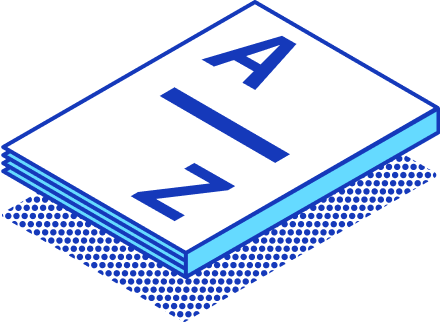
Apache Iceberg
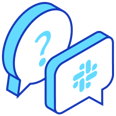
Join StarRocks Community on Slack
Connect on SlackWhat Is Apache Iceberg?
What Are the Components of the Iceberg Architecture?
Iceberg employs a layered approach to data management, distinguishing between the metadata management layer and the data storage layer.
Data Layer: This layer contains the actual data files, such as Parquet and ORC files, which are the physical carriers for storing data.
Metadata Layer: The metadata layer in Iceberg is multi-tiered and is responsible for storing the structure of the table and the indexing information of the data files.
Catalog Layer: This layer stores pointers to the locations of the metadata files, serving as an index for the metadata. Iceberg provides several catalog implementations, such as:
- HadoopCatalog: Directly uses Hadoop filesystem directories to manage table information.
- HiveCatalog: Stores table metadata in the Hive Metastore, facilitating integration with the existing Hive ecosystem.
The metadata management is further divided into three key components:
- Metadata File: Stores the current version's metadata, including all snapshot information.
- Snapshot: Represents a specific operation's snapshot, with each commit generating a new snapshot containing multiple manifests detailing the addresses of the generated data files.
- Manifest: Lists the data files associated with a snapshot, providing a comprehensive view of the data's organization and facilitating efficient data retrieval and modification.
At its core, Iceberg aims to track all changes to a table over time through snapshots, which represent complete collections of table data files at any given moment. Each update operation generates a new snapshot, ensuring data consistency and facilitating historical data analysis and incremental reads.
Key Aspects of Iceberg Table Format
Hidden Partition
- Hidden Partitions: Iceberg allows users to organize data partitions based on timestamps with different granularities (e.g., year, month, day, or hour). Partition information is transparent to users.
- Syntax Usage: Use syntax like
CREATE TABLE catalog.MyTable (..., ts TIMESTAMP) PARTITIONED BY days(ts)
to create partitions based on daily timestamps. New data is automatically placed in the corresponding partitions.
Schema Evolution
- Flexible Schema Evolution: Iceberg provides flexible schema evolution capabilities, supporting DDL operations like adding, deleting, renaming, and updating columns.
- Metadata Updates: Schema changes only update metadata without rewriting or moving data files. Each change is recorded in the metadata, ensuring historical schemas can be tracked and queried.
- Unique Column IDs: Each column in the table is tracked with a unique column ID, ensuring the correctness of read and write operations on files.
Partition Evolution
- Partition Strategy Modification: When modifying partition strategies, existing data files retain their original partition strategy, while new data applies the new strategy.
- Historical Partition Metadata: Metadata records all historical partition schema information.
- Execution Plans: Iceberg can generate different execution plans to handle data involving different partition strategies.
MVCC (Multi-Version Concurrency Control)
- MVCC Mechanism: Ensures that data writes do not interfere with read operations. Each read accesses the latest data snapshot.
- Delete and Overwrite Operations: Modify the status of Parquet files in the Manifest file rather than directly deleting data files.
Consistency
- Concurrent Data Writes: Supports concurrent data writes using an optimistic locking mechanism to handle write conflicts.
- Snapshot-Based Writes: Write operations are based on the same data snapshot. If there are no conflicts, both operations can succeed; if there is a conflict, only one operation succeeds.
Row-Level Update: COW & MOR
- COW (Copy on Write): Supported in V1 table format. Updates data by copying the original data and applying changes to the copy.
- MOR (Merge on Read): Supported in V2 table format. Introduces position delete files and equality delete files to perform logical deletions, reflecting the latest state during data reads.
- Position Delete: Records the position of data rows to be deleted, suitable for scenarios where data changes infrequently.
- Equality Delete: Saves the value of the data to be deleted and executes the deletion through comparison during reads.
Exploring the Benefits of Apache Iceberg
Comprehensive Compute Engine Support
Iceberg's superior kernel abstraction ensures that it is not tied to any specific compute engine, providing broad support for popular processing frameworks like Spark, Flink, and Hive. This flexibility allows users to integrate Iceberg seamlessly into their existing data infrastructure, leveraging the native Java API for direct access to Iceberg tables without being constrained by the choice of computation engine.
Flexible File Organization
Iceberg introduces innovative data organization strategies that support both stream-based incremental and batch full-table computation models. This versatility ensures that both batch tasks and streaming tasks can operate on the same storage model, such as HDFS or OZONE—a next-generation storage engine developed by the Hadoop community. By enabling hidden partitioning and partition layout evolution, Iceberg facilitates easy updates to data partitioning strategies, supporting a variety of storage formats including Parquet, ORC, and Avro. This approach not only eliminates data silos but also aids in building cost-effective, lightweight data lake storage services.
Optimized Data Ingestion Workflow
With its ACID transaction capabilities, Iceberg ensures that newly ingested data is immediately visible, significantly simplifying the ETL process by eliminating the impact on current data processing tasks. The platform's support for upserts and merge into operations at the row level further reduces the latency involved in data ingestion, streamlining the overall data flow into the data lake.
Incremental Read Capabilities
One of Iceberg's standout features is its support for reading incremental data in a streaming fashion, enabling a tight integration with mainstream open-source compute engines for both data ingestion and analysis. This feature is complemented by built-in support for Spark Structured Streaming and Flink Table Source, allowing for sophisticated data analysis workflows. Additionally, Iceberg's ability to perform historical version backtracking enhances data reliability and auditability, offering valuable insights into data evolution over time.
Where and When to Use Apache Iceberg
As one of the core components of a universal data lake solution, Iceberg is primarily suitable for the following scenarios:
Real-time Data Import and Querying
Data flows in real-time from upstream to the Iceberg data lake, where it can be immediately queried. For example, in logging scenarios, Iceberg or Spark streaming jobs are initiated to import log data into Iceberg tables in real-time. This data can then be queried in real-time using Hive, Spark, Iceberg, or Presto. Moreover, Iceberg's support for ACID transactions ensures the isolation of data inflow and querying, preventing dirty data.
Data Deletion or Updating
Most data warehouses struggle to efficiently perform row-level data deletions or updates, typically requiring offline jobs to extract the entire table's raw data, modify it, and then write it back to the original table. Iceberg, however, narrows the scope of changes from the table level to the file level, allowing for localized changes to execute business logic for data modifications or deletions. Within the Iceberg data lake, you can directly execute commands like DELETE FROM test_table WHERE id > 10
to make changes to the data in the table.
Data Quality Control
With the validation function of the Iceberg Schema, abnormal data is excluded during data import, or further processing is performed on abnormal data.
Data Schema Changes
The schema of the data is not fixed and can change; Iceberg supports making changes to the table structure using Spark SQL's DDL statements. When changing the table structure in Iceberg, it is not necessary to re-export all historical data according to the new schema, which greatly speeds up the schema change process. Additionally, Iceberg's support for ACID transactions effectively isolates schema changes from affecting existing read tasks, allowing you to access consistently accurate data.
Real-time Machine Learning
In machine learning scenarios, a significant amount of time is often spent processing data, such as cleaning, transforming, and extracting features, as well as dealing with both historical and real-time data. Iceberg simplifies this workflow, turning the entire data processing process into a complete, reliable real-time stream. Operations like data cleaning, transformation, and feature engineering are all node actions on the stream, eliminating the need to handle historical and real-time data separately. Furthermore, Iceberg also supports a native Python SDK, making it very user-friendly for developers of machine learning algorithms.
Comparison: Apache Iceberg vs. Apache Hive, Apache Hudi, and Delta Lake
Is Apache Hive Still Relevant? Exploring Its Limitations and Challenges
Despite Apache Hive's significant role as an early data warehouse solution, it has inherent performance and flexibility limitations that pose challenges for data processing and analysis in practical applications.
- High Cost of Data Updates and Lack of Real-Time Capabilities: Hive lacks the ability to perform row-level updates efficiently. Updating a single row within a partition typically requires rewriting the entire partition, resulting in significant inefficiencies.
- Lack of ACID Transactions and Issues with Concurrent Modifications: Until version 3.0, Hive did not support ACID transactions, making it unable to handle concurrent modifications to datasets. This often led to data conflicts and inconsistencies.
- Non-Atomic Writes: Overwrite operations on multiple partitions may partially succeed, leading to data inconsistencies and the presence of dirty data.
- High Cost of Schema Changes: Schema modifications, such as adding or dropping columns, usually require the entire table to be rebuilt and re-imported, which is resource-intensive.
- Partition Scheme Change Limitations: Hive does not support changing the schema of existing partitions. Altering the partitioning strategy necessitates creating a new table and migrating data.
- Lack of File-Level Metadata: Hive's table format defines data organization only at the partition level without including specific file information. Retrieving this information requires listing directories via the file system, which is costly.
- No Automatic Derivation of Partition Columns: Partition columns must be explicitly defined and cannot be automatically derived from data columns, increasing the complexity of data management and querying.
- Delayed Statistics Updates: The delay in updating statistics can cause the query optimizer to produce suboptimal execution plans, negatively impacting query performance.
- Poor Compatibility with Cloud Storage: When using object storage services like Amazon S3, Hive's partition mechanism may encounter performance limitations due to S3's constraints on listing files with the same prefix.
-
Apache Iceberg: Stands out for its broad engine compatibility (including Spark, Flink, Presto, and Trino), making it highly versatile. Iceberg's design is optimized for cloud storage efficiency and performance at petabyte scale, addressing issues like metadata scalability and file listing overheads inherent in cloud object stores.
- Apache Hudi: Provides similar capabilities for record-level inserts, updates, and deletes. Hudi is designed to offer faster incremental processing and comes with built-in support for change data capture (CDC), stream processing, and data privacy use cases.
- Delta Lake: Like Iceberg, Delta Lake offers ACID transactions, schema evolution, and time travel. However, it is closely integrated with Spark and Databricks, potentially limiting its flexibility with other processing engines.
Apache Iceberg + StarRocks
StarRocks is the ultimate tool for accelerating queries on Iceberg. It efficiently analyzes data stored locally and can also serve as a computing engine to directly analyze data in data lakes. Users can effortlessly query data stored on Iceberg using the External Catalog provided by StarRocks, eliminating the need for data migration. StarRocks supports reading Iceberg v1 and v2 tables (including position delete and equality delete) and also allows writing data into Iceberg tables.
A query's efficiency relies on metadata retrieval and parsing, execution plan generation, and efficient execution. In scenarios involving Iceberg queries, StarRocks leverages its native vectorized engine and CBO optimizer capabilities and introduces additional optimizations for querying data on lakes. This ensures that queries are executed swiftly and efficiently, making StarRocks a powerful tool for handling Iceberg data.
Explore how industry leaders are transforming their data lakes and lakehouses with Iceberg and StarRocks through our curated case studies. Get direct insights into their design strategies and the benefits they're achieving with these technologies.
-
A leading social media company has shortened its development cycle and improved cost-effectiveness for its trillions of daily records of data by switching to a data lakehouse architecture. Read the case study.
-
A gaming giant is reducing storage costs by 15x while eliminating all pre-aggregations through unifying all workloads on its data lakehouse. Read the case study.
-
An AB testing SAAS platform is unifying its demanding customer-facing workloads on the data lakehouse. Read the case study.
CelerData Cloud + Tabular Managed Iceberg
If you are looking for enterprise-standard features, dedicated support, or just want to these benefits on the cloud, check out our StarRocks-powered CelerData Cloud. Sign up at cloud.celerdata.com for a free trial and see what it can do for you.