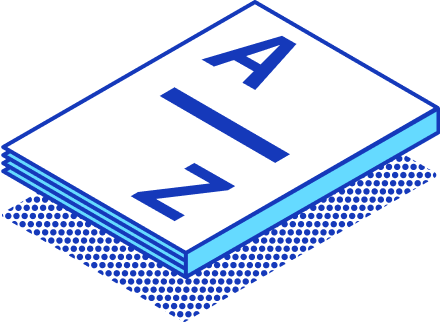
From Theory to Best Practices: Single Instruction, Multiple Data (SIMD)
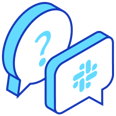
Join StarRocks Community on Slack
Connect on SlackWhat is Single Instruction, Multiple Data (SIMD)?
How SIMD Works
-
Parallel Processing: In traditional CPU operations, instructions are executed on single data elements sequentially. In contrast, SIMD enables the execution of the same operation on multiple data elements at once.
-
Data Organization: Data is organized into vectors or arrays. A SIMD-enabled processor can load, process, and store these vectors efficiently.
-
Instruction Set Architecture (ISA): SIMD utilizes specialized instructions within the processor's instruction set. These instructions are designed to carry out operations on entire data vectors instead of individual elements.
-
Vectorized Operations: Common operations such as addition, subtraction, multiplication, and more are executed on all elements of a vector simultaneously. This parallelism dramatically speeds up processing times for tasks involving large datasets.
-
Reduced Memory Access: Since SIMD processes data in blocks, it reduces the number of times the processor needs to access memory, which is typically a slow operation. This results in a significant performance boost, especially in data-intensive tasks.
-
Efficient Use of Processor Resources: SIMD makes more efficient use of the processor's capabilities, as it can perform multiple operations in the time it would normally take to perform a single operation.
How SIMD Facilitates Vectorization
To fully harness SIMD instruction sets for optimizing vector operations, there are several key steps:
- Step 1: Vectorizing the Code The first step involves converting scalar code into vectorized code, transforming multiple scalar operations into a single vector operation. This can be achieved through automatic compiler vectorization or manually writing vectorized code.
- Step 2: Data Alignment Efficient SIMD operation requires data to be stored in a particular alignment. Aligning data properly ensures that the SIMD instructions can process data in parallel without performance penalties.
- Step 3: Loop Unrolling Loop unrolling expands the number of iterations in a loop, reducing the loop overhead and increasing computational efficiency. This also enhances instruction-level parallelism, further improving performance.
- Step 4: Data Reordering Optimizing data access patterns in memory by reordering data can increase data locality, improving cache utilization and reducing memory access latency. This step is crucial for maximizing SIMD efficiency.
- Step 5: Algorithm Optimization Optimizing the algorithm itself—by reducing unnecessary calculations and memory access—can further improve performance. Techniques like numerical optimization or parallel algorithms help reduce storage and computational overhead.
Key Advantages of a SIMD-Powered Vectorized Query Engine
Increased Throughput and Accelerated Processing
SIMD enables parallel processing of multiple data elements with a single instruction, reducing the total number of instructions and significantly improving execution speed. This is especially beneficial for computationally intensive tasks such as multimedia processing, scientific simulations, and large-scale database queries, where SIMD allows faster processing by handling large data sets simultaneously.
Example: In a database query filtering millions of rows, SIMD processes multiple rows in parallel, cutting down on execution time and boosting throughput compared to scalar processing.
Optimized Cache and Memory Access
By operating on contiguous blocks of memory, SIMD optimizes cache locality, reducing cache misses and memory latency. Processing adjacent data elements in bulk improves memory access efficiency, which is critical for data-heavy tasks.
Example: SIMD-enabled query engines load multiple contiguous data elements into the CPU cache at once, reducing the need for frequent memory lookups and accelerating data processing.
Enhanced Hardware Utilization and Energy Efficiency
SIMD takes full advantage of modern CPUs’ parallel architecture, maximizing core efficiency and reducing idle time. This not only improves performance but also enhances energy efficiency, as fewer processor cycles are needed to complete tasks, leading to lower power consumption.
Example: In modern processors, SIMD instructions enable parallel execution of floating-point operations, improving overall CPU utilization and reducing the energy needed for repetitive calculations.
Simplified Programming with High-Level Abstractions
While SIMD operates at a low hardware level, modern SIMD instruction sets provide high-level programming abstractions that simplify its use. Many compilers and libraries support SIMD out of the box, allowing developers to write optimized code without requiring deep knowledge of processor architecture.
Example: Developers can use built-in functions or libraries in C++ or Python to leverage SIMD, optimizing performance without needing to manually write low-level code.
SIMD-powered vectorized query engines offer significant advantages, including faster processing through parallelism, optimized memory access, better hardware utilization, and improved energy efficiency. Simplified programming abstractions make it easier for developers to take advantage of SIMD’s benefits without extensive low-level optimization efforts. These factors make SIMD essential for high-performance computing tasks across various fields.
Challenges in SIMD Implementation
-
Vectorization: Identifying and vectorizing code sections to leverage SIMD instructions efficiently can be a challenging task, requiring careful analysis and optimization.
-
Data Dependencies: Dependencies among data elements can hinder efficient SIMD execution. Handling dependencies and ensuring data coherence across SIMD lanes can be complex.
-
Memory Alignment: SIMD instructions often require aligned memory access. Ensuring proper memory alignment can be challenging, especially when dealing with irregular data structures or dynamic memory allocations.
-
Code Portability: SIMD implementations are architecture-specific, which can pose challenges when porting SIMD code across different platforms and instruction sets.
-
Load Imbalance: Unequal work distribution among SIMD lanes can result in load imbalance, affecting overall performance. Balancing workloads and data distribution is critical for optimal SIMD utilization.
SIMD Optimization in StarRocks