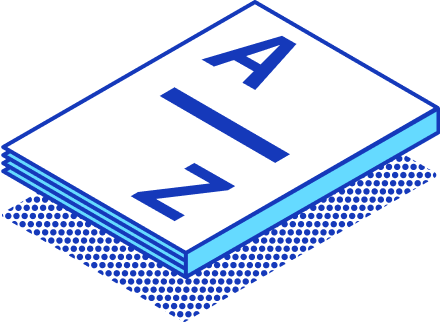
Practical Approaches to Enhance Data Quality
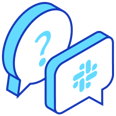
Join StarRocks Community on Slack
Connect on SlackUnderstanding Data Quality
Definition and Key Attributes
Data quality refers to the condition of a data set based on factors such as accuracy, completeness, consistency, reliability, and validity. These attributes determine how well your data serves its intended purpose. When you ensure high data quality, you can trust your data to provide accurate and actionable insights.
-
Accuracy: This attribute measures how closely data reflects the real-world values it represents. Accurate data minimizes errors in decision-making.
-
Completeness: Complete data includes all necessary information. Missing data can lead to incorrect conclusions.
-
Consistency: Consistent data maintains uniformity across different datasets. Inconsistencies can cause confusion and errors.
-
Reliability: Reliable data remains stable over time. It ensures that your analyses are based on dependable information.
-
Validity: Valid data adheres to defined rules and constraints. It ensures that data is logical and meaningful.
By focusing on these key attributes, you can enhance the overall quality of your data. This focus helps you avoid the pitfalls of poor data quality, which can lead to significant financial losses and inefficiencies.
The Significance of Data Quality
Data quality plays a crucial role in your organization's success. Poor data quality can have severe consequences, including financial losses and operational inefficiencies. According to a report by IBM, U.S. businesses lose approximately $3.1 trillion annually due to bad data. This staggering figure highlights the importance of maintaining high data quality standards.
When you prioritize data quality, you enable informed decision-making and optimize operational efficiency. Good data quality allows you to derive accurate, reliable, and actionable insights. These insights drive better decisions, enhance customer experiences, and ensure compliance with regulations.
Addressing data quality issues is pivotal for organizations aiming to harness data-driven insights effectively. Poor data quality can cripple your organization's effectiveness and decision-making processes. By implementing robust data quality management practices, you can transform unreliable data into a valuable asset. This transformation ensures accurate decisions and sustainable growth.
Practical Examples and Use Cases
Data Quality in Healthcare
In healthcare, maintaining high-quality data is crucial for patient care and medical advancements. You rely on accurate and reliable data to make informed decisions about patient treatments and outcomes. Poor data quality can lead to severe consequences, such as misdiagnoses or incorrect treatment plans. For example, Health Net experienced a significant data breach when they lost nine server drives containing unencrypted patient data. This incident affected 2 million individuals and resulted in a $225,000 settlement. Such events highlight the importance of ensuring data quality in healthcare.
To enhance data quality, healthcare organizations must implement robust data governance practices. These practices ensure that data remains accurate, complete, and consistent. By doing so, you can improve patient care and reduce the risk of costly errors. High-quality data also supports medical research and innovation, leading to better healthcare outcomes.
Data Quality in Finance
The finance sector heavily depends on reliable data for decision-making and risk management. Poor data quality can result in financial losses, operational inefficiencies, and compliance issues. U.S. businesses lose approximately $3.1 trillion annually due to bad data, underscoring the need for high-quality data in finance.
You can enhance data quality by implementing data validation techniques and establishing clear guidelines for data entry. These measures help ensure that financial data remains accurate and reliable. By prioritizing data quality, you can make informed investment decisions, manage risks effectively, and maintain regulatory compliance. Reliable data also enables you to provide better customer service and build trust with clients.
Data Quality in Retail
In the retail industry, high-quality data is essential for understanding customer preferences and optimizing inventory management. Poor data quality can lead to revenue loss, inefficiencies, and decreased competitiveness. You need reliable data to make informed decisions about product offerings, pricing strategies, and marketing campaigns.
To improve data quality, retailers should focus on data cleansing and standardizing data quality metrics. These practices help ensure that customer and inventory data remains accurate and consistent. By doing so, you can enhance customer engagement, increase sales, and gain valuable market insights. High-quality data also supports personalized marketing efforts, leading to improved customer satisfaction and loyalty.
Best Practices for Data Quality Assurance
Ensuring high data quality is essential for your organization's success. Implementing effective data quality assurance practices helps you maintain accurate, reliable, and actionable data. Here are some best practices to guide you in this endeavor.
Establishing Data Governance
Data governance forms the backbone of any successful data quality assurance initiative. By establishing a robust data governance framework, you create a structured approach to managing your data assets. This framework defines roles, responsibilities, and processes that ensure data quality across your organization.
-
Define Clear Policies: Set clear policies and standards for data management. These guidelines help you maintain consistency and accuracy in your data.
-
Assign Responsibilities: Designate data stewards who oversee data quality efforts. They ensure adherence to established policies and address any data quality issues.
-
Monitor Compliance: Regularly review and audit data practices to ensure compliance with governance policies. This proactive approach helps you identify and rectify potential problems early.
Implementing Data Profiling
Data profiling is a critical step in your data quality assurance journey. It involves analyzing your data to understand its structure, content, and quality. By implementing data profiling techniques, you can identify anomalies, inconsistencies, and errors in your datasets.
-
Analyze Data Patterns: Examine data patterns to detect irregularities. This analysis helps you pinpoint areas that require attention.
-
Identify Data Anomalies: Use profiling tools to uncover anomalies such as missing values or duplicate records. Addressing these issues enhances data accuracy.
-
Assess Data Quality Metrics: Evaluate key metrics like accuracy, completeness, and consistency. These assessments guide your data quality improvement efforts.
Leveraging Technology
Technology plays a pivotal role in enhancing your data quality assurance efforts. By selecting the right data quality tools, you can automate processes and improve efficiency. These tools help you maintain high data quality standards and streamline your data management practices.
-
Select Data Quality Tools: Choose tools that align with your data quality framework. These tools should support data cleansing, validation, and monitoring.
-
Automate Data Processes: Implement automation to reduce manual errors and increase efficiency. Automation ensures consistent application of data quality rules.
-
Integrate with Existing Systems: Ensure seamless integration of data quality tools with your existing systems. This integration facilitates smooth data flow and enhances overall data quality.
Data quality assurance requires a comprehensive approach that combines governance, profiling, and technology. By following these best practices, you can establish data quality and implement data quality processes effectively. This commitment to data quality assurance not only safeguards your data but also empowers your organization to make informed decisions and drive sustainable growth.
Step-by-Step Guide to Data Quality Assurance
Assessing Current Data Quality
To begin your journey in ensuring data quality, you must first assess the current state of your data. This step involves evaluating the accuracy, completeness, and consistency of your datasets. By understanding the current data quality, you can identify areas that need improvement.
-
Conduct Data Audits: Regularly audit your data to uncover inaccuracies and inconsistencies. This process helps you pinpoint specific issues that may affect your data's reliability.
-
Analyze Data Sources: Examine the origins of your data. Determine if the sources are reliable and if they contribute to accurate data. Reliable sources are crucial for maintaining high data quality.
-
Identify Common Issues: Look for patterns in data errors. Understanding these patterns helps you address root causes effectively. According to data quality engineers, identifying systemic problems is key to successful data quality initiatives.
Developing a Data Quality Plan
Once you have assessed the current data quality, the next step is to develop a comprehensive data quality plan. This plan serves as a roadmap for ensuring data quality across your organization.
-
Set Clear Objectives: Define what you aim to achieve with your data quality initiatives. Clear objectives guide your efforts and help you measure success.
-
Establish Data Quality Metrics: Develop metrics to evaluate the effectiveness of your data quality checks. These metrics provide a benchmark for assessing improvements over time.
-
Create a Data Governance Framework: Implement a framework that outlines roles, responsibilities, and processes for managing data quality. This framework ensures accountability and consistency in your data management practices.
-
Prioritize Data Quality Initiatives: Focus on initiatives that have the most significant impact on your business outcomes. Prioritizing helps you allocate resources effectively and achieve better results.
Implementing and Monitoring
With a solid plan in place, you can now implement and monitor your data quality initiatives. This step involves putting your strategies into action and continuously evaluating their effectiveness.
-
Execute Data Quality Checks: Regularly perform data quality checks to ensure accurate data. These checks help you maintain high standards and prevent errors from affecting your operations.
-
Leverage Technology: Use technology to automate data quality processes. Automation reduces manual errors and increases efficiency in ensuring data quality.
-
Monitor Progress: Continuously monitor the progress of your data quality initiatives. Regular monitoring allows you to make necessary adjustments and improve your strategies.
-
Foster a Culture of Quality: Encourage a culture that values accurate data. Educate your team on the importance of data quality and involve them in your initiatives.
By following this step-by-step guide, you can effectively enhance your data quality assurance efforts. Ensuring data quality not only safeguards your data but also empowers your organization to make informed decisions and drive sustainable growth.
The Role of Data Intelligence Platforms
In today's fast-paced digital world, maintaining high data quality is essential for your organization's success. Data intelligence platforms play a pivotal role in enhancing your data quality efforts. These platforms provide you with the tools and insights needed to manage and improve data quality effectively.
Enhancing Data Quality Efforts
Data intelligence platforms offer a comprehensive suite of features that help you enhance your data quality initiatives. One of the key features is automated data lineage. This feature allows you to track the origins, transformations, and usage of your data. By visualizing data changes at each critical stage, you gain transparency and control over your data quality.
-
Automated Data Lineage: Understand where your data comes from and how it changes over time. This transparency is crucial for maintaining data accuracy and reliability.
-
Centralized Metadata Management: Store and manage metadata in a centralized repository. This enhances data discoverability and governance, ensuring that your data remains consistent and compliant.
-
Data Profiling and Quality Scoring: Use automated tools to profile your data and assign quality scores. This helps you identify and address data quality issues proactively.
By leveraging these features, you can enforce data governance policies and standards. This ensures that your data remains secure and compliant with regulations. Data intelligence platforms empower you to maintain high data quality, which is essential for making informed decisions and driving business success.
Integration with Existing Systems
Integrating a data intelligence platform with your existing systems is crucial for seamless data management. These platforms are designed to work with your current infrastructure, enhancing your data quality efforts without disrupting your operations.
-
Seamless Integration: Ensure that your data intelligence platform integrates smoothly with your existing systems. This facilitates data flow and enhances overall data quality.
-
Automated Data Monitoring: Implement automated data monitoring solutions to continuously analyze your data. This helps you identify and rectify issues in real time, reducing the risk of errors and inconsistencies.
-
Collaboration and Governance: Foster collaboration among your teams by providing a centralized platform for data management. This promotes a culture of data quality and ensures that everyone is aligned with your data governance policies.
By integrating a data intelligence platform into your organization, you can streamline your data management processes and enhance data quality. This integration allows you to focus on strategic initiatives and leverage data as a valuable asset.
Conclusion
Maintaining high data quality is crucial for your organization's success. It impacts decision-making, customer engagement, and operational efficiency. By prioritizing Effective Data Quality Assurance, you ensure that your data remains accurate and reliable. This commitment helps you avoid financial losses and inefficiencies caused by poor data quality.
Key takeaways include the importance of data governance, technology integration, and continuous monitoring. These strategies empower you to derive actionable insights and drive informed decisions. Stay committed to improving data quality. This ongoing effort will enhance your business operations and support sustainable growth.