottlenecks and improved data relevance. PayPal treated data as a product, ensuring discoverability, trustworthiness, and interoperability. The company used self-serve data infrastructure tools to enable autonomous data management.
Federated computational governance played a vital role in PayPal's Data Mesh implementation. The company established policies for data quality, security, and compliance. Regular audits ensured adherence to these standards. PayPal's approach to Data Mesh resulted in enhanced data accessibility and reliability, fostering innovation across the organization.
Conclusion
Real-world examples from companies like Zalando, Netflix, Intuit, and PayPal demonstrate the effectiveness of Data Mesh. These organizations successfully decentralized data ownership, enhanced data quality, and improved accessibility. Implementing Data Mesh requires robust governance strategies and a commitment to continuous improvement. The experiences of these companies highlight the transformative potential of Data Mesh in modern data architecture.
Data Mesh offers significant benefits for modern data architecture. Companies like Zalando and Delivery Hero have successfully implemented Data Mesh, enhancing data accessibility and quality. Real-time analytics and improved decision-making have become achievable goals. Government agencies have also optimized public services by adopting Data Mesh principles, leading to better policy development and service delivery.
Organizations should explore and implement Data Mesh to unlock these advantages. Embracing this approach can revolutionize data management practices. The future of data architecture lies in decentralized ownership, fostering a culture of innovation and collaboration.