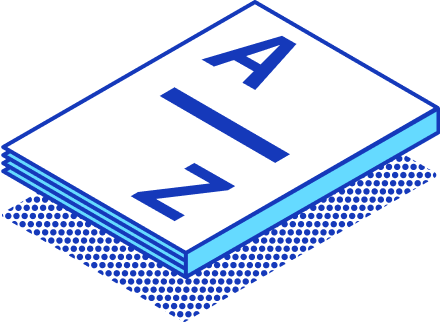
Data Analytics for Financial Services
Understanding Data Analytics for Financial Services
What is Data Analytics?
Data analytics involves examining large datasets to uncover patterns, correlations, and insights. This process transforms raw data into valuable information that supports decision-making. Financial institutions utilize data analytics to enhance their operations and strategies. The ability to analyze both structured and unstructured data provides a competitive edge in the finance sector.
Core Components of Data Analytics
The core components of data analytics include data collection, data cleaning, analysis techniques, and data visualization. Data collection involves gathering relevant information from various sources. Data cleaning ensures accuracy by removing errors and inconsistencies. Analysis techniques apply statistical and computational methods to interpret data. Data visualization presents findings in a clear and understandable format. These components work together to provide comprehensive insights.
Importance in Financial Services
Role in Modern Finance
Data analytics plays a pivotal role in modern finance. Financial institutions rely on data-driven insights to make informed decisions. These insights help in identifying market trends and customer preferences. Data analytics enables organizations to optimize their operations and improve efficiency. The use of advanced analytical tools enhances the ability to forecast financial outcomes and manage risks effectively.
Impact on Business Strategies
Data analytics significantly impacts business strategies in the financial sector. Organizations leverage data to develop innovative products and services. The insights gained from data analytics guide strategic planning and execution. Financial institutions use data to enhance customer experiences and build stronger relationships. The ability to predict market changes allows businesses to adapt quickly and maintain competitiveness.
Historical Evolution and Technological Advancements
Evolution of Data Analytics in Finance
Early Uses and Developments
Data Analytics for Financial Services has undergone significant transformation since the mid-1990s. Financial institutions initially relied on manual calculations and basic statistical tools. These methods provided limited insights and required substantial time and effort. The introduction of data analytics tools marked a turning point. Financial organizations began adopting these tools to streamline operations and enhance decision-making. The shift from manual processes to automated data analysis improved efficiency and accuracy.
Key Milestones
Several key milestones have shaped the evolution of Data Analytics for Financial Services. The development of relational databases allowed for efficient data storage and retrieval. This advancement enabled financial institutions to manage large datasets effectively. The rise of big data technologies further revolutionized data analytics. Financial organizations could now analyze vast amounts of structured and unstructured data. These innovations paved the way for more sophisticated analytical techniques. Financial institutions gained the ability to forecast trends and make informed decisions.
Technological Innovations
Big Data and Machine Learning
Big data and machine learning represent major technological innovations in Data Analytics for Financial Services. Big data platforms provide the infrastructure needed to process and analyze massive datasets. Financial institutions leverage these platforms to gain insights into customer behavior and market trends. Machine learning algorithms enhance predictive analytics capabilities. Financial organizations use these algorithms to identify patterns and make accurate predictions. The combination of big data and machine learning empowers financial institutions to optimize strategies and improve outcomes.
Cloud Computing and AI
Cloud computing and artificial intelligence (AI) have further advanced Data Analytics for Financial Services. Cloud computing offers scalable and cost-effective solutions for data storage and processing. Financial institutions benefit from the flexibility and accessibility of cloud-based analytics platforms. AI technologies, such as natural language processing and computer vision, enhance data analysis capabilities. Financial organizations utilize AI to automate processes and improve decision-making. The integration of cloud computing and AI drives innovation and efficiency in the financial sector.
Benefits of Data Analytics in Financial Services
Enhanced Decision-Making
Data-Driven Strategies
Data Analytics for Financial Services empowers institutions to make informed decisions. Financial organizations analyze structured and unstructured data to uncover patterns. These insights guide strategic planning and execution. Data-driven strategies enable financial institutions to adapt quickly to market changes. The ability to anticipate trends provides a competitive advantage.
Predictive Analytics
Predictive analytics plays a crucial role in forecasting financial outcomes. Financial institutions use advanced algorithms to predict market movements. This capability helps in optimizing investment strategies. Predictive models identify potential risks and opportunities. Financial organizations leverage these insights to enhance decision-making processes.
Risk Management
Identifying and Mitigating Risks
Data Analytics for Financial Services enhances risk management capabilities. Financial institutions utilize data to identify unusual patterns and behaviors. Advanced analytics techniques help in assessing potential threats. Institutions can implement proactive measures to mitigate risks. This approach ensures stability and security in financial operations.
Fraud Detection
Fraud detection becomes more efficient with data analytics. Financial organizations analyze vast datasets to spot fraudulent activities. Machine learning algorithms enhance the accuracy of fraud detection. Institutions can respond swiftly to prevent financial losses. Data analytics strengthens the overall security framework.
Customer Personalization
Tailored Services
Data Analytics for Financial Services enables personalized customer experiences. Financial institutions segment customers based on behavior and preferences. This segmentation allows for tailored product offerings. Personalized services foster customer loyalty and satisfaction. Financial organizations can better meet individual needs through data insights.
Improved Customer Experience
Enhanced customer experience results from effective data analytics. Financial institutions understand customer habits and movements through data analysis. Insights into customer preferences lead to improved service delivery. Organizations build stronger relationships by addressing specific needs. Data analytics transforms customer interactions in the financial sector.
Applications of Data Analytics
Investment and Portfolio Management
Analyzing Market Trends
Data Analytics for Financial Services transforms investment strategies. Financial institutions analyze market trends to make informed decisions. The analysis involves examining historical data and current market conditions. Data analytics tools identify patterns and predict future movements. This process enables financial organizations to anticipate market shifts. Accurate trend analysis enhances investment strategies and maximizes returns.
Optimizing Portfolios
Portfolio optimization benefits from Data Analytics for Financial Services. Financial institutions assess asset performance and risk factors. Data analytics provides insights into asset correlations and diversification opportunities. Institutions use these insights to balance risk and return in portfolios. Optimization techniques improve portfolio performance and align with investment goals. Data-driven decisions enhance the overall efficiency of portfolio management.
Banking and Credit Services
Credit Scoring Models
Credit scoring models rely on Data Analytics for Financial Services. Financial institutions evaluate creditworthiness using data-driven methods. Data analytics assesses factors like payment history and credit utilization. Advanced algorithms calculate credit scores with precision. Accurate credit scoring reduces default risk and improves lending decisions. Financial organizations enhance their credit assessment processes through data analytics.
Loan Approval Processes
Loan approval processes benefit from Data Analytics for Financial Services. Financial institutions streamline applications using data insights. Data analytics evaluates applicant profiles and financial histories. Automated systems expedite loan approvals and reduce processing time. This approach increases efficiency and customer satisfaction. Financial organizations optimize their lending operations with data-driven solutions.
Insurance and Actuarial Science
Risk Assessment
Risk assessment in insurance relies on Data Analytics for Financial Services. Insurers analyze data to evaluate potential risks and liabilities. Data analytics identifies patterns and trends in claims and incidents. This information helps insurers develop accurate risk profiles. Effective risk assessment minimizes losses and enhances policy pricing. Insurers improve their underwriting processes through data-driven insights.
Pricing Strategies
Pricing strategies in insurance benefit from Data Analytics for Financial Services. Insurers analyze customer data to determine premium rates. Data analytics considers factors like age, location, and coverage needs. This approach ensures fair and competitive pricing for policyholders. Insurers adjust pricing strategies based on market trends and customer behavior. Data-driven pricing enhances profitability and customer retention.
Challenges and Considerations
Data Privacy and Security
Regulatory Compliance
Data Analytics for Financial Services requires strict adherence to regulatory compliance. Financial institutions must navigate complex regulations like the Gramm-Leach-Bliley Act of 1999. This act establishes consumers' rights to control how their financial information is shared. Compliance ensures that financial institutions balance privacy with operational needs. Regulatory frameworks guide data handling practices to protect consumer interests.
Protecting Customer Data
Protecting customer data stands as a critical concern in Data Analytics for Financial Services. Financial institutions must implement robust security measures to safeguard sensitive information. Data breaches can lead to severe financial and reputational damage. Encryption and access controls are essential tools for protecting data. Institutions must regularly update security protocols to counter evolving threats.
Integration and Implementation
Overcoming Technical Barriers
Integration of Data Analytics for Financial Services involves overcoming technical barriers. Financial institutions face challenges in merging legacy systems with modern analytics platforms. Compatibility issues can hinder data flow and analysis. Institutions must invest in scalable infrastructure to support data integration. Collaboration between IT and analytics teams ensures seamless implementation.
Ensuring Data Quality
Ensuring data quality is vital for effective Data Analytics for Financial Services. Financial institutions must maintain accurate and consistent datasets. Data cleaning processes remove errors and inconsistencies from raw data. High-quality data enhances the reliability of analytical insights. Institutions must establish data governance frameworks to uphold standards. Regular audits and monitoring help maintain data integrity.
Future of Data Analytics in Financial Services
Emerging Trends
Real-Time Analytics
Real-time analytics transforms how financial institutions operate. Immediate data processing allows organizations to make swift decisions. This capability enhances responsiveness to market changes. Financial services benefit from real-time insights into transactions and customer behavior. Institutions can optimize operations and improve service delivery.
Blockchain and Data Analytics
Blockchain technology revolutionizes data analytics for financial services. Secure and transparent data management becomes possible with blockchain. Financial institutions utilize blockchain to enhance data integrity. This technology supports efficient transaction verification and fraud prevention. Integration of blockchain strengthens analytical capabilities in finance.
Future Technologies
Quantum Computing
Quantum computing promises to elevate data analytics for financial services. Complex calculations become feasible with quantum technology. Financial institutions can analyze vast datasets with unprecedented speed. Quantum computing enhances risk assessment and portfolio optimization. This technology offers a competitive edge in the financial sector.
Advanced AI Models
Advanced AI models drive innovation in data analytics for financial services. Machine learning and natural language processing improve data interpretation. Financial institutions leverage AI to gain deeper insights into financial data. Predictive analytics and automation enhance decision-making processes. AI models support personalized customer experiences and strategic planning.
Conclusion
Data analytics has transformed financial services by enhancing decision-making and operational efficiency. Financial institutions leverage data to gain insights into market trends and customer behavior. Predictive analytics plays a crucial role in forecasting financial outcomes and optimizing strategies. The integration of AI and machine learning continues to drive innovation, providing competitive advantages. As technology evolves, financial organizations will increasingly rely on real-time analytics and advanced models to meet strategic objectives. Data analytics remains essential for navigating the complex landscape of modern finance.
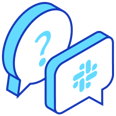
Join StarRocks Community on Slack
Connect on Slack