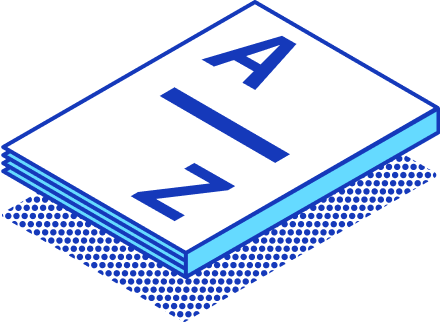
Key Real-Time Processing Trends Shaping the Future
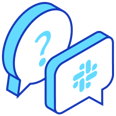
Join StarRocks Community on Slack
Connect on SlackReal-time processing plays a pivotal role in maintaining a competitive edge across various industries. Companies leverage real-time data to respond promptly to changing conditions, enhancing operational efficiency and decision-making. In fact, 80% of businesses report increased revenue through real-time insights. This Comprehensive Guide explores how these trends transform business strategies. Experts predict a 21.5% growth in the real-time data processing market from 2022-2028, underscoring its significance. As organizations seek immediate insights, this Comprehensive OverviewThe Comprehensive Guide provides a comprehensive understanding of the evolving landscape.
AI Integration in Real-Time Data Processing
Understanding AI Integration
Artificial Intelligence (AI) has become a cornerstone in the realm of real-time data processing. It encompasses a wide range of technologies that enable computers to perform tasks typically requiring human intelligence. Advanced analytics models leverage AI to process and analyze data streams, allowing businesses to make informed decisions swiftly. These models utilize machine learning and deep learning to identify patterns and predict outcomes, optimizing operations and enhancing efficiency.
Key technologies driving AI integration include neural networks, natural language processing, and computer vision. These technologies empower systems to learn from data continuously, improving their accuracy and reliability. By incorporating AI into real-time data flows, companies can automate complex decision-making processes and conduct predictive analysis instantaneously.
Benefits of AI Integration
AI integration offers numerous benefits in real-time data processing. Enhanced decision-making capabilities stand out as a primary advantage. AI refines predictions by drawing from continuous data streams and learning from ongoing patterns. This allows businesses to act swiftly and confidently, as noted by experts in AI and real-time analytics.
Improved efficiency and accuracy also result from AI integration. Advanced AI models transform real-time analytics, enabling firms to extract valuable insights quickly. By automating processes and optimizing operations, companies can navigate the fast-paced digital landscape effectively.
Real-World Applications
AI integration finds applications across various industries, including healthcare, finance, and manufacturing. In healthcare, AI-driven real-time monitoring of patient vitals leads to timely interventions, improving patient outcomes. Financial institutions use AI to detect and respond to fraudulent activities as they occur, safeguarding assets and customer trust. In manufacturing, AI optimizes production processes by predicting equipment failures and minimizing downtime.
Success stories and case studies highlight the transformative impact of AI in real-time data processing. For instance, a leading financial firm implemented AI models to enhance fraud detection, resulting in a significant reduction in fraudulent transactions. Similarly, a healthcare provider leveraged AI to improve patient care, achieving better health outcomes and increased patient satisfaction.
The Rise of Edge Computing in Real-Time Processing
What is Edge Computing?
Edge computing represents a transformative approach to data processing. It involves processing data closer to its source rather than relying on centralized cloud servers. This architecture reduces latency, enabling faster response times crucial for applications like fraud detection and equipment fault diagnosis. Unlike traditional cloud computing, which abstracts application infrastructure by placing server hardware in private data centers, edge computing brings computation and storage closer to the geographical location of the data source. This proximity allows organizations to analyze and act upon data in near real-time.
Advantages of Edge Computing
Edge computing offers several advantages over traditional cloud-based data integration.
-
Reduced Latency and Faster Processing: By processing data at the source, edge computing minimizes the time it takes for data to travel to a central server and back. This reduction in latency is vital for applications requiring immediate insights, such as autonomous vehicles and industrial automation.
-
Enhanced Data Security and Privacy: Edge computing enhances data security by keeping sensitive information closer to its source. This localized processing reduces the risk of data breaches during transmission to centralized cloud servers. Additionally, it provides more control over data privacy, as organizations can implement security measures tailored to their specific needs.
Industry Applications
Edge computing finds applications across various industries, revolutionizing how they handle data analytics.
-
Implementation in IoT and Smart Cities: In the realm of the Internet of Things (IoT), edge computing enables devices to process data locally, reducing the need for constant cloud communication. This capability proves essential for smart cities, where real-time data analysis optimizes traffic management, energy consumption, and public safety.
-
Impact on Autonomous Vehicles and Industrial Automation: Autonomous vehicles rely on edge computing to process vast amounts of data from sensors and cameras in real-time. This local processing ensures quick decision-making, enhancing safety and efficiency. Similarly, in industrial automation, edge computing allows for real-time monitoring and control of machinery, minimizing downtime and improving productivity.
Edge computing complements cloud-based data integration by bringing cloud services closer to end-user devices. This distributed framework addresses the limitations of centralized cloud computing, providing quicker results, more immediate insights, and better security. As industries continue to embrace edge computing, they unlock new possibilities for data analytics and real-time processing.
IT-OT Convergence and Real-Time Data Processing
Defining IT-OT Convergence
Overview of IT and OT systems
Information Technology (IT) and Operational Technology (OT) systems serve distinct roles within organizations. IT systems manage data processing, storage, and communication, while OT systems control physical devices and processes in industries like manufacturing and utilities. Traditionally, these systems operated independently, each with its own set of technologies and protocols.
The significance of their integration
Integrating IT and OT systems creates a unified framework that enhances real-time data processing capabilities. This convergence enables businesses to leverage data from both domains, facilitating predictive and prescriptive analytics. By bridging the gap between information systems and operational processes, organizations can achieve greater operational efficiency and improved security.
Benefits of Convergence
Streamlined operations and improved productivity
IT-OT convergence streamlines operations by enabling seamless communication between IT and OT systems. This integration allows for the automation of processes, reducing manual intervention and minimizing errors. As a result, businesses experience increased productivity and efficiency, leading to cost savings and enhanced competitiveness.
Enhanced data analytics and insights
The convergence of IT and OT systems enhances data analytics by providing a comprehensive view of operations. Organizations can utilize advanced data mining techniques to extract valuable insights from real-time data streams. This capability empowers businesses to make informed decisions quickly, optimizing operations and driving business success.
Practical Implementations
Examples in energy and utilities sectors
In the energy and utilities sectors, IT-OT convergence plays a crucial role in optimizing operations. For instance, digital twins and predictive maintenance models enable companies to monitor equipment performance in real-time, reducing downtime and improving reliability. Additionally, data visualization tools provide operators with actionable insights, enhancing decision-making processes.
Case studies in manufacturing and logistics
Manufacturers adopting Industry 4.0 principles focus on IT-OT convergence to increase efficiency and scale operations. By leveraging automation and data management trends, they achieve tangible benefits such as improved cybersecurity, cloud services integration, and augmented reality applications. In logistics, IT-OT convergence facilitates real-time tracking and data democratization, ensuring efficient supply chain management.
Real-Time Data Streaming and Its Impact
Exploring Real-Time Data Streaming
Real-time data streaming represents a transformative shift in how organizations handle data. It involves the continuous flow of data from sources to processing systems, allowing for immediate analysis and action. This approach eliminates latency, enabling organizations to respond swiftly to dynamic events. Technologies such as Apache Kafka and Amazon Kinesis play a crucial role in facilitating real-time data integration, ensuring that data streams seamlessly into analytics systems.
Advantages of Real-Time Streaming
Real-time data streaming offers several advantages that enhance business operations:
-
Immediate Insights and Faster Decision-Making: By processing data as it is generated, organizations gain immediate insights. This capability allows for quicker decision-making, which is crucial in fast-paced environments like finance and telecommunications.
-
Scalability and Flexibility in Data Handling: Real-time streaming provides scalability, accommodating large volumes of data without compromising performance. This flexibility ensures that businesses can adapt to changing data demands efficiently.
Volt Active Data highlights that real-time data streaming facilitates the continuous and instantaneous flow of data, uncovering actionable insights with near immediacy. This capability empowers organizations to embrace advanced data analytics and stay ahead of the competition.
Applications Across Industries
Real-time data streaming finds applications across various industries, transforming how they operate:
-
Use in Media, Entertainment, and E-Commerce: In media and entertainment, real-time streaming enables personalized content delivery, enhancing user experiences. E-commerce platforms leverage real-time data integration to adjust pricing dynamically and personalize customer interactions.
-
Impact on Financial Services and Telecommunications: Financial institutions use real-time analytics platforms to detect and respond to fraudulent activities instantly. In telecommunications, real-time data streaming optimizes network performance and enhances customer service.
Imply.io notes that real-time data analytics offers instant insights and actionable data from ongoing streams. This capability proves essential in sectors like manufacturing, DevOps, and cybersecurity, where large volumes of data move at speed.
The future of big data hinges on real-time data management trends, as organizations increasingly adopt real-time stream analytics to drive business success. By embracing advanced data analytics, businesses can unlock new possibilities and gain a competitive edge in the evolving landscape of data analytics technology trends.
Data Security and Real-Time Processing
Importance of Data Security
Data security remains a critical concern in real-time processing. Organizations must address several challenges to protect sensitive information effectively.
Challenges in Securing Real-Time Data
Real-time data processing involves continuous data streams, which can expose vulnerabilities. These vulnerabilities include data integration issues, quality inconsistencies, and latency problems. Additionally, the sheer volume of data can overwhelm systems, making it difficult to maintain security. Legacy systems often lack the necessary security features, further complicating the protection of real-time data.
Solutions and Best Practices
To safeguard real-time data, organizations should implement robust security measures. Data encryption ensures that information remains confidential during transmission. Access control restricts data access to authorized personnel only. Regular audits help identify potential security breaches and ensure compliance with data governance trends. Furthermore, organizations should adopt comprehensive cybersecurity protocols, including system updates and anomaly detection, to protect against threats.
Real-World Implications
The implications of data security in real-time processing extend across various industries, highlighting its importance.
Case Studies in Finance and Healthcare
In the finance sector, real-time data processing plays a vital role in fraud detection. Financial institutions monitor transactions continuously, identifying suspicious activities instantly. This proactive approach helps safeguard assets and maintain customer trust. In healthcare, real-time monitoring of patient data requires stringent security measures to protect sensitive information. Hospitals implement data encryption and access controls to ensure patient privacy and comply with regulations.
Future Trends in Data Security
As data governance trends evolve, organizations must adapt to new security challenges. The integration of artificial intelligence and machine learning in real-time processing will enhance security measures by predicting and mitigating threats. Additionally, advancements in edge computing will enable localized data processing, reducing the risk of data breaches during transmission. Organizations must stay informed about these trends to maintain robust data security in an ever-changing landscape.
Tools and Technologies for Real-Time Data Processing
Overview of Key Tools
Apache Kafka and Spark
Apache Kafka stands as a leading event streaming platform, widely adopted by companies like Uber and Netflix. It excels in real-time data processing by enabling data pipelines that generate near-immediate insights. Kafka's architecture supports high throughput and fault-tolerant storage, making it ideal for handling large volumes of data. It allows organizations to publish and subscribe to real-time data streams, facilitating seamless data integration and analysis.
Apache Spark, another powerful tool, complements Kafka by providing a unified analytics engine for big data processing. Spark's in-memory computing capabilities enhance the speed of data processing, making it suitable for real-time analytics. Together, Kafka and Spark form a robust framework for managing and analyzing streaming data efficiently.
Portable's Platform and Its Features
Portable offers an innovative platform designed for real-time data processing. It integrates seamlessly with various data sources, utilizing advanced technologies to ensure low latency and high throughput. Portable's architecture supports scalable operations, allowing businesses to handle growing data volumes without compromising performance. The platform's no-code integrations simplify the implementation process, enabling organizations to leverage real-time analytics without extensive technical expertise.
Benefits of Using Advanced Tools
Low Latency and High Throughput
Advanced tools like Kafka and Portable provide low latency and high throughput, essential for real-time data processing. By minimizing the time it takes to process data, these tools enable organizations to gain immediate insights and make timely decisions. This capability proves crucial in fast-paced industries such as finance and telecommunications, where rapid response times are vital.
Seamless Integration with Data Sources
Seamless integration with data sources is another significant benefit of using advanced tools. Platforms like Portable and Astera Centerprise offer easy connectivity to various data sources, ensuring that data flows smoothly into analytics systems. This integration allows businesses to harness the full potential of their data, driving informed decision-making and enhancing operational efficiency.
The Data Leaders Forum emphasizes the importance of using advanced data analytics tools to stay competitive in today's data-driven world. During the Leaders Forum recap, experts highlighted how tools like Kafka and Portable empower organizations to extract valuable insights from real-time data streams. As noted by Jessup University, these tools play a pivotal role in transforming data into actionable intelligence, enabling businesses to thrive in an ever-evolving landscape.
In conclusion, the adoption of advanced tools and technologies for real-time data processing is essential for organizations seeking to leverage immediate insights. By utilizing platforms like Kafka, Spark, and Portable, businesses can enhance their data processing capabilities, ensuring they remain at the forefront of innovation and growth.
Conclusion
The blog explored key trends in real-time data processing, highlighting their transformative impact across industries. These trends, including AI integration, edge computing, and IT-OT convergence, are reshaping how businesses operate. They enable organizations to harness immediate insights, enhancing decision-making and operational efficiency. As data volumes grow, these trends will traverse all sectors, making scaling essential for survival. Companies must stay informed and adapt to these evolving technologies to maintain a competitive edge. Tools like Portable play a crucial role in facilitating real-time data processing, empowering businesses to thrive in a data-driven world.