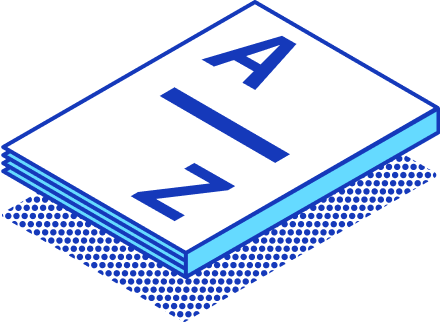
Correlation Analysis
What Is a Correlation Analysis
Definition and Basic Concepts
Correlation Analysis serves as a fundamental tool in understanding the relationship between two variables. This Analysis measures how one variable affects another, providing valuable Insights into their relationship. Researchers often use Correlation to identify patterns within data. The Analysis involves calculating a coefficient that indicates the strength and direction of the relationship.
Understanding Variables
Variables are the core components of any Correlation Analysis. These elements represent measurable characteristics or quantities that can change. For example, temperature and ice cream sales can be variables in a study. Understanding how these variables interact is crucial for deriving meaningful Insights. Researchers must carefully select and define variables to ensure accurate Analysis.
Types of Correlation
Different types of Correlation exist, each revealing unique relationships. Positive Correlation occurs when both variables increase together. Negative Correlation happens when one variable decreases as the other increases. No Correlation indicates no discernible pattern between the variables. Spearman's Rank Correlation and Pearson's Correlation Coefficient are common Methods used to assess these relationships. Spearman's Rank is particularly useful when dealing with ordinal data.
Importance of Correlation Analysis
Correlation Analysis plays a vital role in various fields, offering significant benefits. This Analysis provides a starting point for further research and helps in understanding the behavior between two variables. Drive Research emphasizes the importance of recognizing these relationships for informed decision-making.
Identifying Relationships
Identifying relationships between variables is a primary goal of Correlation Analysis. This Analysis reveals how much one variable influences another. For instance, Drive Research might use Correlation to determine how customer satisfaction impacts sales. Recognizing these relationships allows businesses to make strategic decisions based on data-driven Insights.
Predictive Analysis
Correlation Analysis also aids in predictive Analysis. By understanding relationships, researchers can forecast future trends. Drive Research utilizes Correlation to predict market behaviors, enhancing strategic planning. This predictive capability makes Correlation Analysis an invaluable tool in research and business Analysis.
How to Measure Correlation
Correlation Coefficients
Understanding correlation coefficients is crucial for effective analysis. These coefficients measure the strength and direction of the relationship between two variables. The range of a correlation coefficient is from -1 to +1. A value of +1 indicates a perfect positive relationship, while -1 signifies a perfect negative relationship. A value of 0 suggests no relationship exists between the variables.
Pearson's Correlation Coefficient
Pearson's correlation coefficient is widely used in research. This method measures the linear relationship between two continuous variables. Researchers often use Pearson's coefficient when data follows a normal distribution. The calculation involves comparing the covariance of the variables to their individual standard deviations. A high Pearson's coefficient indicates a strong linear relationship.
Spearman's Rank Correlation
Spearman's Rank correlation is another valuable tool in analysis. This method assesses the monotonic relationship between two variables. Unlike Pearson's, Spearman's Rank does not require normally distributed data. Researchers use this method for ordinal data or when outliers are present. Spearman's Rank provides insights into the order of data rather than their exact values.
Interpreting Correlation Coefficients
Interpreting correlation coefficients helps in understanding relationships. Positive and negative correlations reveal different dynamics between variables.
Positive vs. Negative Correlation
A positive correlation means both variables increase together. For example, as temperature rises, ice cream sales might also rise. A negative correlation indicates that as one variable increases, the other decreases. An example includes the relationship between exercise frequency and body weight.
Strength of Correlation
The strength of a correlation reveals how closely variables are related. A high correlation coefficient, close to +1 or -1, indicates a strong relationship. A low correlation coefficient, near 0, suggests a weak relationship. Understanding the strength helps in making informed decisions in market research analysis.
Applications of Correlation Analysis
In Business and Economics
Market Trends
Businesses use correlation analysis to understand market trends. This analysis helps identify the relationship between different economic indicators. For example, companies analyze the correlation between consumer spending and economic growth. This understanding allows businesses to make informed decisions. Drive Research often employs this method to predict future market behaviors. Identifying these patterns supports strategic planning and investment.
Consumer Behavior
Understanding consumer behavior is crucial for businesses. Correlation analysis reveals how different factors influence purchasing decisions. Companies examine the correlation between advertising efforts and sales growth. This analysis provides insights into effective marketing strategies. Drive Research uses these findings to tailor campaigns that resonate with target audiences. Businesses can enhance customer engagement by recognizing these relationships.
In Science and Research
Experimental Studies
Researchers rely on correlation analysis in experimental studies. This method uncovers relationships between variables in scientific experiments. Scientists explore the correlation between environmental changes and species adaptation. These insights contribute to advancements in ecological research. Drive Research applies similar techniques to study various scientific phenomena. Understanding these connections aids in developing innovative solutions.
Data Analysis
Data analysis benefits greatly from correlation analysis. Analysts use this method to detect meaningful relationships within datasets. For instance, data scientists investigate the correlation between website traffic and conversion rates. This analysis helps optimize digital marketing strategies. Drive Research leverages these insights to improve client outcomes. Recognizing these correlations enhances decision-making and efficiency.
Common Misconceptions
Correlation vs. Causation
Explanation of the Difference
Many people confuse correlation with causation. Correlation shows a relationship between two variables. Causation indicates that one variable directly affects another. Understanding this difference is crucial for accurate analysis.
Examples of Misinterpretation
Misinterpretations often occur in research. For example, rising breast cancer rates may correlate with increased joint surgeries. This correlation does not mean one causes the other. Orthopedic studies often highlight the need to differentiate between correlational and causal associations.
Limitations of Correlation Analysis
Non-linear Relationships
Correlation analysis focuses on linear relationships. Non-linear relationships may exist between variables. These relationships require different analytical methods. Recognizing this limitation helps in choosing the right approach.
Outliers and Their Impact
Outliers can distort correlation results. These extreme values affect the strength and direction of relationships. Analysts must identify and address outliers. Proper handling ensures more accurate interpretations.
Practical Examples of Correlation Analysis
Case Studies
Real-world Example 1
Blood Pressure and Medication: Researchers often explore the relationship between blood pressure levels and medication effectiveness. A study might measure how a specific drug impacts systolic and diastolic readings. This analysis helps medical professionals tailor treatments to individual needs. Understanding these correlations improves patient outcomes.
Real-world Example 2
Sales and Advertising Spend: Businesses frequently analyze the link between advertising budgets and sales figures. Companies assess how increased spending influences revenue growth. This insight guides marketing strategies and budget allocations. Recognizing these patterns supports more effective decision-making.
Step-by-step Analysis
Data Collection
Start by gathering relevant data. Identify the variables you want to analyze. Use surveys, experiments, or existing datasets. Ensure data accuracy and reliability. Proper data collection forms the foundation of your analysis.
Calculation and Interpretation
Calculate correlation coefficients using statistical software. Choose methods like Pearson or Spearman based on your data type. Analyze the results to understand the strength and direction of relationships. Use visual aids like scatter plots for clarity. Interpret findings to draw meaningful conclusions. Apply insights to inform strategies and decisions.
FAQs and Additional Resources
Frequently Asked Questions
Common Queries
-
What is correlation analysis?
Correlation analysis measures the relationship between two variables. This method helps identify how one variable may influence another. -
How do you interpret correlation coefficients?
A coefficient close to +1 indicates a strong positive relationship. A coefficient close to -1 shows a strong negative relationship. A coefficient near 0 suggests no relationship. -
What is the difference between correlation and causation?
Correlation shows a relationship between variables. Causation means one variable directly affects another.
Expert Answers
-
Can correlation imply causation?
Correlation does not imply causation. Reverse causality can distort relationships. For example, low BMI may correlate with higher mortality due to underlying health issues. -
When should you use Pearson or Spearman correlation?
Use Pearson for linear relationships with normally distributed data. Spearman works well with ordinal data or when outliers are present.
Further Reading and Tools
Recommended Books
-
"Statistics for Business and Economics" by Paul Newbold
This book provides a comprehensive understanding of statistical methods, including correlation analysis. -
"The Art of Statistics: Learning from Data" by David Spiegelhalter
Explore the principles of statistics with practical examples and insights.
Online Resources
-
Khan Academy: Correlation and Causality
Access free courses that explain correlation and its implications. -
Statistical Software Tutorials
Learn how to calculate and interpret correlation coefficients using various software tools.
These resources will enhance your understanding and application of correlation analysis in different fields.
Conclusion
Correlation analysis plays a vital role in understanding relationships between variables. This method empowers you to make informed decisions and uncover hidden patterns. Applying this knowledge in real-world scenarios enhances strategic planning and decision-making. Exploring further resources will deepen your understanding and improve your analytical skills. Embrace correlation analysis as a starting point for investigating relationships and making impactful choices.
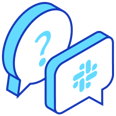
Join StarRocks Community on Slack
Connect on Slack